Adaptive Sampling for Convex Regression.
arXiv: Learning(2018)
摘要
In this paper, we introduce the first principled adaptive-sampling procedure for learning a convex function in the $L_infty$ norm, a problem that arises often in the behavioral and social sciences. We present a function-specific measure of complexity and use it to prove that, for each convex function $f_{star}$, our algorithm nearly attains the information-theoretically optimal, function-specific error rate. We also corroborate our theoretical contributions with numerical experiments, finding that our method substantially outperforms passive, uniform sampling for favorable synthetic and data-derived functions in low-noise settings with large sampling budgets. Our results also suggest an idealized oracle strategy, which we use to gauge the potential advance of any adaptive-sampling strategy over passive sampling, for any given convex function.
更多查看译文
AI 理解论文
溯源树
样例
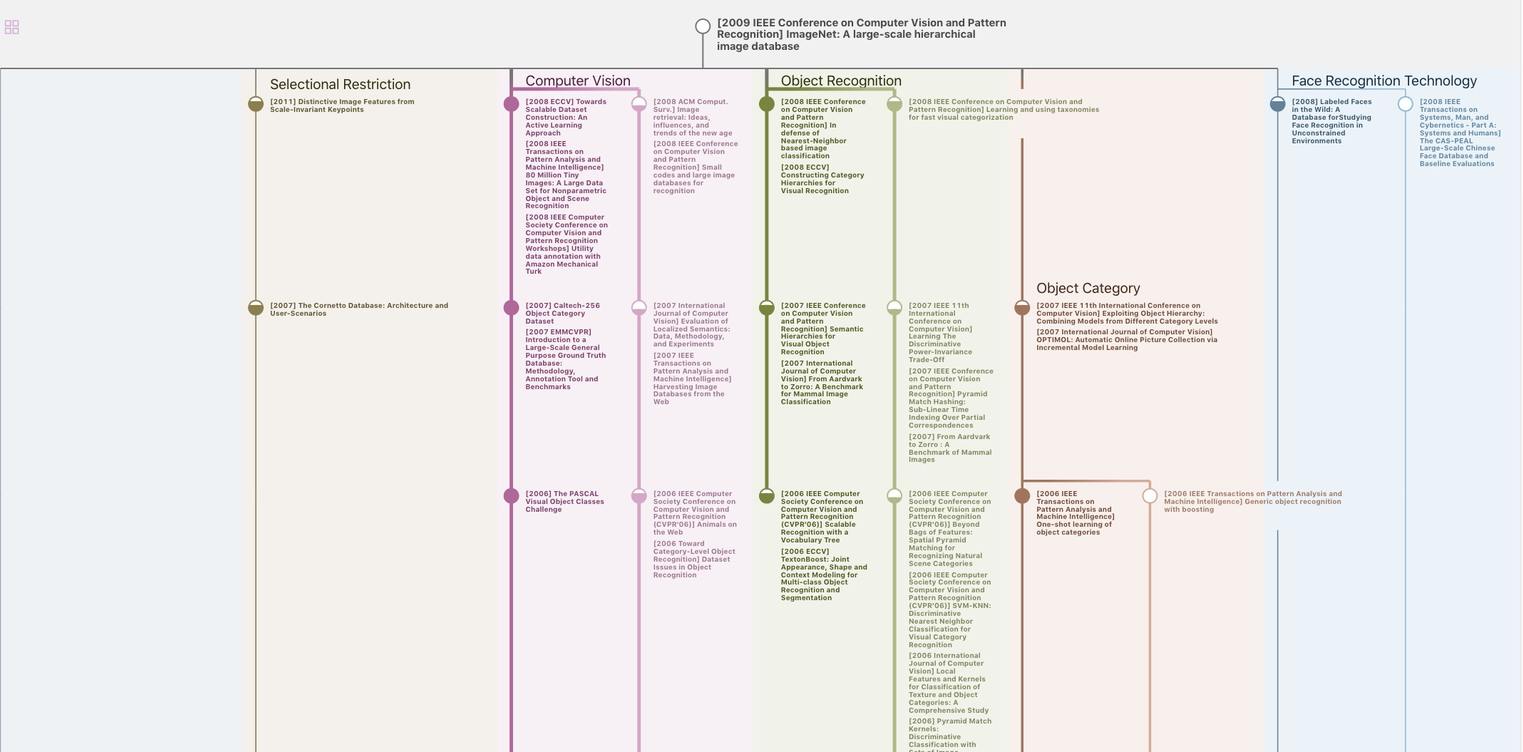
生成溯源树,研究论文发展脉络
Chat Paper
正在生成论文摘要