Filter Sampling And Combination Cnn (Fsc-Cnn): A Compact Cnn Model For Small-Footprint Asr Acoustic Modeling Using Raw Waveforms
19TH ANNUAL CONFERENCE OF THE INTERNATIONAL SPEECH COMMUNICATION ASSOCIATION (INTERSPEECH 2018), VOLS 1-6: SPEECH RESEARCH FOR EMERGING MARKETS IN MULTILINGUAL SOCIETIES(2018)
摘要
Learning an ASR acoustic model directly from raw waveforms using CNNs has proved to be effective, where convolutional layers with learnable filters are able to automatically extract useful features. However, these filters, with independent parameters, can be highly redundant resulting in inefficient systems. In this paper, we propose a novel method to generate CNN filter parameters by first sampling from a low-dimensional parameter space and then using a trainable scalar vector to perform a linear combination. This filter sampling and combination method (denoted as FSC) not only naturally enforces parameter sharing in the low-dimensional sampling space, but also adds to the learning capacity of filters. The FSC-CNN model has a significantly smaller number of parameters and is more efficient compared to conventional CNN models, which makes it feasible for small-footprint ASR. Experimental results on the WSJ LVCSR task show that FSC-CNNs are able to achieve a WER of 3.67 with a standard decoder set-up with only 1.19M nonlinear layer parameters (better than a strong baseline CNN model with 3.2x more parameters). It also outperforms a CNN model with a similar number of parameters by a relative improvement of 10.26%.
更多查看译文
关键词
speech recognition, small-footprint, acoustic modeling, raw audio, filter sampling and combination, CNNs
AI 理解论文
溯源树
样例
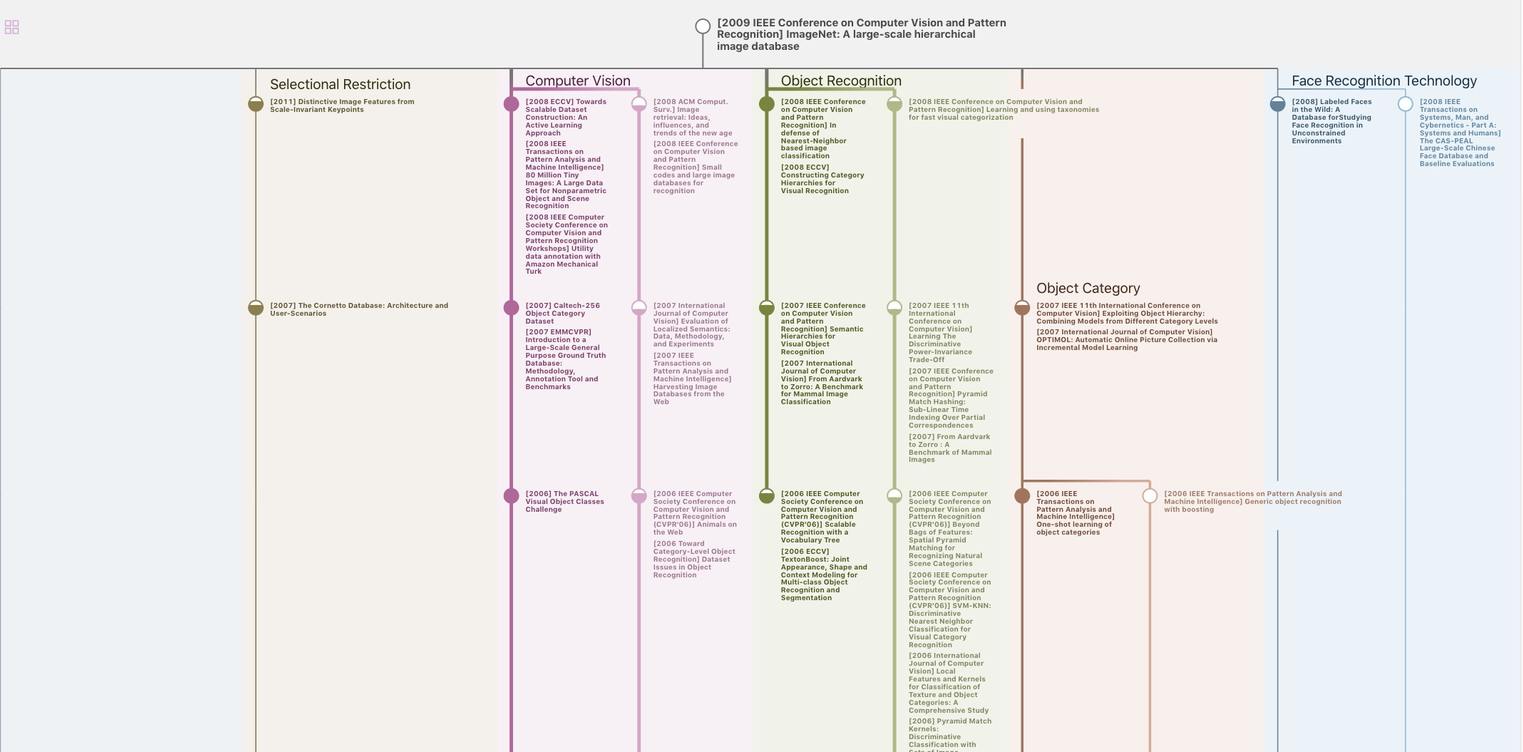
生成溯源树,研究论文发展脉络
Chat Paper
正在生成论文摘要