DeepDownscale: a Deep Learning Strategy for High-Resolution Weather Forecast
2018 IEEE 14th International Conference on e-Science (e-Science)(2018)
摘要
Running high-resolution physical models is computationally expensive and essential for many disciplines. Agriculture, transportation, and energy are sectors that depend on high-resolution weather models, which typically consume many hours of large High Performance Computing (HPC) systems to deliver timely results. Many users cannot afford to run the desired resolution and are forced to use low resolution output. One simple solution is to interpolate results for visualization. It is also possible to combine an ensemble of low resolution models to obtain a better prediction. However, these approaches fail to capture the redundant information and patterns in the low-resolution input that could help improve the quality of prediction. In this paper, we propose and evaluate a strategy based on a deep neural network to learn a high-resolution representation from low-resolution predictions using weather forecast as a practical use case. We take a supervised learning approach, since obtaining labeled data can be done automatically. Our results show significant improvement when compared with standard practices and the strategy is still lightweight enough to run on modest computer systems.
更多查看译文
关键词
Deep Learning,Weather Forecast,Downscale,Machine Learning
AI 理解论文
溯源树
样例
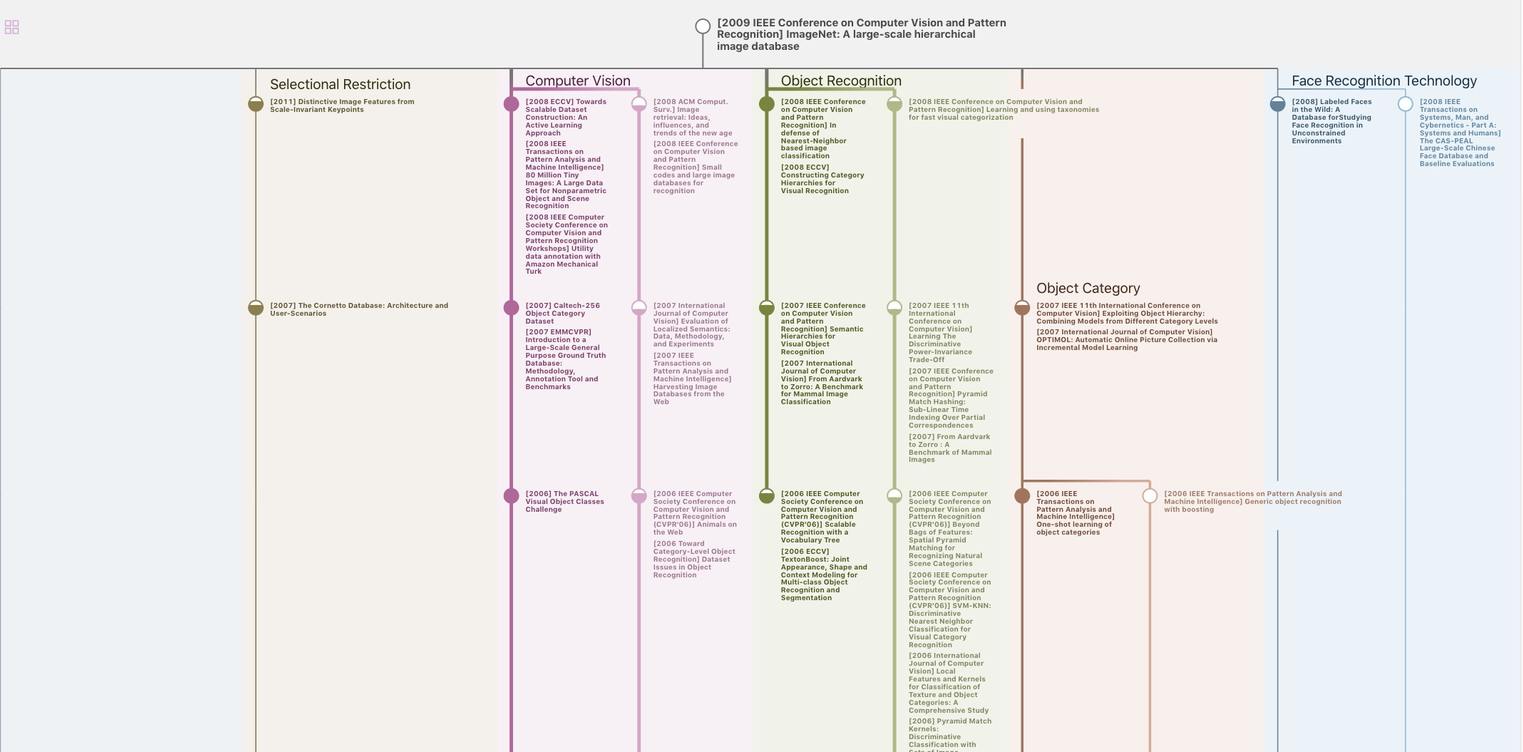
生成溯源树,研究论文发展脉络
Chat Paper
正在生成论文摘要