Predicting Arousal And Valence From Waveforms And Spectrograms Using Deep Neural Networks
19TH ANNUAL CONFERENCE OF THE INTERNATIONAL SPEECH COMMUNICATION ASSOCIATION (INTERSPEECH 2018), VOLS 1-6: SPEECH RESEARCH FOR EMERGING MARKETS IN MULTILINGUAL SOCIETIES(2018)
摘要
Automatic recognition of spontaneous emotion in conversational speech is an important yet challenging problem. In this paper, we propose a deep neural network model to track continuous emotion changes in the arousal-valence two-dimensional space by combining inputs from raw waveform signals and spectrograms, both of which have been shown to be useful in the emotion recognition task. The neural network architecture contains a set of convolutional neural network (CNN) layers and bidirectional long short-term memory (BLSTM) layers to account for both temporal and spectral variation and model contextual content. Experimental results of predicting valence and arousal on the SEMAINE database and the RECOLA database show that the proposed model significantly outperforms model using hand-engineered features, by exploiting waveforms and spectrograms as input. We also compare the effects of waveforms vs. spectrograms and find that waveforms are better at capturing arousal, while spectrograms are better at capturing valence. Moreover, combining information from both inputs provides further improvement to the performance.
更多查看译文
关键词
speech emotion recognition, computational paralinguistics, deep learning
AI 理解论文
溯源树
样例
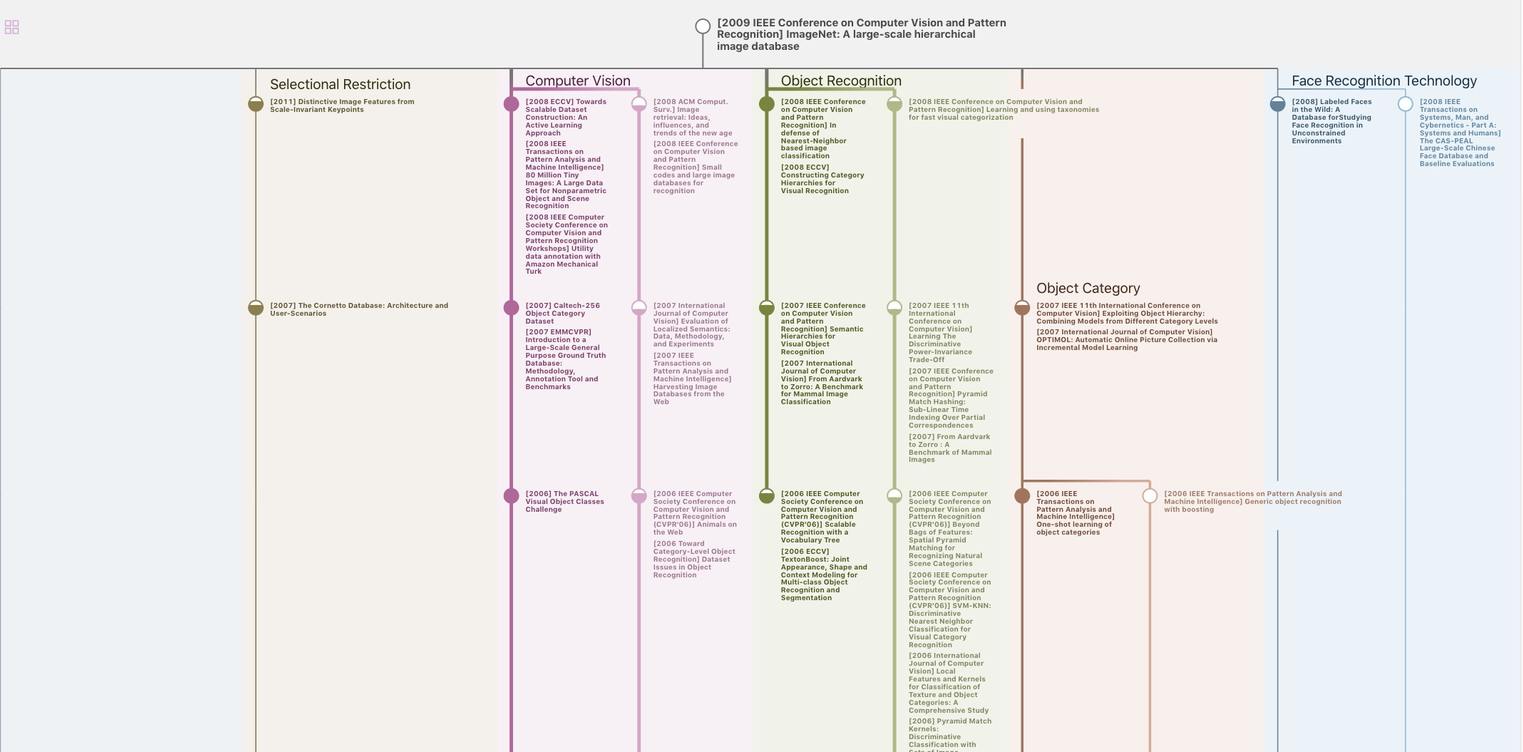
生成溯源树,研究论文发展脉络
Chat Paper
正在生成论文摘要