Tensor Completion Based on Triple Tubal Nuclear Norm.
ALGORITHMS(2018)
摘要
Many tasks in computer vision suffer from missing values in tensor data, i.e., multi-way data array. The recently proposed tensor tubal nuclear norm (TNN) has shown superiority in imputing missing values in 3D visual data, like color images and videos. However, by interpreting in a circulant way, TNN only exploits tube (often carrying temporal/channel information) redundancy in a circulant way while preserving the row and column (often carrying spatial information) relationship. In this paper, a new tensor norm named the triple tubal nuclear norm (TriTNN) is proposed to simultaneously exploit tube, row and column redundancy in a circulant way by using a weighted sum of three TNNs. Thus, more spatial-temporal information can be mined. Further, a TriTNN-based tensor completion model with an ADMM solver is developed. Experiments on color images, videos and LiDAR datasets show the superiority of the proposed TriTNN against state-of-the-art nuclear norm-based tensor norms.
更多查看译文
关键词
tensor completion,tensor SVD,ADMM,image inpainting,video inpainting
AI 理解论文
溯源树
样例
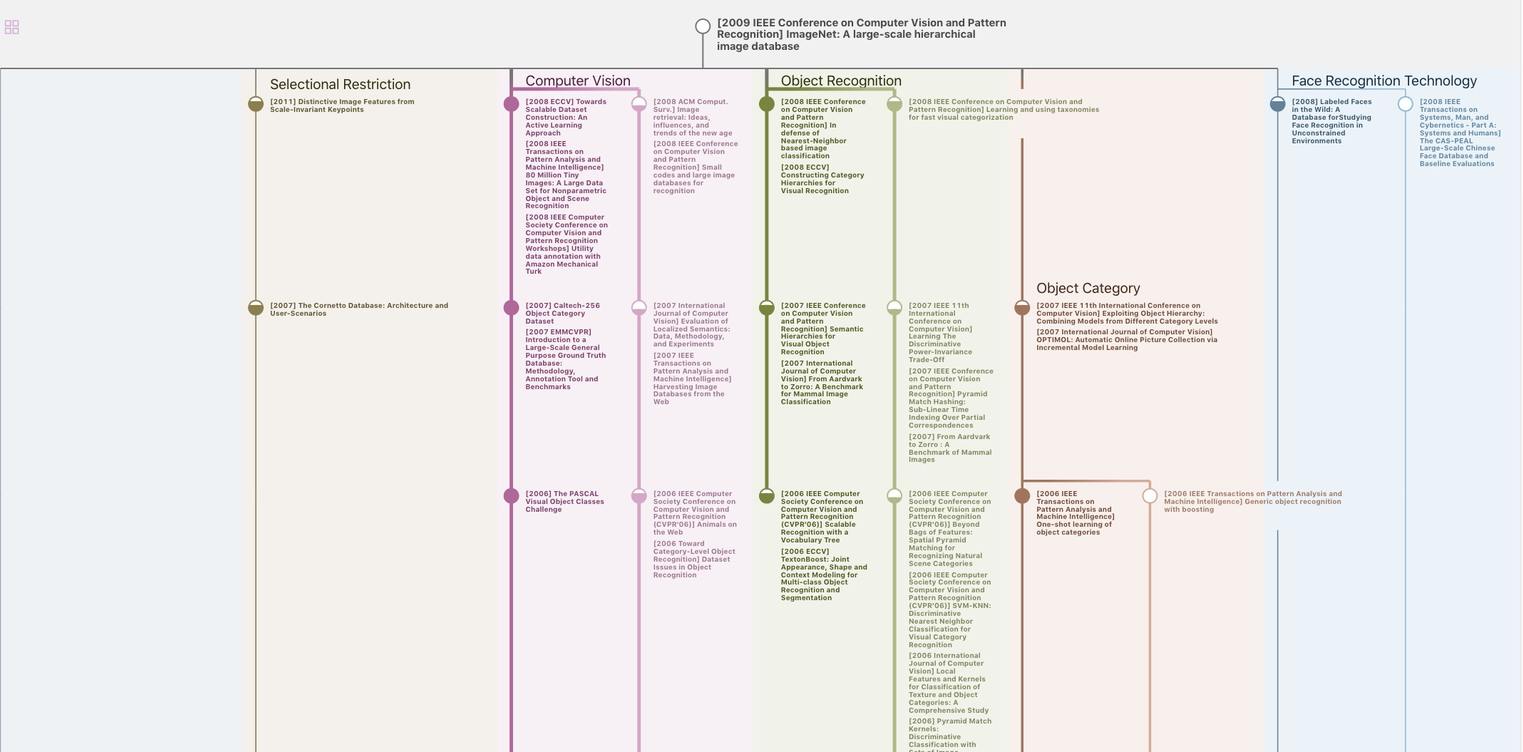
生成溯源树,研究论文发展脉络
Chat Paper
正在生成论文摘要