Face Alignment by Combining Residual Features in Cascaded Hourglass Network
2018 25th IEEE International Conference on Image Processing (ICIP)(2018)
摘要
Fully Convolutional Networks (FCN) are popular in face alignment thanks to its capacity to retain accurate spatial information. In this work we study the effect of kernel functions of FCN for face alignment. We claim that neither the cross entropy nor the pixel-wise L2 losses can reflect the alignment error accurately if we generate the ground truth probability matrix with kernel functions. Based on this analysis, firstly, we develop a Cascaded Hourglass Network (CHN) as our baseline, and then regress the residual face shape via features obtained from the middle layer of the network, which are called Residual Features (RF). The proposed RF-CHN method obtains Normalized Mean Error (NME) of 6.84, which gives an error reduction of 0.21 compared to the current state-of-the-art on the challenging 300-W database.
更多查看译文
关键词
Face Alignment,Fully Convolutional Network,Residual Features
AI 理解论文
溯源树
样例
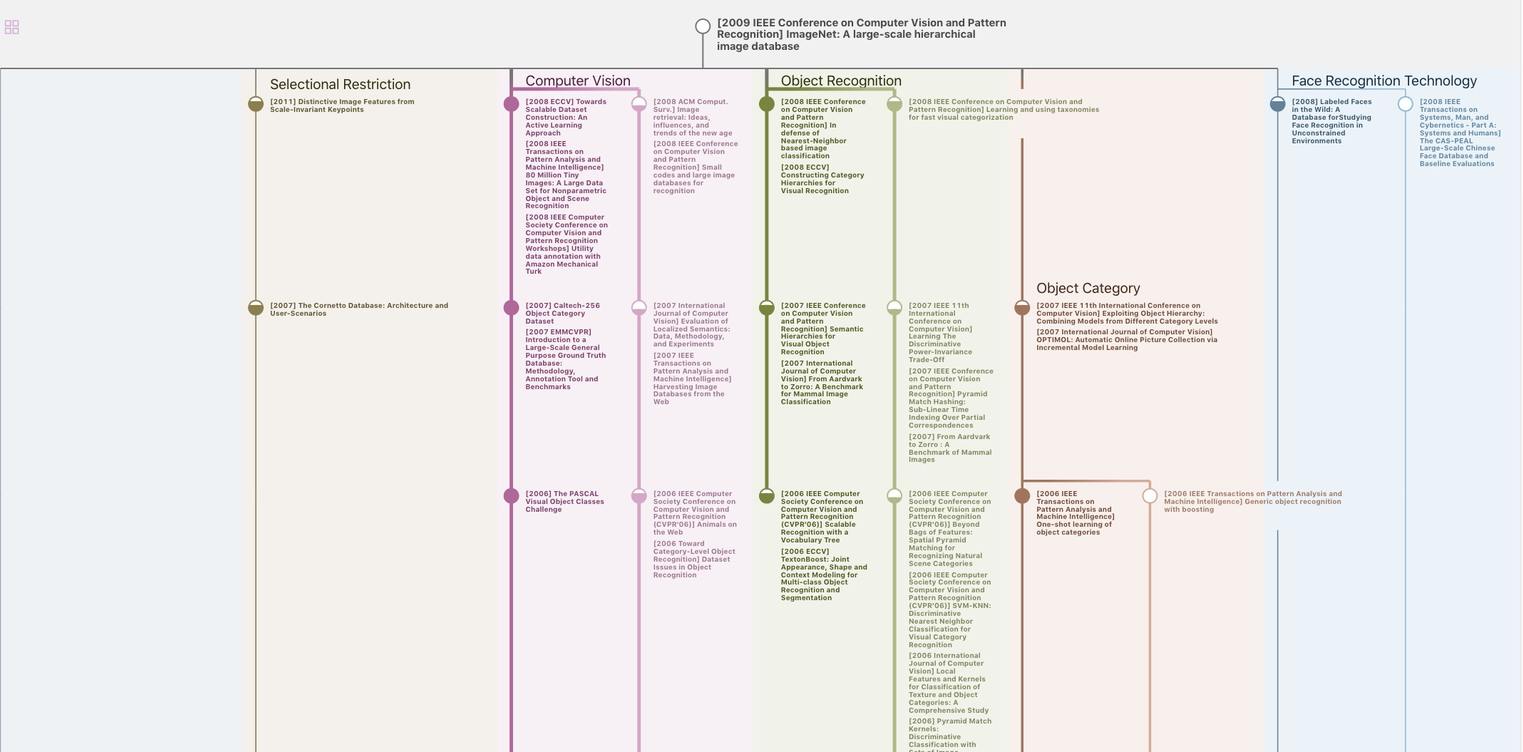
生成溯源树,研究论文发展脉络
Chat Paper
正在生成论文摘要