On the Randomized Complexity of Minimizing a Convex Quadratic Function
arXiv: Learning(2018)
摘要
Minimizing a convex, quadratic objective of the form f_𝐀,𝐛(x) := 1/2x^⊤𝐀 x - ⟨𝐛, x ⟩ for 𝐀≻ 0 is a fundamental problem in machine learning and optimization. In this work, we prove gradient-query complexity lower bounds for minimizing convex quadratic functions which apply to both deterministic and randomized algorithms. Specifically, for κ > 1, we exhibit a distribution over (𝐀,𝐛) with condition number cond(𝐀) ≤κ, such that any randomized algorithm requires Ω(√(κ)) gradient queries to find a solution x̂ for which x̂ - 𝐱_⋆≤ϵ_0𝐱_⋆, where 𝐱_⋆ = 𝐀^-1𝐛 is the optimal solution, and ϵ_0 a small constant. Setting κ =1/ϵ, this lower bound implies the minimax rate of T = Ω(λ_1(𝐀)𝐱_⋆^2/√(ϵ)) queries required to minimize an arbitrary convex quadratic function up to error f(x̂) - f(𝐱_⋆) ≤ϵ. Our lower bound holds for a distribution derived from classical ensembles in random matrix theory, and relies on a careful reduction from adaptively estimating a planted vector 𝐮 in a deformed Wigner model. A key step in deriving sharp lower bounds is demonstrating that the optimization error 𝐱_⋆ - x̂ cannot align too closely with 𝐮. To this end, we prove an upper bound on the cosine between 𝐱_⋆ - x̂ and 𝐮 in terms of the MMSE of estimating the plant 𝐮 in a deformed Wigner model. We then bound the MMSE by carefully modifying a result due to Lelarge and Miolane 2016, which rigorously establishes a general replica-symmetric formula for planted matrix models.
更多查看译文
AI 理解论文
溯源树
样例
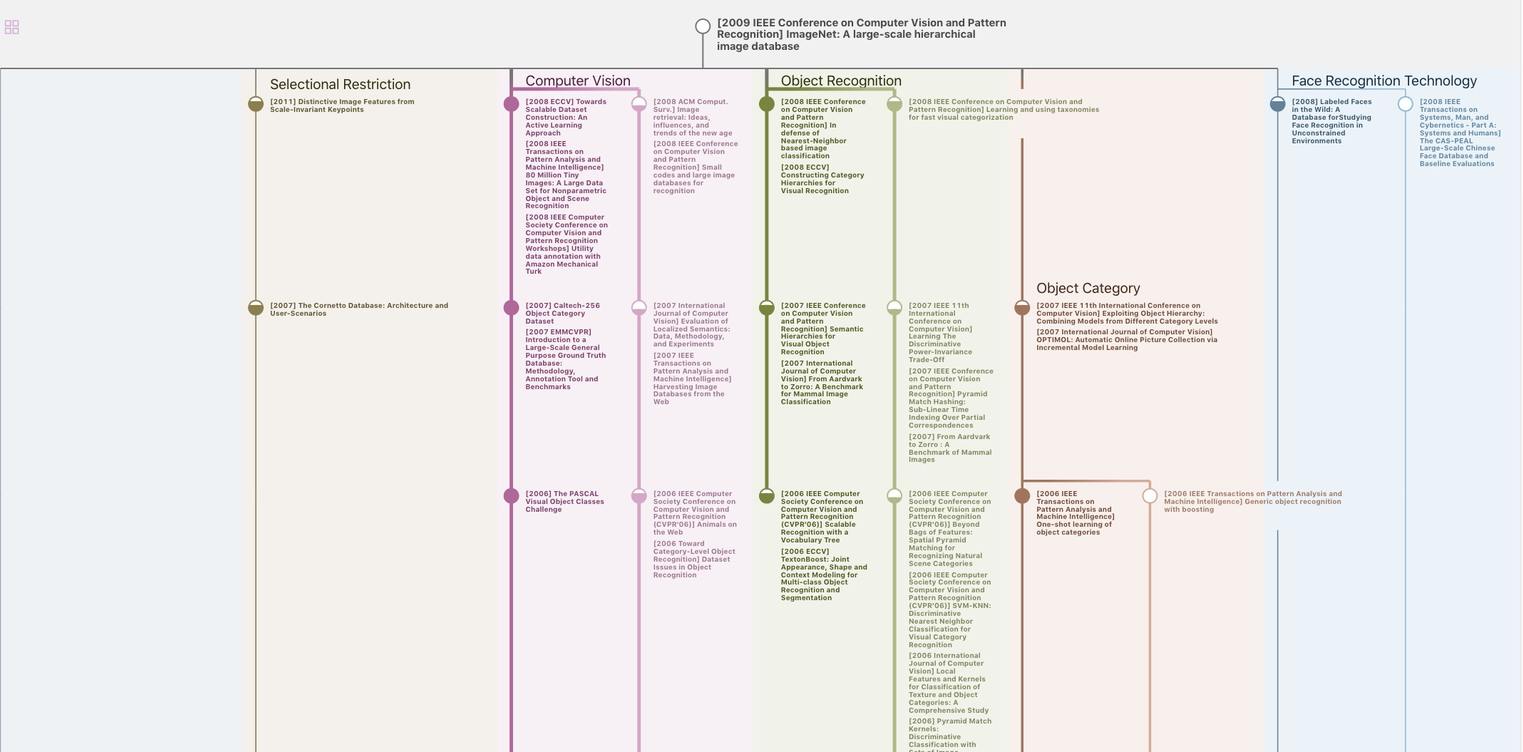
生成溯源树,研究论文发展脉络
Chat Paper
正在生成论文摘要