STEAP: simultaneous trajectory estimation and planning
Autonomous Robots(2018)
摘要
We present a unified probabilistic framework for simultaneous trajectory estimation and planning. Estimation and planning problems are usually considered separately, however, within our framework we show that solving them simultaneously can be more accurate and efficient. The key idea is to compute the full continuous-time trajectory from start to goal at each time-step. While the robot traverses the trajectory, the history portion of the trajectory signifies the solution to the estimation problem, and the future portion of the trajectory signifies a solution to the planning problem. Building on recent probabilistic inference approaches to continuous-time localization and mapping and continuous-time motion planning, we solve the joint problem by iteratively recomputing the maximum a posteriori trajectory conditioned on all available sensor data and cost information. Our approach can contend with high-degree-of-freedom trajectory spaces, uncertainty due to limited sensing capabilities, model inaccuracy, the stochastic effect of executing actions, and can find a solution in real-time. We evaluate our framework empirically in both simulation and on a mobile manipulator.
更多查看译文
关键词
Estimation,Motion planning,Replanning,Trajectory optimization,Probabilistic inference,Factor graphs,Gaussian processes
AI 理解论文
溯源树
样例
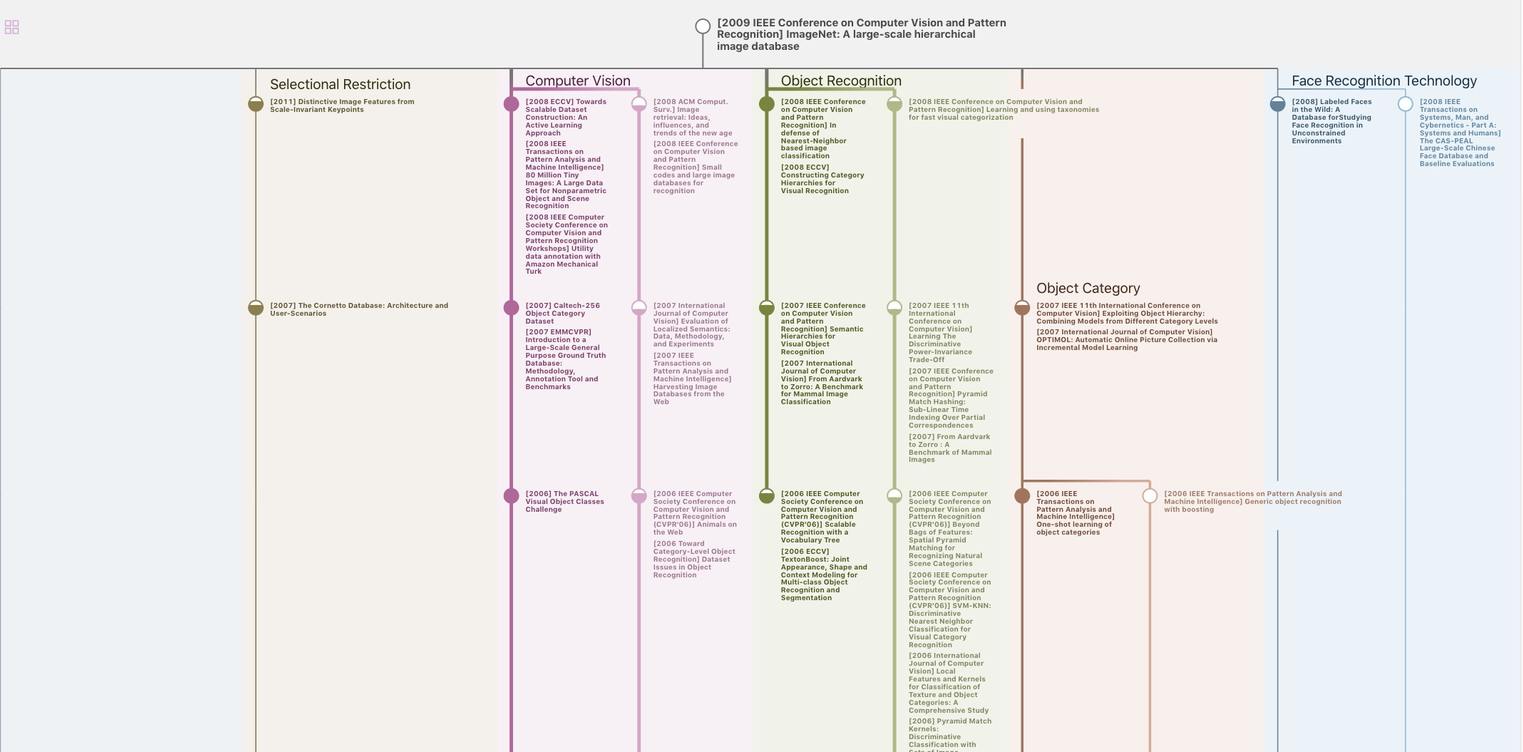
生成溯源树,研究论文发展脉络
Chat Paper
正在生成论文摘要