Semantics Meet Saliency: Exploring Domain Affinity and Models for Dual-Task Prediction
BMVC(2018)
摘要
Much research has examined models for prediction of semantic labels or instances including dense pixel-wise prediction. The problem of predicting salient objects or regions of an image has also been examined in a similar light. With that said, there is an apparent relationship between these two problem domains in that the composition of a scene and associated semantic categories is certain to play into what is deemed salient. In this paper, we explore the relationship between these two problem domains. This is carried out in constructing deep neural networks that perform both predictions together albeit with different configurations for flow of conceptual information related to each distinct problem. This is accompanied by a detailed analysis of object co-occurrences that shed light on dataset bias and semantic precedence specific to individual categories.
更多查看译文
关键词
Interpretable Models,Model Interpretability,Responsibility in AI,Gradient-Based Localization,Visual Explanations
AI 理解论文
溯源树
样例
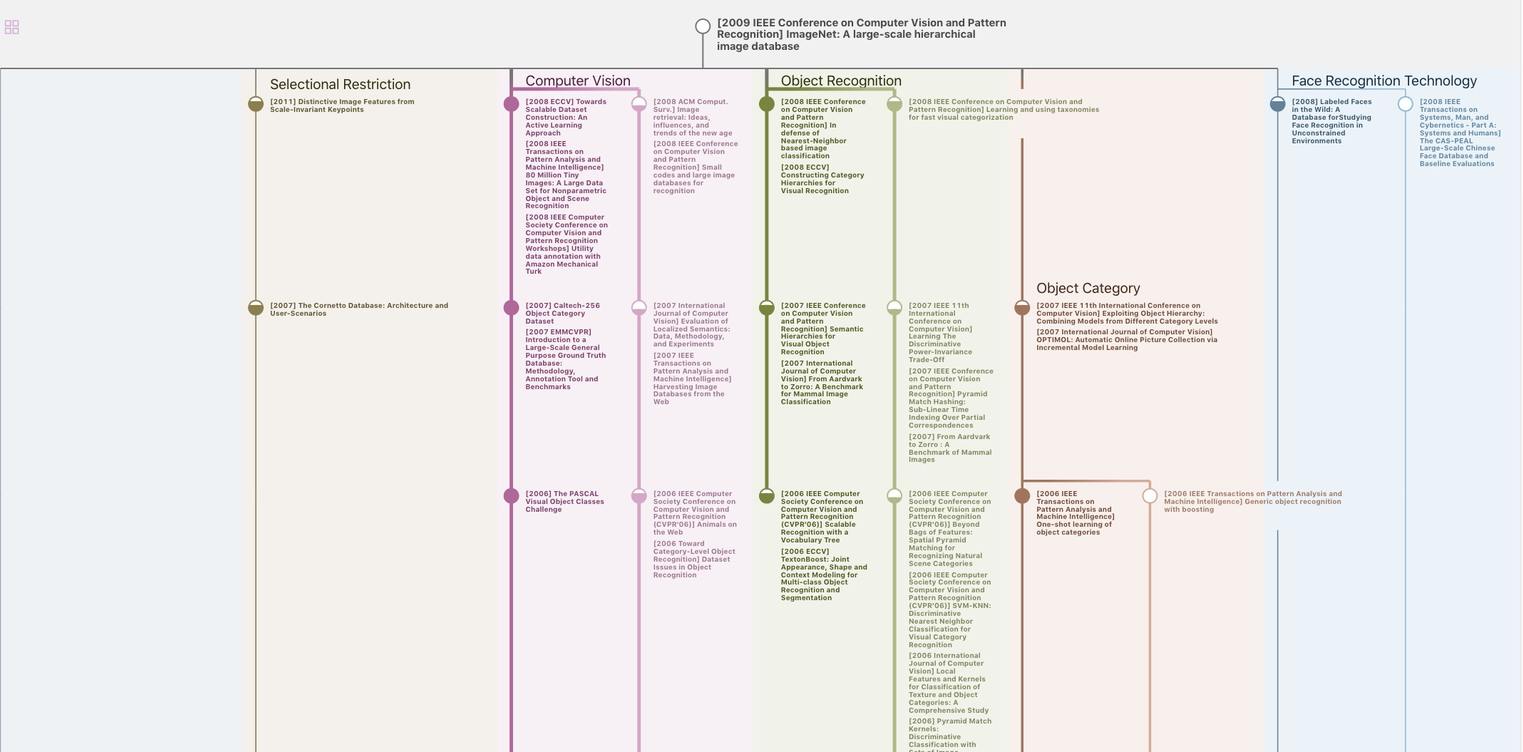
生成溯源树,研究论文发展脉络
Chat Paper
正在生成论文摘要