Learning to embed music and metadata for context-aware music recommendation
World Wide Web(2017)
摘要
Contextual factors greatly influence users’ musical preferences, so they are beneficial remarkably to music recommendation and retrieval tasks. However, it still needs to be studied how to obtain and utilize the contextual information. In this paper, we propose a context-aware music recommendation approach, which can recommend music pieces appropriate for users’ contextual preferences for music. In analogy to matrix factorization methods for collaborative filtering, the proposed approach does not require music pieces to be represented by features ahead, but it can learn the representations from users’ historical listening records. Specifically, the proposed approach first learns music pieces’ embeddings (feature vectors in low-dimension continuous space) from music listening records and corresponding metadata. Then it infers and models users’ global and contextual preferences for music from their listening records with the learned embeddings. Finally, it recommends appropriate music pieces according to the target user’s preferences to satisfy her/his real-time requirements. Experimental evaluations on a real-world dataset show that the proposed approach outperforms baseline methods in terms of precision, recall, F1 score, and hitrate. Especially, our approach has better performance on sparse datasets.
更多查看译文
关键词
Recommender systems, Music recommendation, Context-aware recommendation, Embedding
AI 理解论文
溯源树
样例
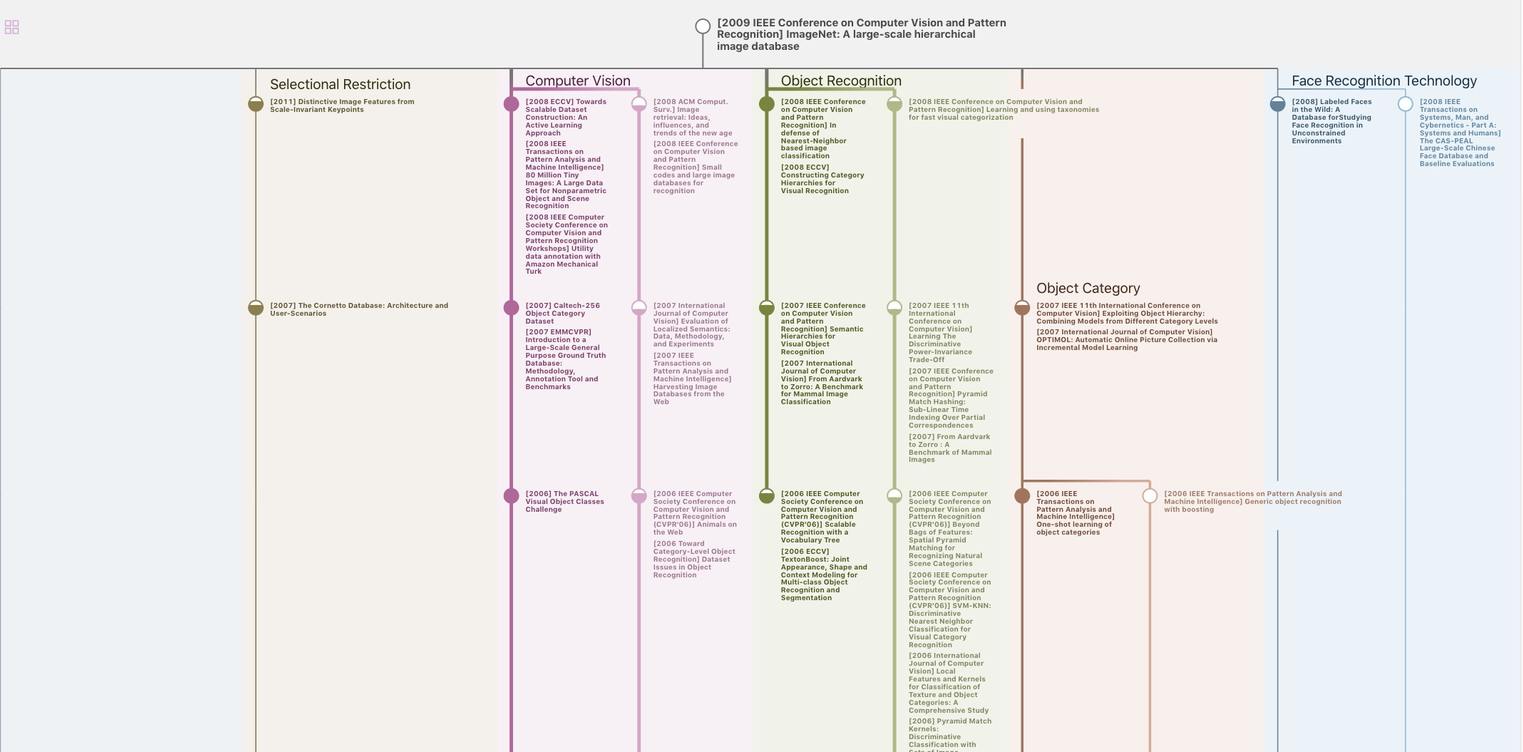
生成溯源树,研究论文发展脉络
Chat Paper
正在生成论文摘要