Reinforced Auto-Zoom Net: Towards Accurate And Fast Breast Cancer Segmentation In Whole-Slide Images
DEEP LEARNING IN MEDICAL IMAGE ANALYSIS AND MULTIMODAL LEARNING FOR CLINICAL DECISION SUPPORT, DLMIA 2018(2018)
摘要
Convolutional neural networks have led to significant breakthroughs in the domain of medical image analysis. However, the task of breast cancer segmentation in whole-slide images (WSIs) is still under-explored. WSIs are large histopathological images with extremely high resolution. Constrained by the hardware and field of view, using high-magnification patches can slow down the inference process and using low-magnification patches can cause the loss of information. In this paper, we aim to achieve two seemingly conflicting goals for breast cancer segmentation: accurate and fast prediction. We propose a simple yet efficient framework Reinforced Auto-Zoom Net (RAZN) to tackle this task. Motivated by the zoom-in operation of a pathologist using a digital microscope, RAZN learns a policy network to decide whether zooming is required in a given region of interest. Because the zoom-in action is selective, RAZN is robust to unbalanced and noisy ground truth labels and can efficiently reduce overfitting. We evaluate our method on a public breast cancer dataset. RAZN outperforms both single-scale and multi-scale baseline approaches, achieving better accuracy at low inference cost.
更多查看译文
关键词
Breast cancer, Deep reinforcement learning, Medical image segmentation, Whole-slide images
AI 理解论文
溯源树
样例
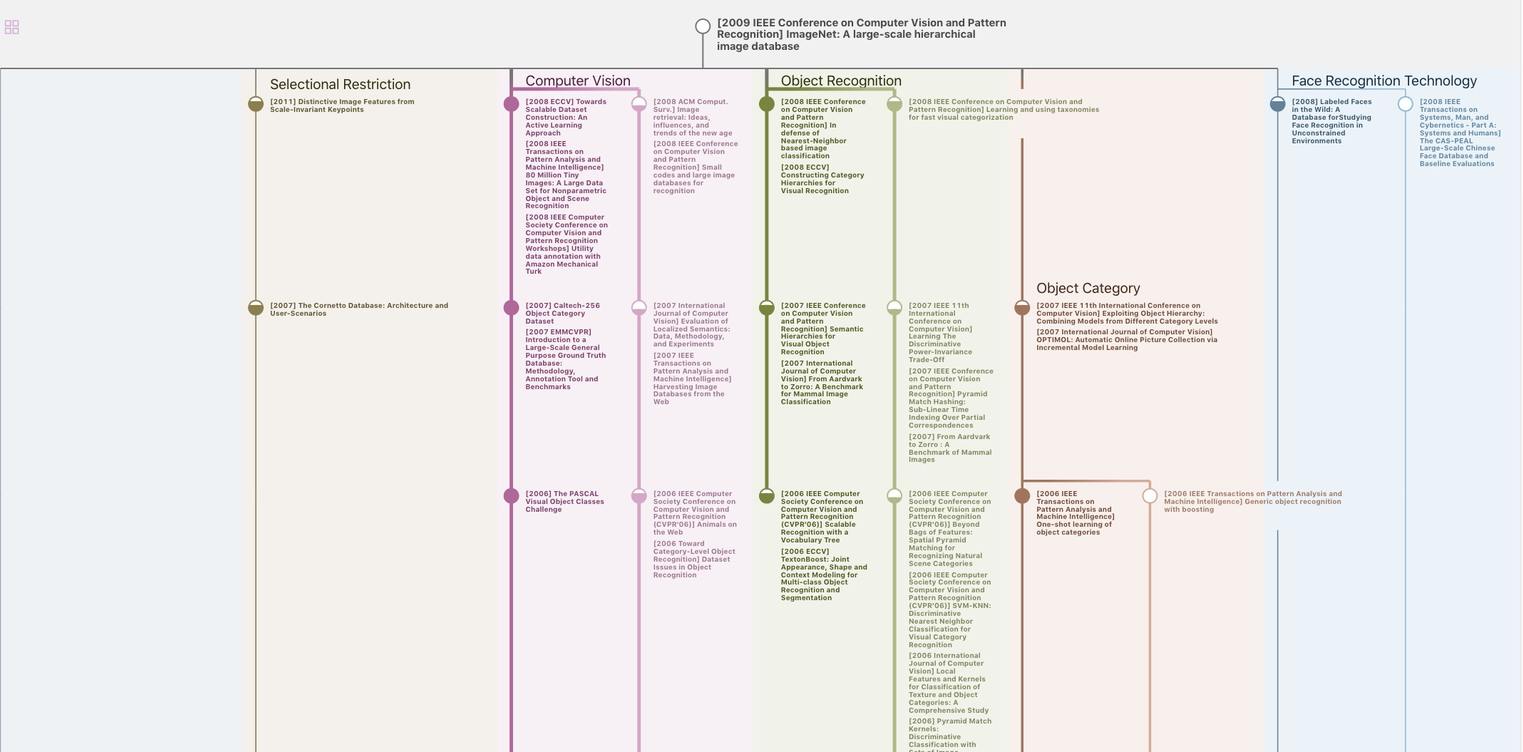
生成溯源树,研究论文发展脉络
Chat Paper
正在生成论文摘要