Modeling Longitudinal Voxelwise Feature Change in Normal Aging with Spatial-Anatomical Regularization.
Lecture Notes in Computer Science(2018)
摘要
Image voxel/vertex-wise feature in the brain is widely used for automatic classification or significant region detection of various dementia syndromes. In these studies, the non-imaging variables, such as age, will affect the results, but may be uninterested to the clinical applications. Imaging data can be considered as a combination of the confound variable (e. g. age) and the variable of clinical interest (e. g. AD diagnosis). However, non-imaging confound variable is not well dealt in each voxel. In this paper, we proposed a spatial-anatomical regularized parametric function fitting approach that explicitly modeling the relationship between the voxelwise feature and the confound variable. By adding the spatial-anatomical regularization, our model not only obtains a better voxelwise feature estimation, but also generates a more interpretable parameter map to help understand the effect of confound variable on imaging features. Besides the commonly used linear model, we also develop a spatial-anatomical regularized voxelwise general logistic model to investigate deeper of the aging process in gray matter and white matter density map.
更多查看译文
关键词
Voxelwise feature,Spatial-anatomical regularization,General logistic,Longitudinal model,Normal aging
AI 理解论文
溯源树
样例
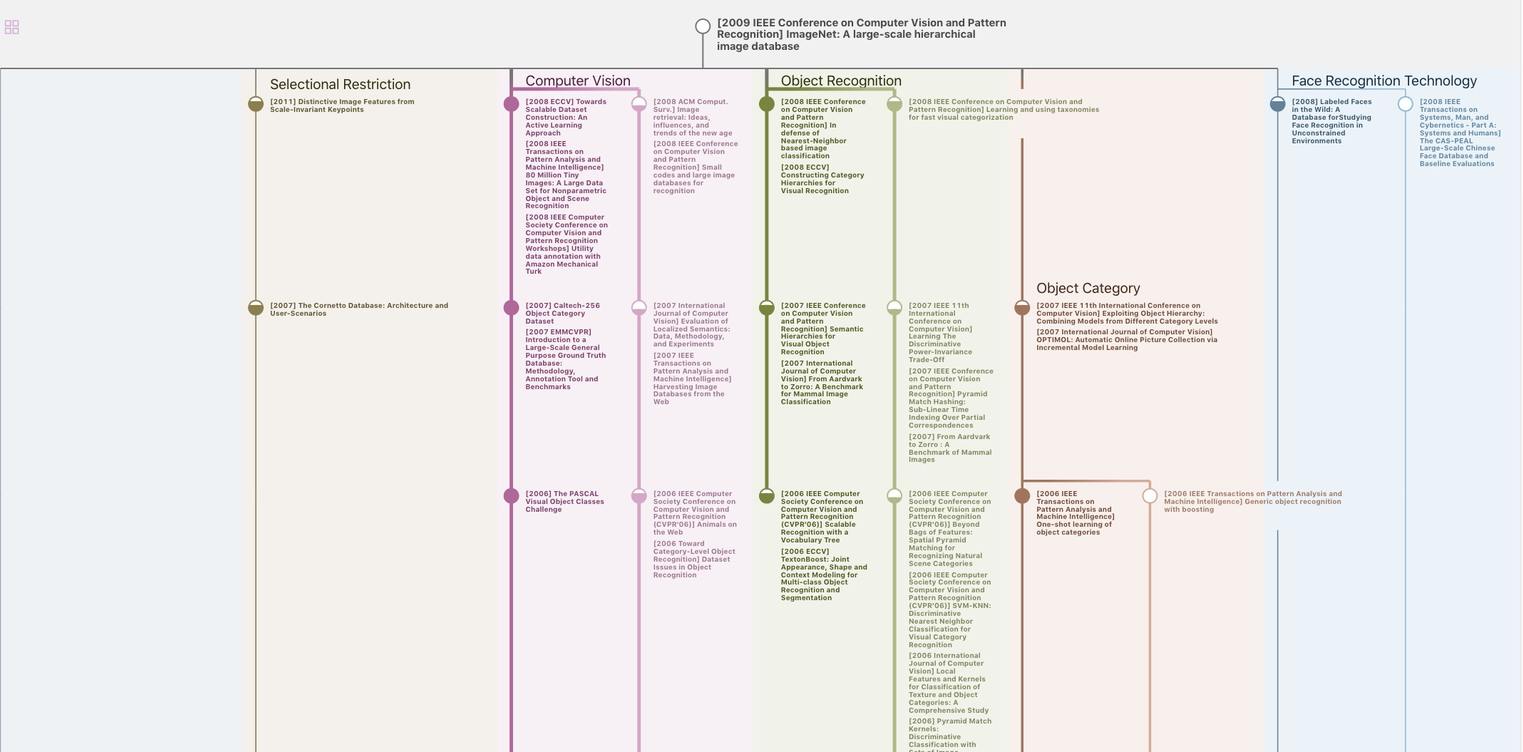
生成溯源树,研究论文发展脉络
Chat Paper
正在生成论文摘要