Identifying Agile Waveforms with Neural Networks
2018 21ST INTERNATIONAL CONFERENCE ON INFORMATION FUSION (FUSION)(2018)
摘要
With the advent of widespread digital technology, modern radar and communication systems have grown more complex and agile, rendering them difficult to adequately document and identify. The traditional solution of comparing incoming signals to a library of known waveforms is therefore becoming unworkable. The authors present two solutions to the problem of modern radio frequency (RF) waveform identification: a deep neural network and a recurrent neural network using GRUs. Both networks are designed to fuse together an arbitrary number of agile RF pulses and identify the emitter that produced them. Compared to a naïve DNN approach that simply averaged together pulses before classification, our solution adds a pre-projection step, which preserves information about sequential agility, even after averaging across pulses. After being trained against a set of 15 highly ambiguous emitters, the naïve DNN identified 52.2 % of test waveforms, our DNN with projection identified 72.3 %, and our RNN solution identified 84.8%.
更多查看译文
关键词
RNN solution,neural networks,communication systems,incoming signals,deep neural network,recurrent neural network,agile RF pulses,naïve DNN approach,sequential agility,digital technology,radar systems,agile waveforms,radio frequency waveform identification,GRUs
AI 理解论文
溯源树
样例
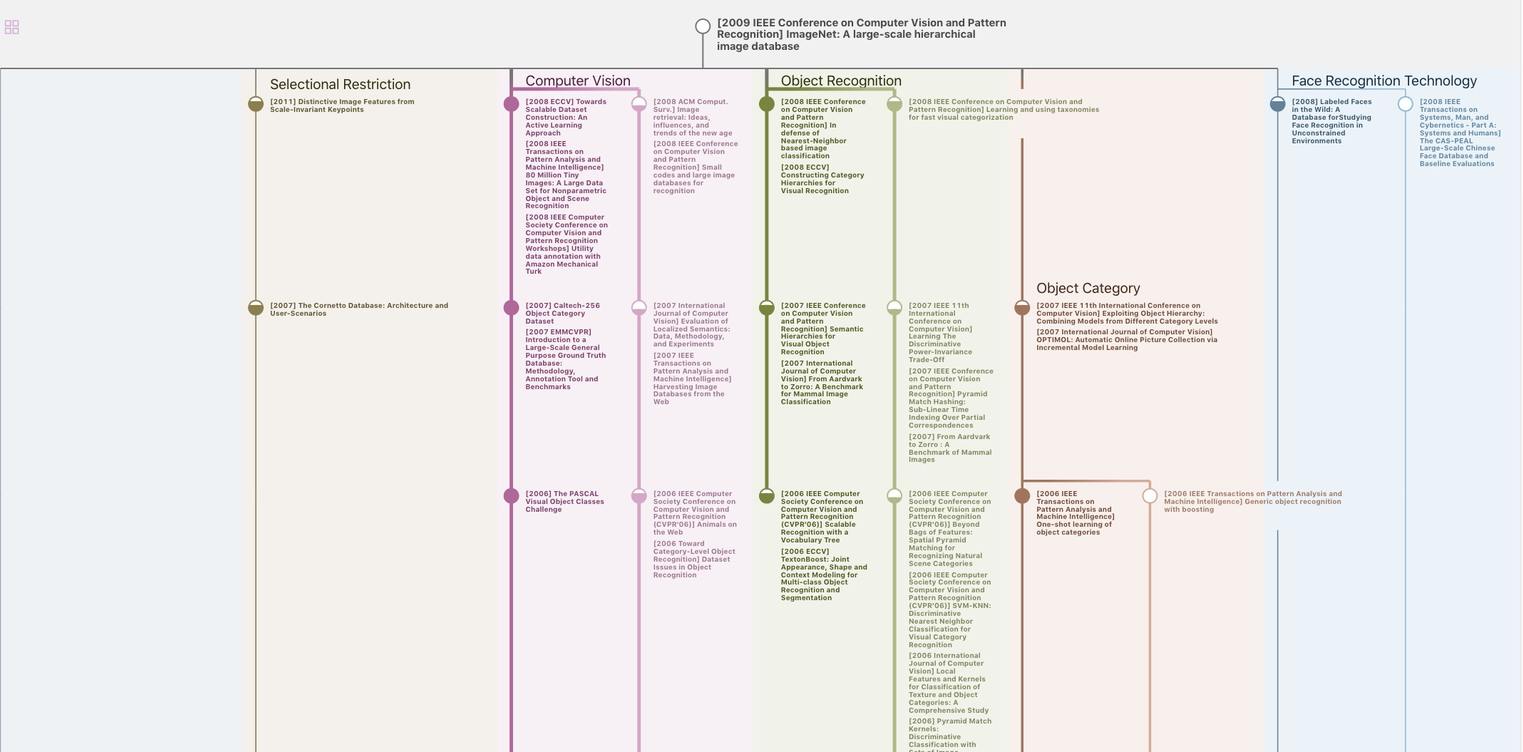
生成溯源树,研究论文发展脉络
Chat Paper
正在生成论文摘要