Handling Missing Annotations for Semantic Segmentation with Deep ConvNets.
Lecture notes in computer science(2018)
摘要
Annotation of medical images for semantic segmentation is a very time consuming and difficult task. Moreover, clinical experts often focus on specific anatomical structures and thus, produce partially annotated images. In this paper, we introduce SMILE, a new deep convolutional neural network which addresses the issue of learning with incomplete ground truth. SMILE aims to identify ambiguous labels in order to ignore them during training, and don’t propagate incorrect or noisy information. A second contribution is SMILEr which uses SMILE as initialization for automatically relabeling missing annotations, using a curriculum strategy. Experiments on 3 organ classes (liver, stomach, pancreas) show the relevance of the proposed approach for semantic segmentation: with 70% of missing annotations, SMILEr performs similarly as a baseline trained with complete ground truth annotations.
更多查看译文
关键词
Medical images,Deep learning,Convolutional Neural Networks,Incomplete ground truth annotation,Noisy labels,Missing labels
AI 理解论文
溯源树
样例
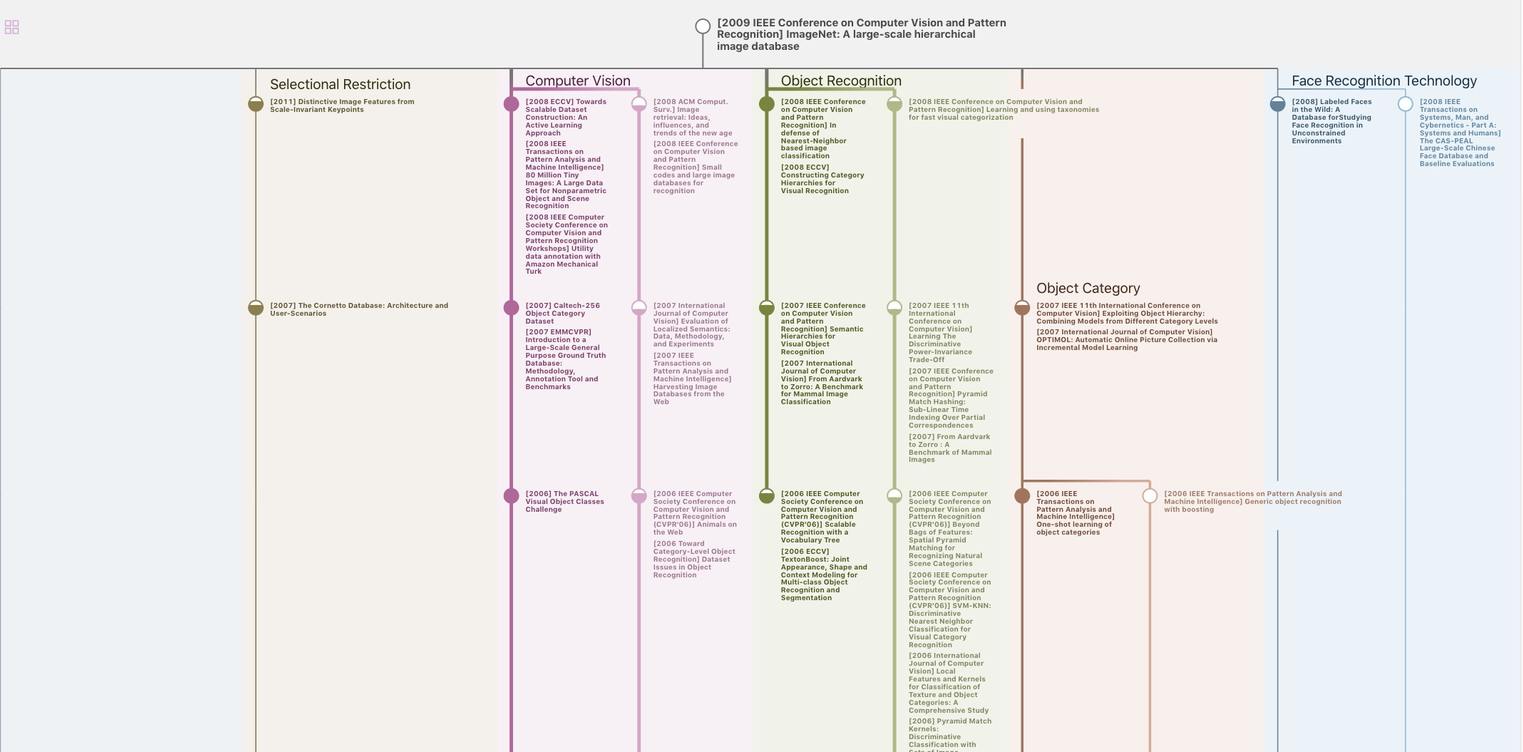
生成溯源树,研究论文发展脉络
Chat Paper
正在生成论文摘要