Iterated Lifting for Robust Cost Optimization.
BMVC(2017)
摘要
Optimization of latent model parameters using robust formulations usually creates a large number of local minima due to the quasi-convex shape of the underlying robust kernel. Lifting the robust kernel, i.e. embedding the problem into a higher-dimensional space, leads to significantly better local minima in a range of 3D computer vision problems (e.g. [10, 11, 12]). In this work we propose to iterate this lifting construction to obtain a more gradual lifting scheme for a given target kernel. Thus, a robust kernel is not directly lifted against the (non-robust) quadratic kernel, but initially against a different , less robust kernel. This process is iterated until the quadratic kernel is reached to allow utilization of efficient non-linear least-squares solvers. We demonstrate in synthetic and real problem instances that iterated lifting generally reaches better local minima than IRLS and standard half-quadratic lifting.
更多查看译文
AI 理解论文
溯源树
样例
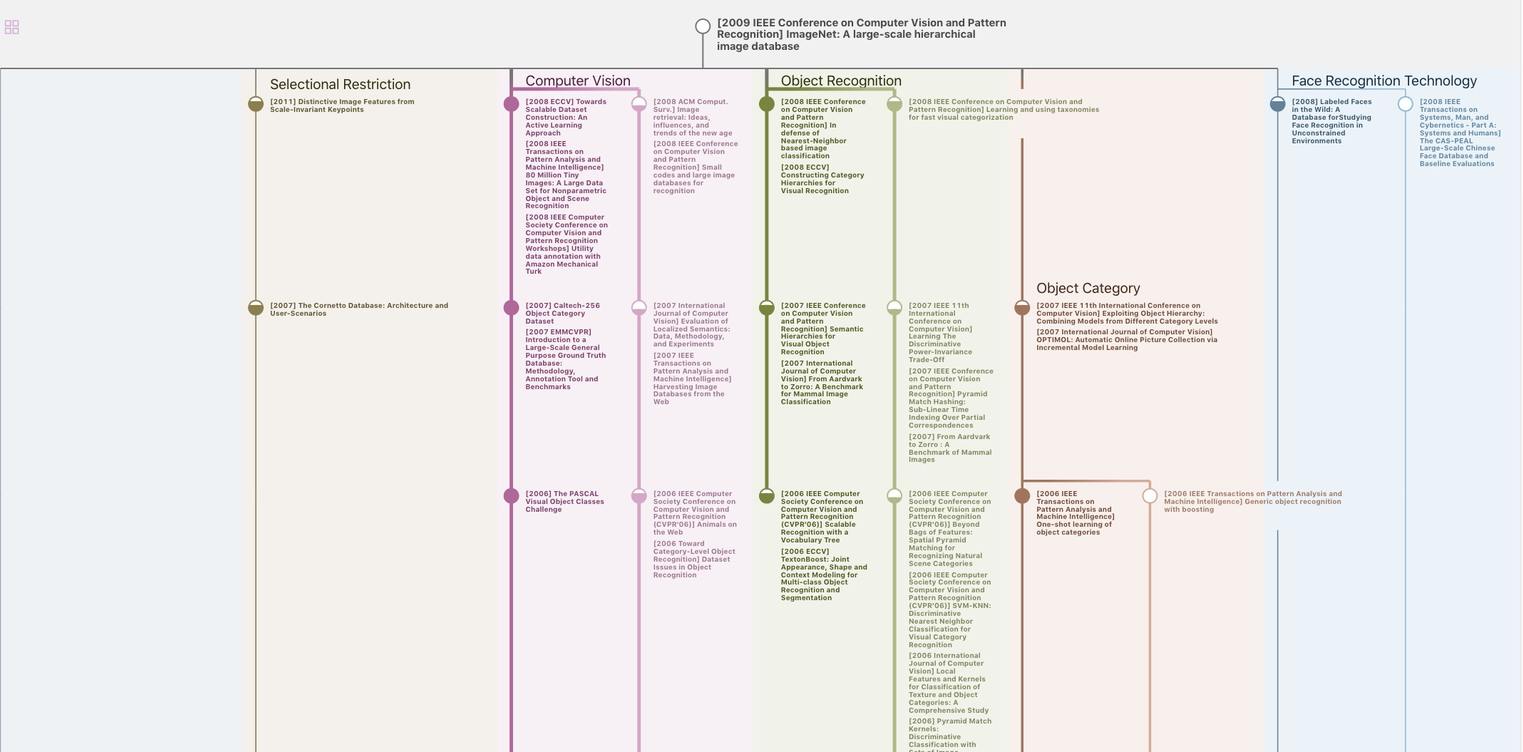
生成溯源树,研究论文发展脉络
Chat Paper
正在生成论文摘要