Learning Modes Of Within-Hand Manipulation
2018 IEEE INTERNATIONAL CONFERENCE ON ROBOTICS AND AUTOMATION (ICRA)(2018)
摘要
In this work, we investigate methods to detect four phenomena (modes) that occur during prehensile fingertip-based within-hand manipulation without the use of tactile sensors. By using actuator states and visual data, we aim to recognize different modes of operation such as interpreting if the hand is about to drop the object, if the object will begin to slide on the fingers, or if the system is at or near a singularity. For this purpose, we utilize supervised learning techniques, which allow us to detect the modes without the use of a mechanical model of the system. We analyze the individual roles of specific features available through both the actuator and visual data, and identify the ones that have the most significance for detecting the operation modes. Our results show classification performance of 96% (using either Extra Trees, Gradient Boosting, or SVM) when using combined actuator and visual features. Interestingly, we were able to achieve a 94% classification rate using only actuator information, and 93% using only visual information. Overall, the classifiers identified actuator positions, actuator loads, and commanded velocities as the most important features for detecting a mode. These results have implications for enabling the control of within-hand manipulation movements utilizing a minimal amount of sensory information without a model of the hand/object system.
更多查看译文
关键词
learning modes,prehensile fingertip-based within-hand manipulation,tactile sensors,actuator states,visual data,supervised learning techniques,classification performance,Extra Trees,Gradient Boosting,visual features,classification rate,actuator loads,within-hand manipulation movements,hand/object system
AI 理解论文
溯源树
样例
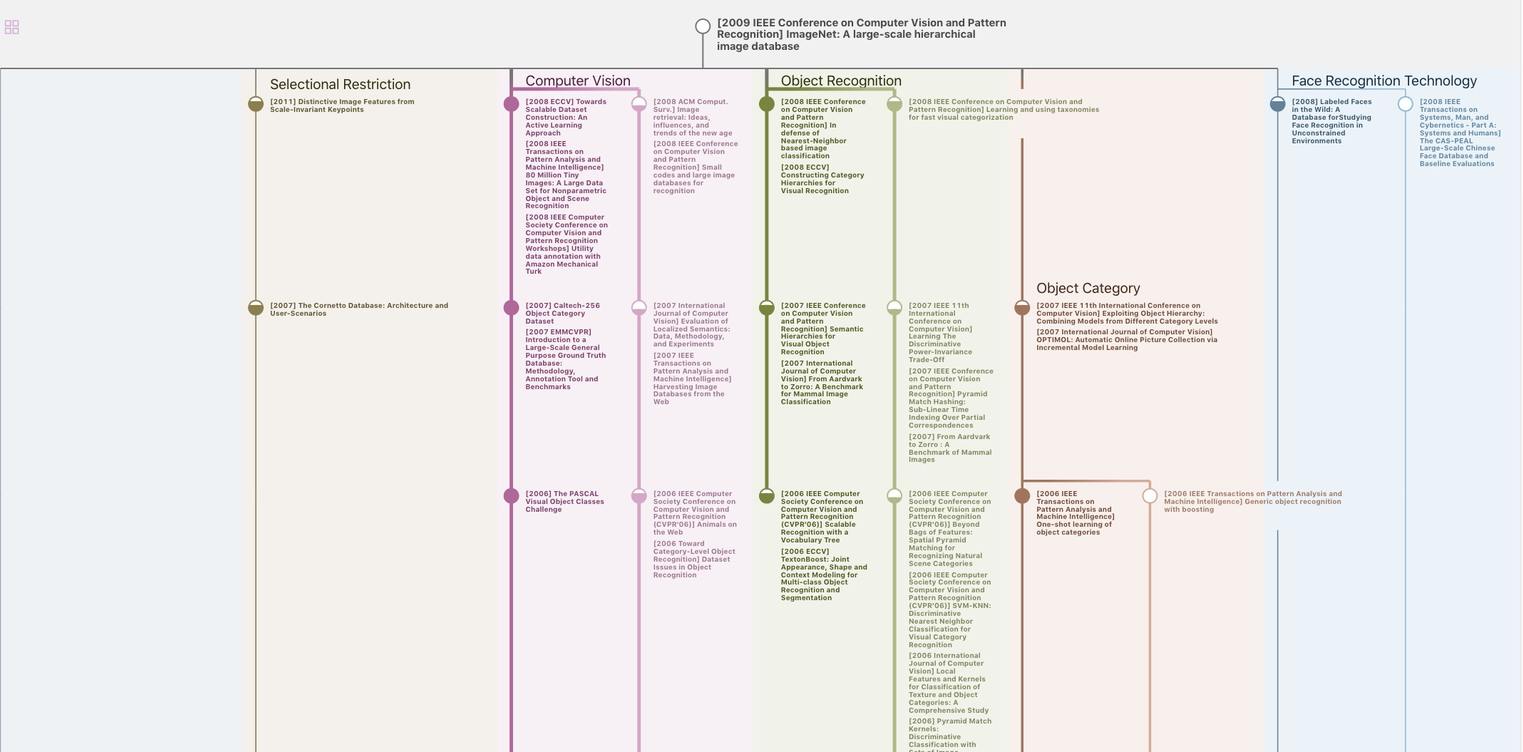
生成溯源树,研究论文发展脉络
Chat Paper
正在生成论文摘要