Non-smooth M-estimator for Maximum Consensus Estimation.
BMVC(2018)
摘要
This paper revisits the application of M-estimators for a spectrum of robust estimation problems in computer vision, particularly with the maximum consensus criterion. Current practice makes use of smooth robust loss functions, e.g. Huber loss, which enables M-estimators to be tackled by such well-known optimization techniques as Iteratively Re-weighted Least Square (IRLS). When consensus maximization is used as loss function for M-estimators, however, the optimization problem becomes non-smooth. Our paper proposes an approach to resolve this issue. Based on the Alternating Direction Method of Multiplier (ADMM) technique, we develop a deterministic algorithm that is provably convergent, which enables the maximum consensus problem to be solved in the context of M-estimator. We further show that our algorithm outperforms other differentiable robust loss functions that are currently used by many practitioners. Notably, the proposed method allows the sub-problems to be solved efficiently in parallel, thus entails it to be implemented in distributed settings.
更多查看译文
关键词
Huber loss,Consensus,M-estimator,Optimization problem,Deterministic algorithm,Iterative method,Maximization,Context (language use),Mathematical optimization,Computer science
AI 理解论文
溯源树
样例
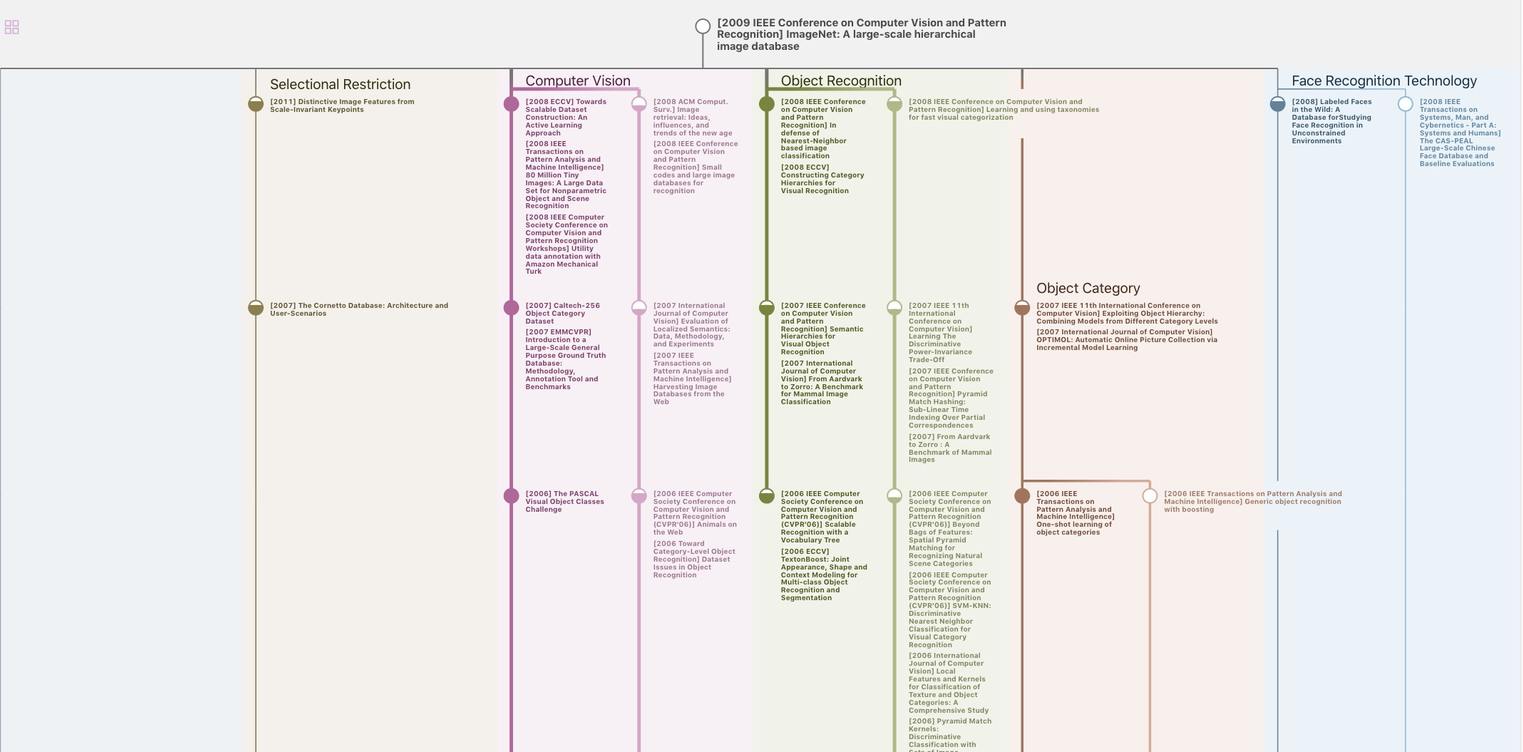
生成溯源树,研究论文发展脉络
Chat Paper
正在生成论文摘要