Ordinal Multi-modal Feature Selection for Survival Analysis of Early-Stage Renal Cancer.
Lecture Notes in Computer Science(2018)
摘要
Existing studies have demonstrated that combining genomic data and histopathological images can better stratify cancer patients with distinct prognosis than using single biomarker, for different biomarkers may provide complementary information. However, these multi-modal data, most high-dimensional, may contain redundant features that will deteriorate the performance of the prognosis model, and therefore it has become a challenging problem to select the informative features for survival analysis from the redundant and heterogeneous feature groups. Existing feature selection methods assume that the survival information of one patient is independent to another, and thus miss the ordinal relationship among the survival time of different patients. To solve this issue, we make use of the important ordinal survival information among different patients and propose an ordinal sparse canonical correlation analysis (i.e., OSCCA) framework to simultaneously identify important image features and eigengenes for survival analysis. Specifically, we formulate our framework basing on sparse canonical correlation analysis model, which aims at finding the best linear projections so that the highest correlation between the selected image features and eigengenes can be achieved. In addition, we also add constrains to ensure that the ordinal survival information of different patients is preserved after projection. We evaluate the effectiveness of our method on an early-stage renal cell carcinoma dataset. Experimental results demonstrate that the selected features correlated strongly with survival, by which we can achieve better patient stratification than the comparing methods.
更多查看译文
关键词
Sparse Canonical Correlation Analysis, Eigengene, Multi-modal Data, Selected Image Features, Early Stage Renal Cell Carcinoma
AI 理解论文
溯源树
样例
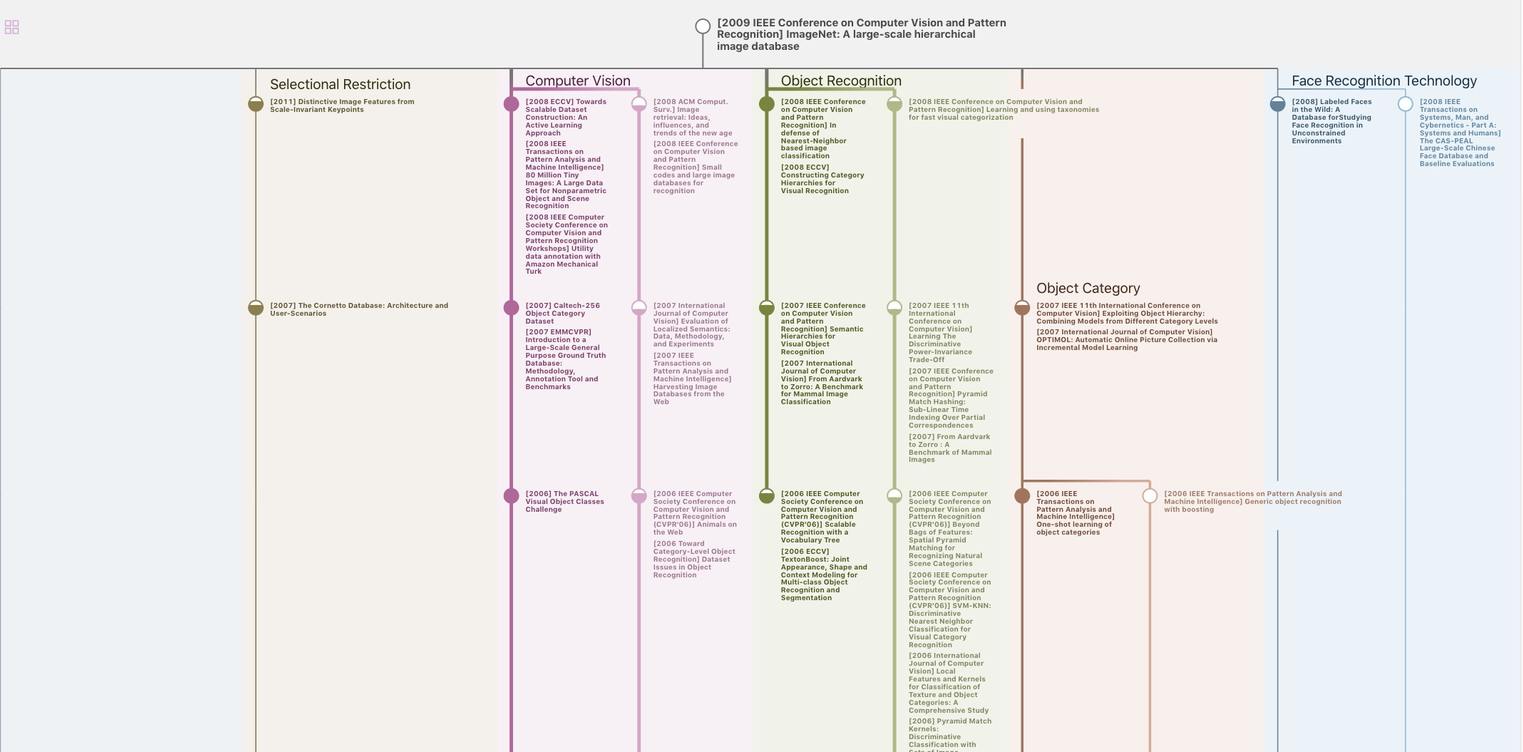
生成溯源树,研究论文发展脉络
Chat Paper
正在生成论文摘要