TLR: Transfer Latent Representation for Unsupervised Domain Adaptation
2018 IEEE INTERNATIONAL CONFERENCE ON MULTIMEDIA AND EXPO (ICME)(2018)
摘要
Domain adaptation refers to the process of learning prediction models in a target domain by making use of data from a source domain. Many classic methods solve the domain adaptation problem by establishing a common latent space, which may cause the loss of many important properties across both domains. In this manuscript, we develop a novel method, transfer latent representation (TLR), to learn a better latent space. Specifically, we design an objective function based on a simple linear autoencoder to derive the latent representations of both domains. The encoder in the autoencoder aims to project the data of both domains into a robust latent space. Besides, the decoder imposes an additional constraint to reconstruct the original data, which can preserve the common properties of both domains and reduce the noise that causes domain shift. Experiments on cross-domain tasks demonstrate the advantages of TLR over competing methods.
更多查看译文
关键词
Domain adaptation,linear autoencoder,object and action recognition
AI 理解论文
溯源树
样例
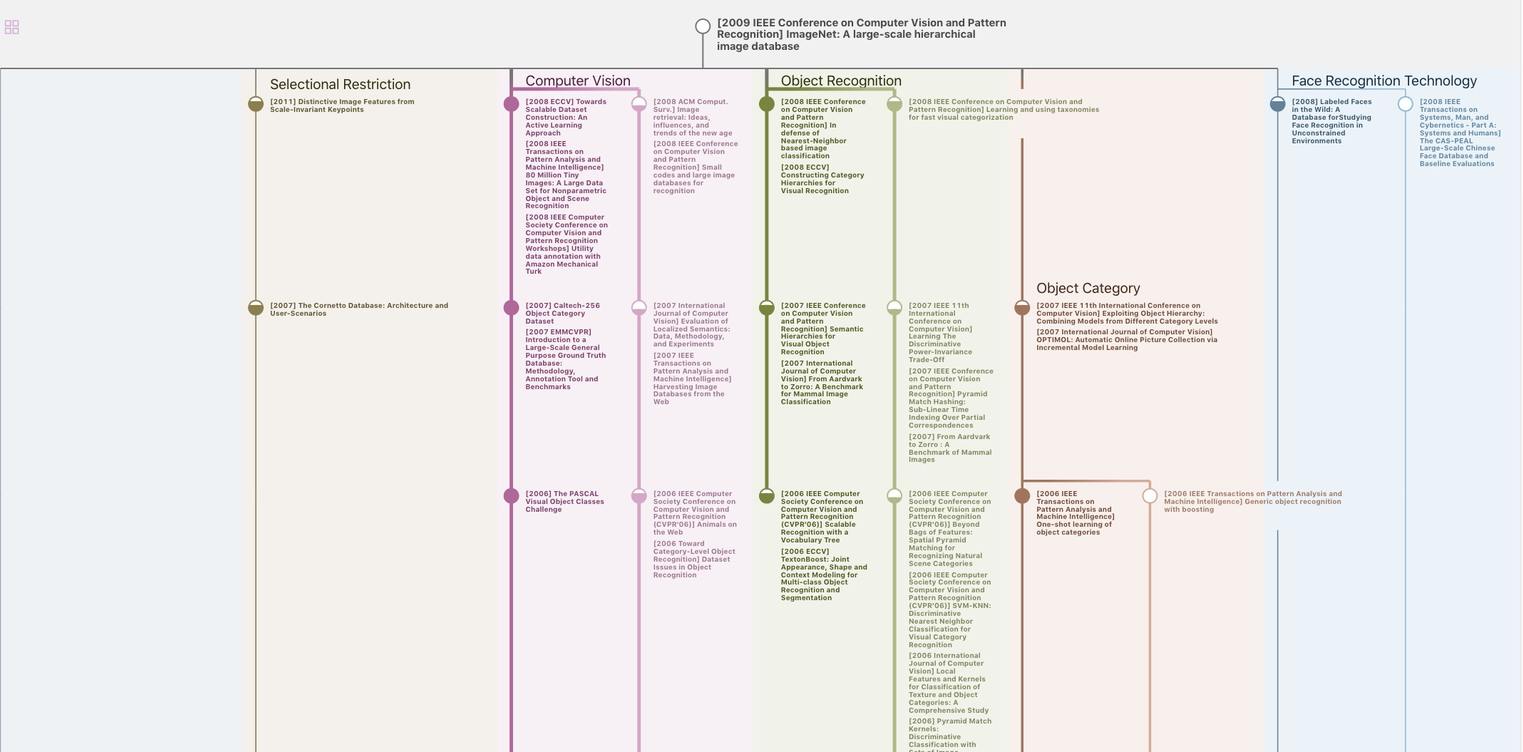
生成溯源树,研究论文发展脉络
Chat Paper
正在生成论文摘要