Diversity of a User's Friend Circle in OSNs and Its Use for Profiling.
Lecture notes in computer science(2018)
关键词
Online social network,Usage purpose,Diversity
AI 理解论文
溯源树
样例
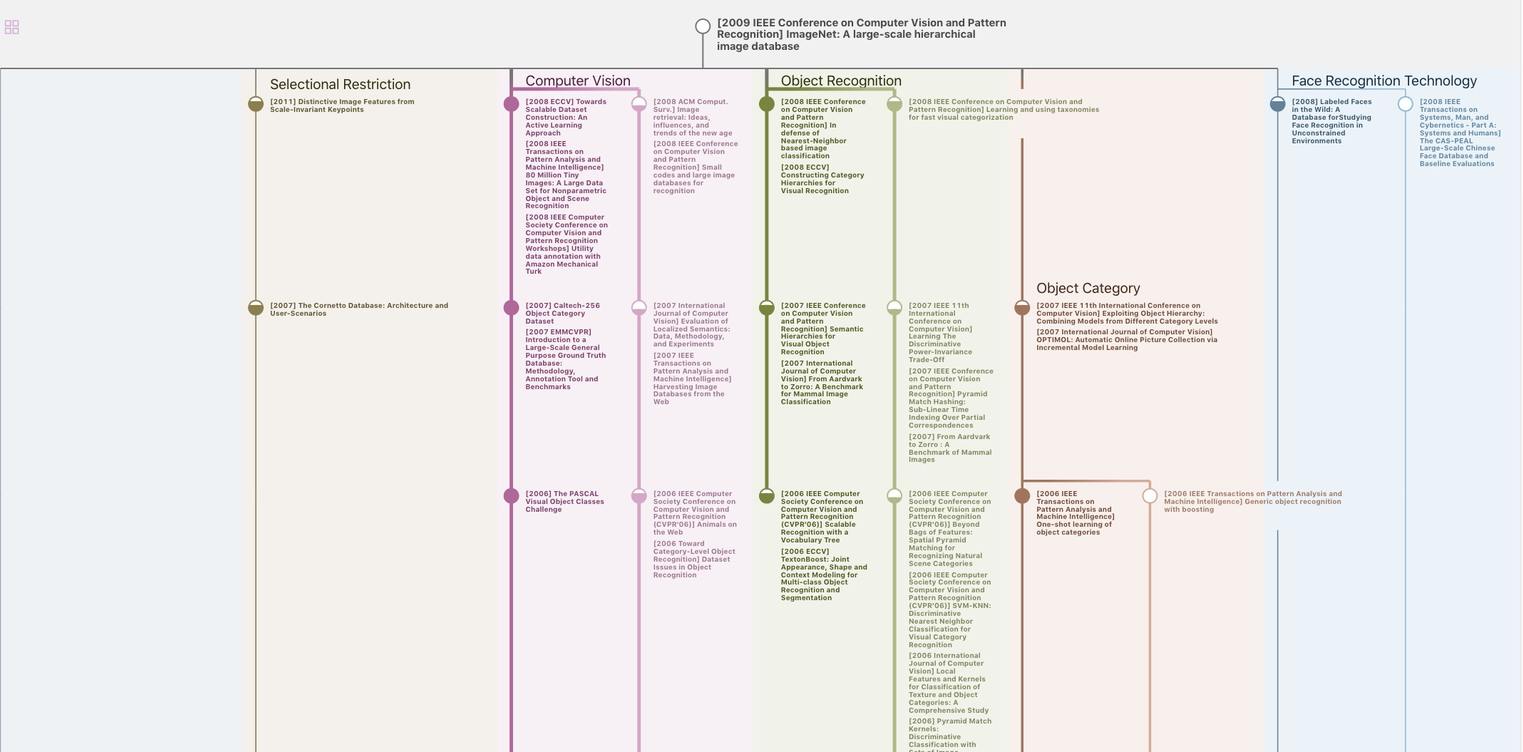
生成溯源树,研究论文发展脉络
Chat Paper
正在生成论文摘要
Lecture notes in computer science(2018)