Auditory Filterbank Learning For Temporal Modulation Features In Replay Spoof Speech Detection
19TH ANNUAL CONFERENCE OF THE INTERNATIONAL SPEECH COMMUNICATION ASSOCIATION (INTERSPEECH 2018), VOLS 1-6: SPEECH RESEARCH FOR EMERGING MARKETS IN MULTILINGUAL SOCIETIES(2018)
摘要
In this paper, we present a standalone replay spoof speech detection (SSD) system to classify the natural vs. replay speech. The replay speech spectrum is known to be affected in the higher frequency range. In this context, we propose to exploit an auditory filterbank learning using Convolutional Restricted Boltzmann Machine (ConvRBM) with the pre-emphasized speech signals. Temporal modulations in amplitude (AM) and frequency (FM) are extracted from the ConvRBM subbands using the Energy Separation Algorithm (ESA). ConvRBM-based short-time AM and FM features are developed using cepstral processing, denoted as AM-ConvRBM-CC and FM-ConvRBM-CC. Proposed temporal modulation features performed better than the baseline Constant-Q Cepstral Coefficients (CQCC) features. On the evaluation set, an absolute reduction of 7.48 % and 5.28 % in Equal Error Rate (EER) is obtained using AM-ConvRBM-CC and FM-ConvRBM-CC, respectively compared to our CQCC baseline. The best results are achieved by combining scores from AM and FM cues (0.82 % and 8.89 % EER for development and evaluation set, respectively). The statistics of AM-FM features are analyzed to understand the performance gap and complementary information in both the features.
更多查看译文
关键词
ConvRBM, amplitude and frequency modulations (AM-FM), replay spoof speech detection
AI 理解论文
溯源树
样例
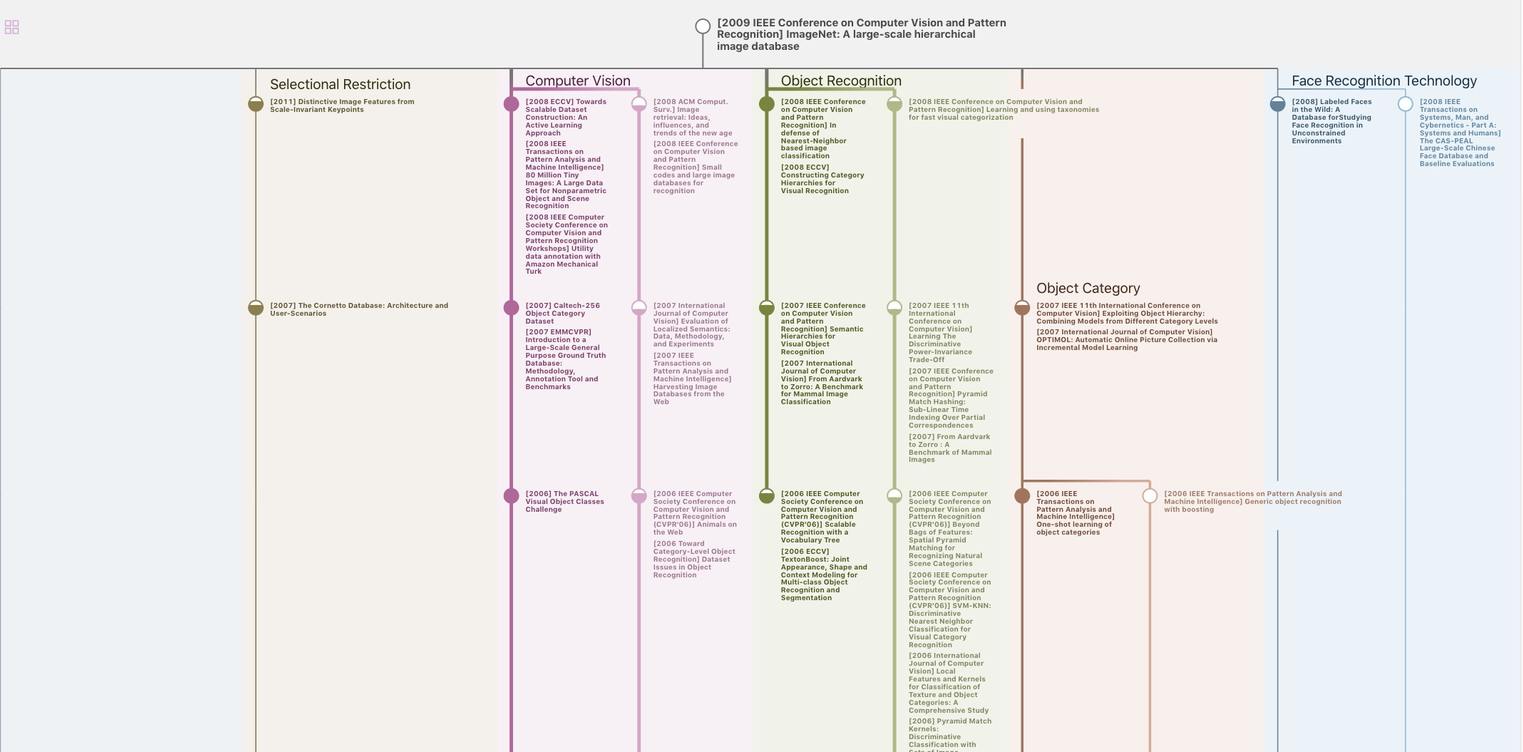
生成溯源树,研究论文发展脉络
Chat Paper
正在生成论文摘要