A Framework for Automated Cellular Network Tuning with Reinforcement Learning.
IEEE Transactions on Communications(2019)
摘要
Tuning cellular network performance against always occurring wireless impairments can dramatically improve reliability to end users. In this paper, we formulate cellular network performance tuning as a reinforcement learning (RL) problem and provide a solution to improve the performance for indoor and outdoor environments. By leveraging the ability of Q-learning to estimate future performance improvement rewards, we propose two algorithms: (1) closed loop power control (PC) for downlink voice over LTE (VoLTE) and (2) self-organizing network (SON) fault management. The VoLTE PC algorithm uses RL to adjust the indoor base station transmit power so that the signal to interference plus noise ratio (SINR) of a user equipment (UE) meets the target SINR. It does so without the UE having to send power control requests. The SON fault management algorithm uses RL to improve the performance of an outdoor base station cluster by resolving faults in the network through configuration management. Both algorithms exploit measurements from the connected users, wireless impairments, and relevant configuration parameters to solve a non-convex performance optimization problem using RL. Simulation results show that our proposed RL based algorithms outperform the industry standards today in realistic cellular communication environments.
更多查看译文
关键词
Cellular networks,Interference,Long Term Evolution,Tuning,Wireless communication,Base stations,Quality of experience
AI 理解论文
溯源树
样例
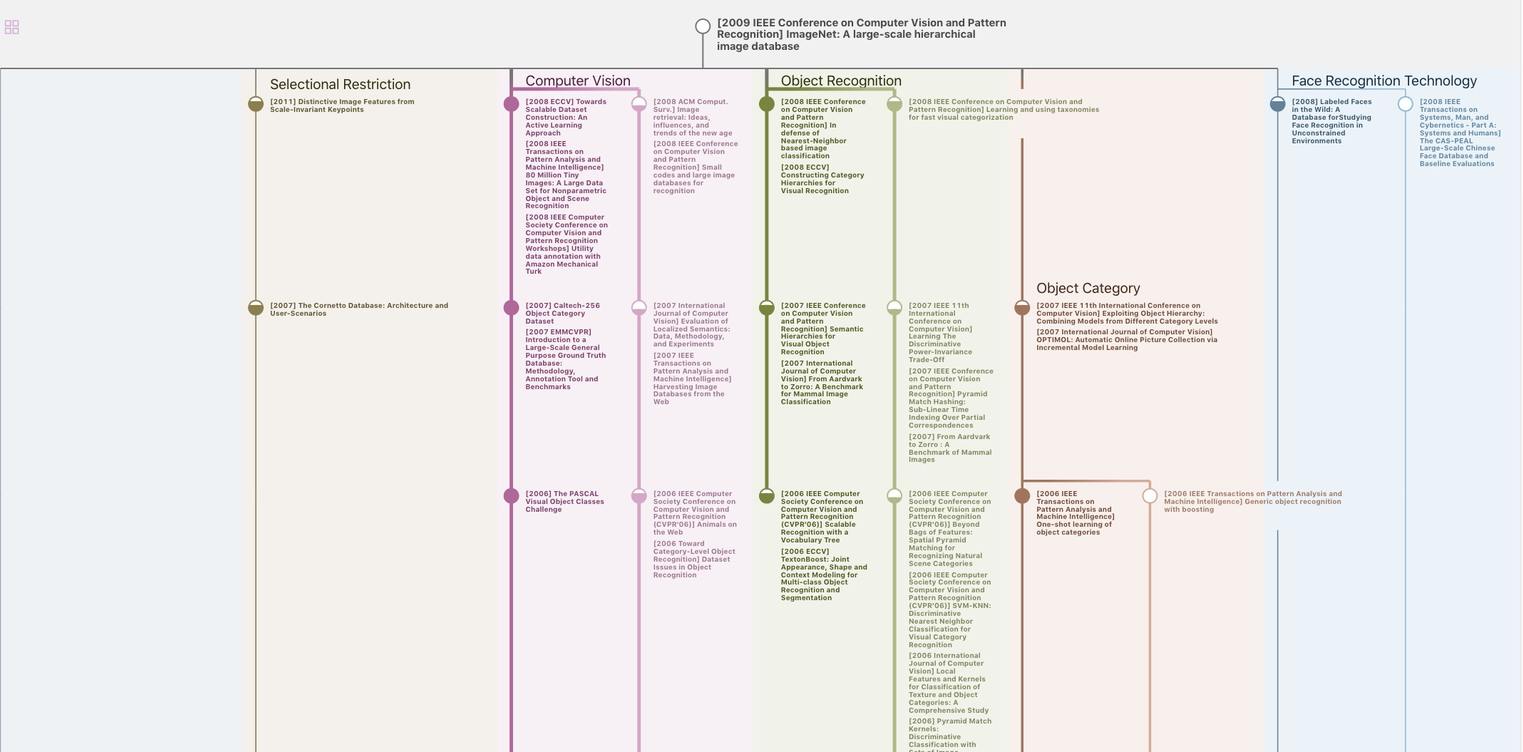
生成溯源树,研究论文发展脉络
Chat Paper
正在生成论文摘要