Recurrent Neural Networks for Classifying Human Embryonic Stem Cell-Derived Cardiomyocytes.
Lecture Notes in Computer Science(2018)
摘要
Classification of human embryonic stem cell-derived cardiomyocytes (hESC-CMs) is important for many applications in cardiac regenerative medicine. However, a key challenge is the lack of ground truth labels for hESC-CMs: Whereas adult phenotypes are well-characterized in terms of their action potentials (APs), the understanding of how the shape of the AP of immature CMs relates to that of adult CMs remains limited. Recently, a new metamorphosis distance has been proposed to determine if a query immature AP is closer to a particular adult AP phenotype. However, the metamorphosis distance is difficult to compute making it unsuitable for classifying a large number of CMs. In this paper we propose a semi-supervised learning framework for the classification of hESC-CM APs. The proposed framework is based on a recurrent neural network with LSTM units whose parameters are learned by minimizing a loss consisting of two parts. The supervised part uses labeled data obtained from computational models of adult CMs, while the unsupervised part uses the metamorphosis distance in an efficient way. Experiments confirm the benefit of integrating information from both adult and stem cell-derived domains in the learning scheme, and also show that the proposed method generates results similar to the state-of-the-art (94.73%) with clear computational advantages when applied to new samples.
更多查看译文
关键词
Semi-supervised learning,LSTM,Embryonic cardiomyocytes
AI 理解论文
溯源树
样例
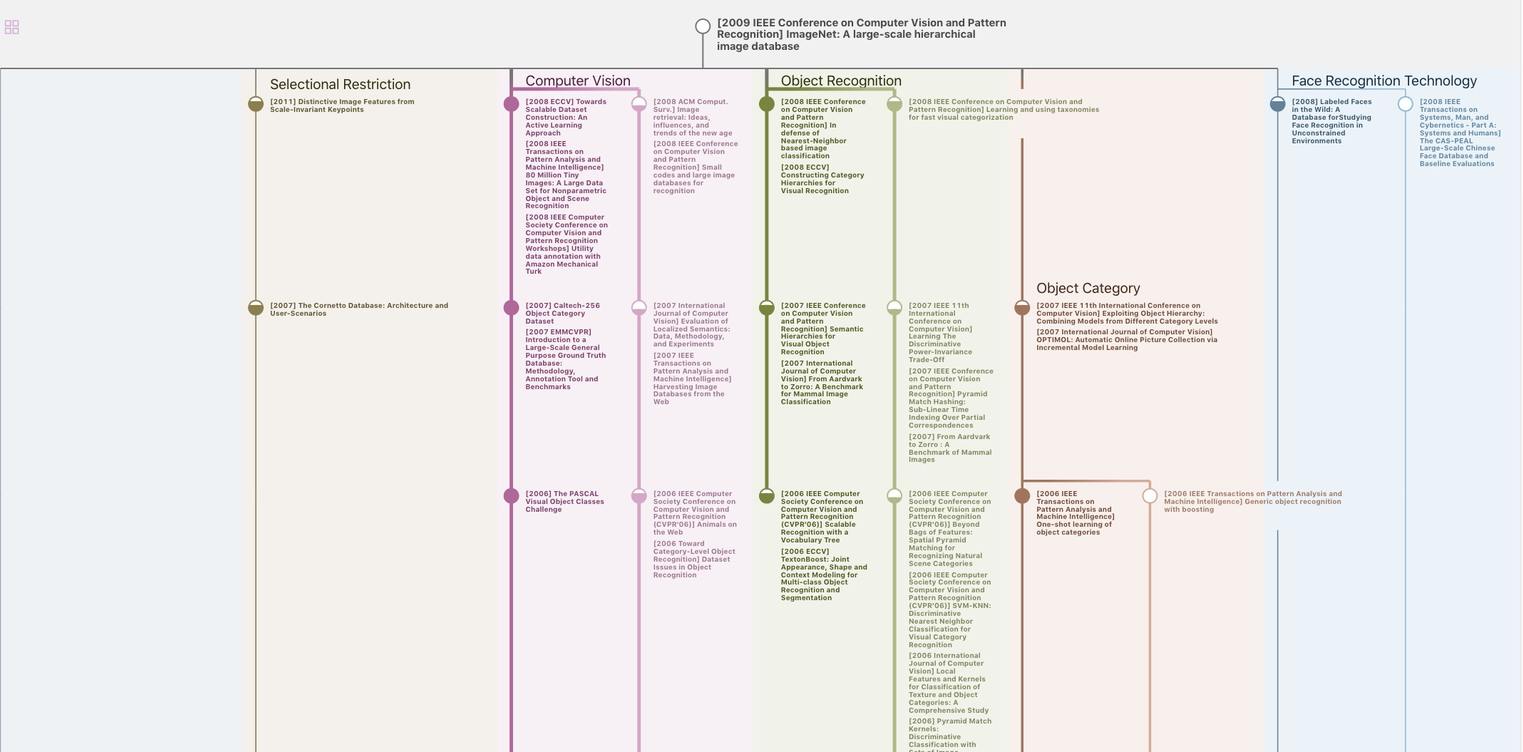
生成溯源树,研究论文发展脉络
Chat Paper
正在生成论文摘要