Using Voice Quality Supervectors For Affect Identification
19TH ANNUAL CONFERENCE OF THE INTERNATIONAL SPEECH COMMUNICATION ASSOCIATION (INTERSPEECH 2018), VOLS 1-6: SPEECH RESEARCH FOR EMERGING MARKETS IN MULTILINGUAL SOCIETIES(2018)
摘要
The voice quality of speech sounds often conveys perceivable information about the speaker's affect. This study proposes perceptually important voice quality features to recognize affect represented in speech excerpts from individuals with mental, neurological, and/or physical disabilities. The voice quality feature set consists of F0, harmonic amplitude differences between the first, second, fourth harmonics and the harmonic near 2 kHz, the center frequency and amplitudes of the first 3 formants, and cepstral peak prominence. The feature distribution of each utterance was represented with a supervector, and the Gaussian mixture model and support vector machine classifiers were used for affect classification. Similar classification systems using the MFCCs and ComParEl 6 feature set were implemented. The systems were fused by taking the confidence mean of the classifiers. Applying the fused system to the Interspeech 2018 Atypical Affect subchallenge task resulted in unweighted average recalls of 43.9% and 41.0% on the development and test dataset, respectively. Additionally, we investigated clusters obtained by unsupervised learning to address gender-related differences.
更多查看译文
关键词
emotion recognition, human-computer interaction, computational paralinguistics
AI 理解论文
溯源树
样例
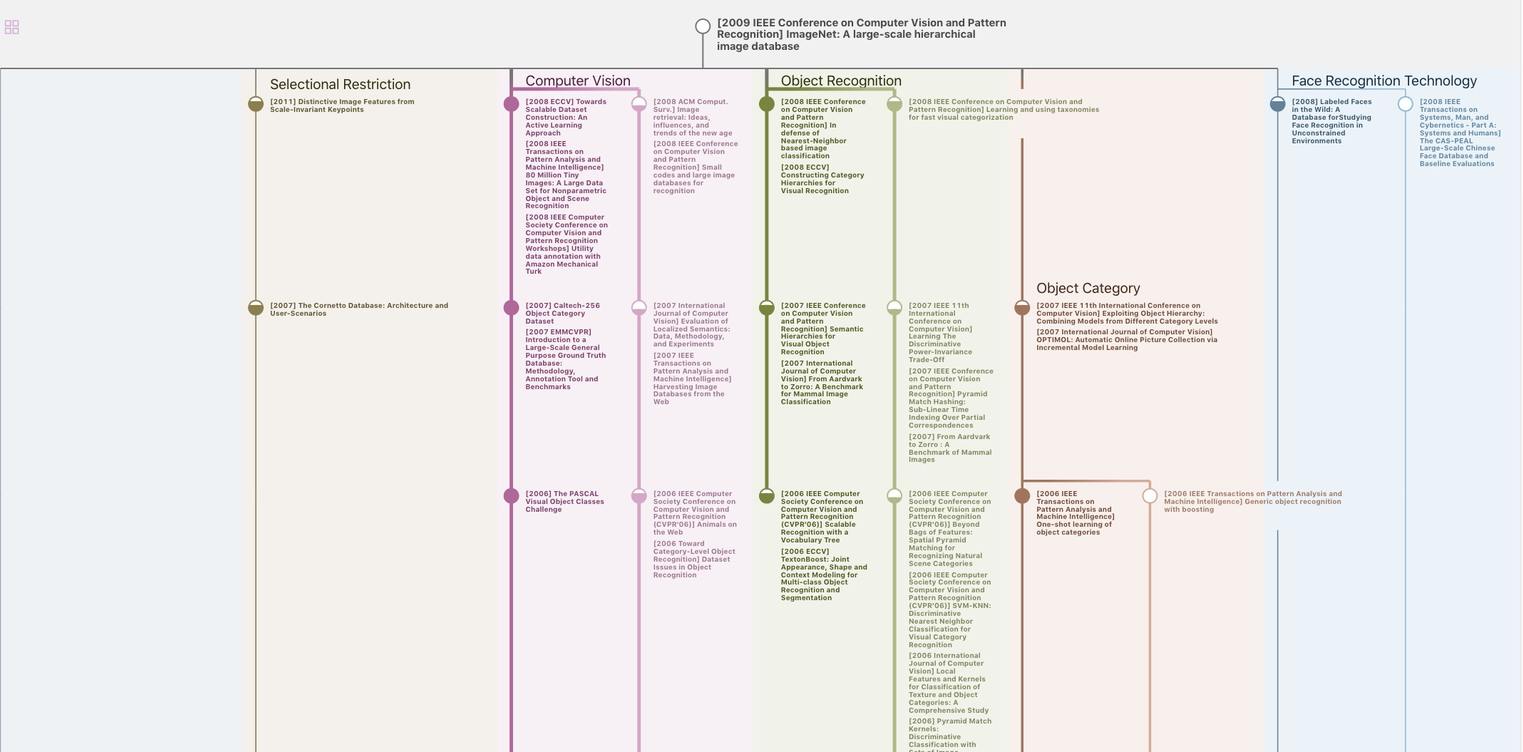
生成溯源树,研究论文发展脉络
Chat Paper
正在生成论文摘要