An Analysis of Asynchronous Stochastic Accelerated Coordinate Descent.
arXiv: Optimization and Control(2018)
摘要
Gradient descent, and coordinate descent in particular, are core tools in machine learning and elsewhere. Large problem instances are common. To help solve them, two orthogonal approaches are known: acceleration and parallelism. In this work, we ask whether they can be used simultaneously. The answer is yes. More specifically, we consider an asynchronous parallel version of the accelerated coordinate descent algorithm proposed and analyzed by Lin, Liu and Xiao (SIOPTu002715). We give an analysis based on the efficient implementation of this algorithm. The only constraint is a standard bounded asynchrony assumption, namely that each update can overlap with at most q others. (q is at most the number of processors times the ratio in the lengths of the longest and shortest updates.) We obtain the following three results: 1. A linear speedup for strongly convex functions so long as q is not too large. 2. A substantial, albeit sublinear, speedup for strongly convex functions for larger q. 3. A substantial, albeit sublinear, speedup for convex functions.
更多查看译文
AI 理解论文
溯源树
样例
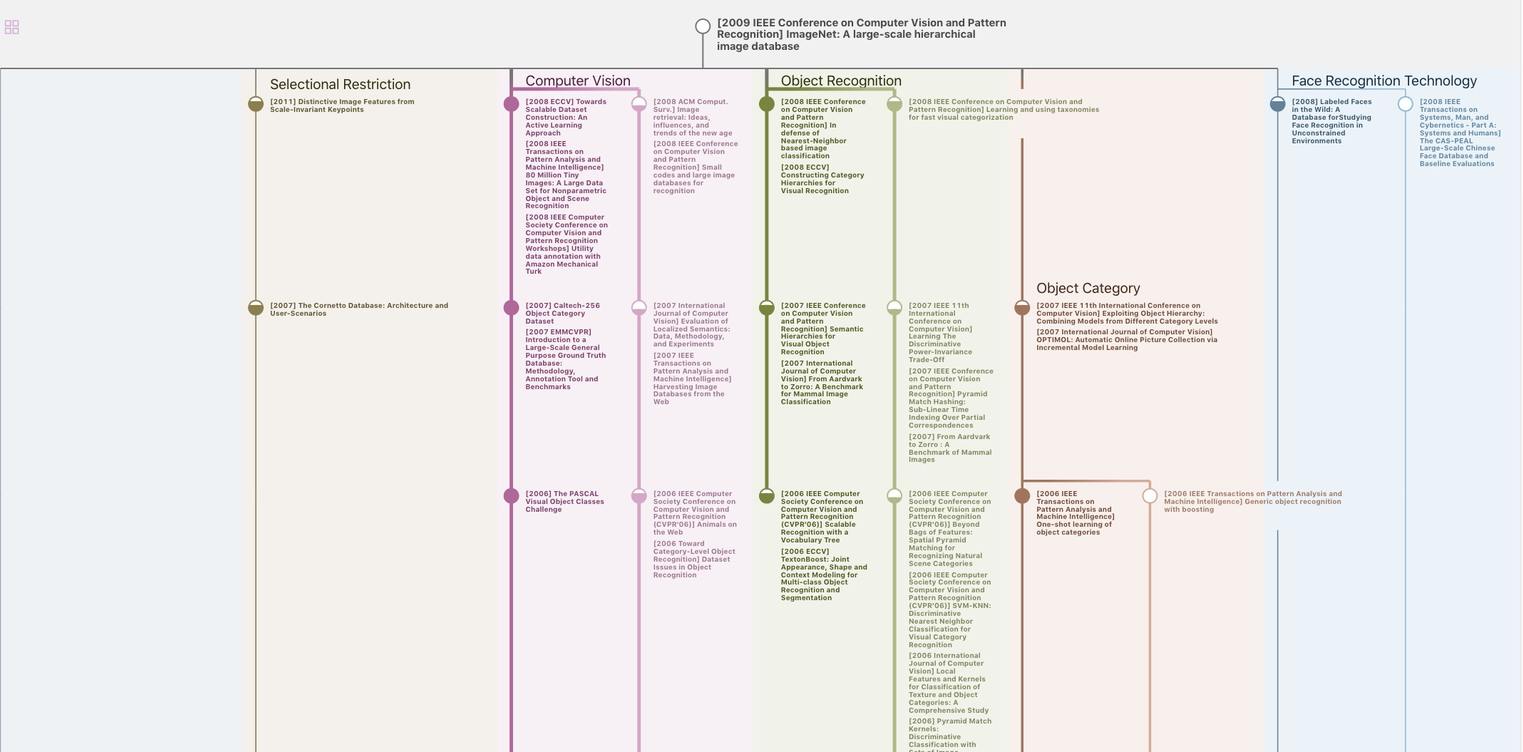
生成溯源树,研究论文发展脉络
Chat Paper
正在生成论文摘要