A Privacy-Preserving Principal Component Analysis Outsourcing Framework
2018 17TH IEEE INTERNATIONAL CONFERENCE ON TRUST, SECURITY AND PRIVACY IN COMPUTING AND COMMUNICATIONS (IEEE TRUSTCOM) / 12TH IEEE INTERNATIONAL CONFERENCE ON BIG DATA SCIENCE AND ENGINEERING (IEEE BIGDATASE)(2018)
摘要
In order to sense and analyze potential information from huge digital data, data mining outsourcing schemes as a principle and effective techniques have recently attracted attention. In this paper, we attempt to guarantee the data privacy of a light-weighted data mining method, Principal Component Analysis (PCA). To achieve our goal, we propose a privacy-preserving PCA(PP-PCA) outsourcing framework, which can understand underlying information without simultaneously disclosing the concrete contents of the data, either the training/predicting data or the prediction results. In our framework, we introduce the Asymmetric Orthogonal Random Linear(AorL) transformation to perturb the training data, utilized by the training model. To protect the privacy of the predicting data and the prediction results, we propose a novel similar-Homomorphic(s-H) preserving technique based on homomorphism encryption mechanism to encrypt the transformed data. Furthermore, our framework is effective and scalable, which allows data users to utilize the trained model from data owner in cloud provider to predict their result. Detailed theoretical analysis and extensive experiments based on three real datasets confirm the security and high efficiency of our framework, respectively.
更多查看译文
关键词
Privacy preserving,PCA,Machine learning,Outsourcing
AI 理解论文
溯源树
样例
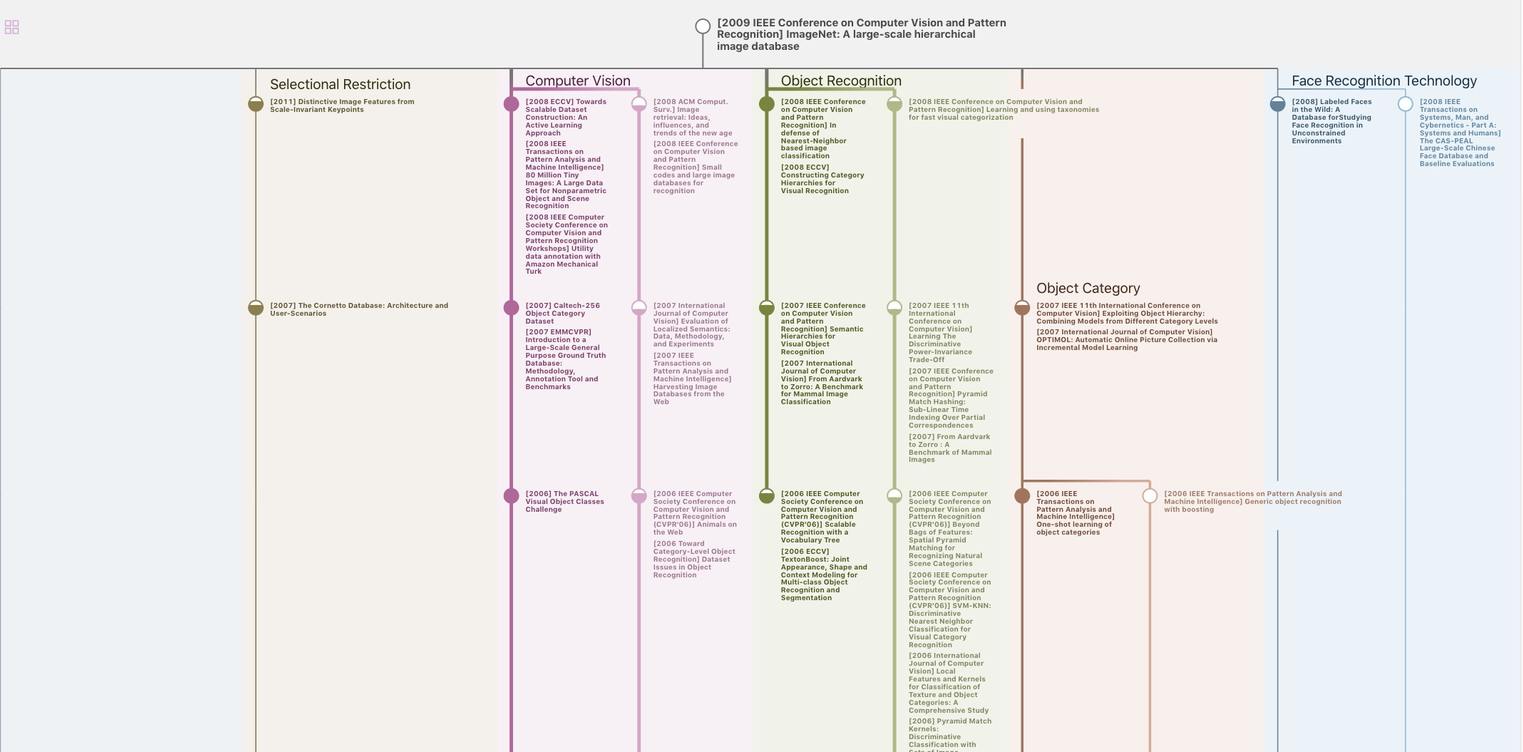
生成溯源树,研究论文发展脉络
Chat Paper
正在生成论文摘要