Knowledge Transfer In Permutation Invariant Training For Single-Channel Multi-Talker Speech Recognition
2018 IEEE INTERNATIONAL CONFERENCE ON ACOUSTICS, SPEECH AND SIGNAL PROCESSING (ICASSP)(2018)
摘要
This paper proposes a framework that combines teacher-student training and permutation invariant training (PIT) for single-channel multi-talker speech recognition. In contrast to most of conventional teacher-student training methods that aim at compressing the model, the proposed method distills knowledge from the single-talker model to improve the multi-talker model in the PIT framework. The inputs to the teacher and student networks are the single-talker clean speech and the multi-talker mixed speech, respectively. The knowledge is transferred to the student through the soft labels generated by the teacher. Furthermore, the ensemble of multiple teachers is exploited with a progressive training scheme to further improve the system. In this framework it is easy to take advantage of data augmentation and perform domain adaptation for multi-talker speech recognition using only untranscribed data. The proposed techniques were evaluated on artificially mixed two-talker AMI speech data. The experimental results show that the teacher-student training can cut the word error rate (WER) by relative 20% against the baseline PIT model. We also evaluated our unsupervised domain adaptation method on an artificially mixed WSJO corpus and achieved relative 30% WER reduction against the AMI PIT model.
更多查看译文
关键词
permutation invariant training, knowledge distillation, multi-talker speech recognition, unsupervised training
AI 理解论文
溯源树
样例
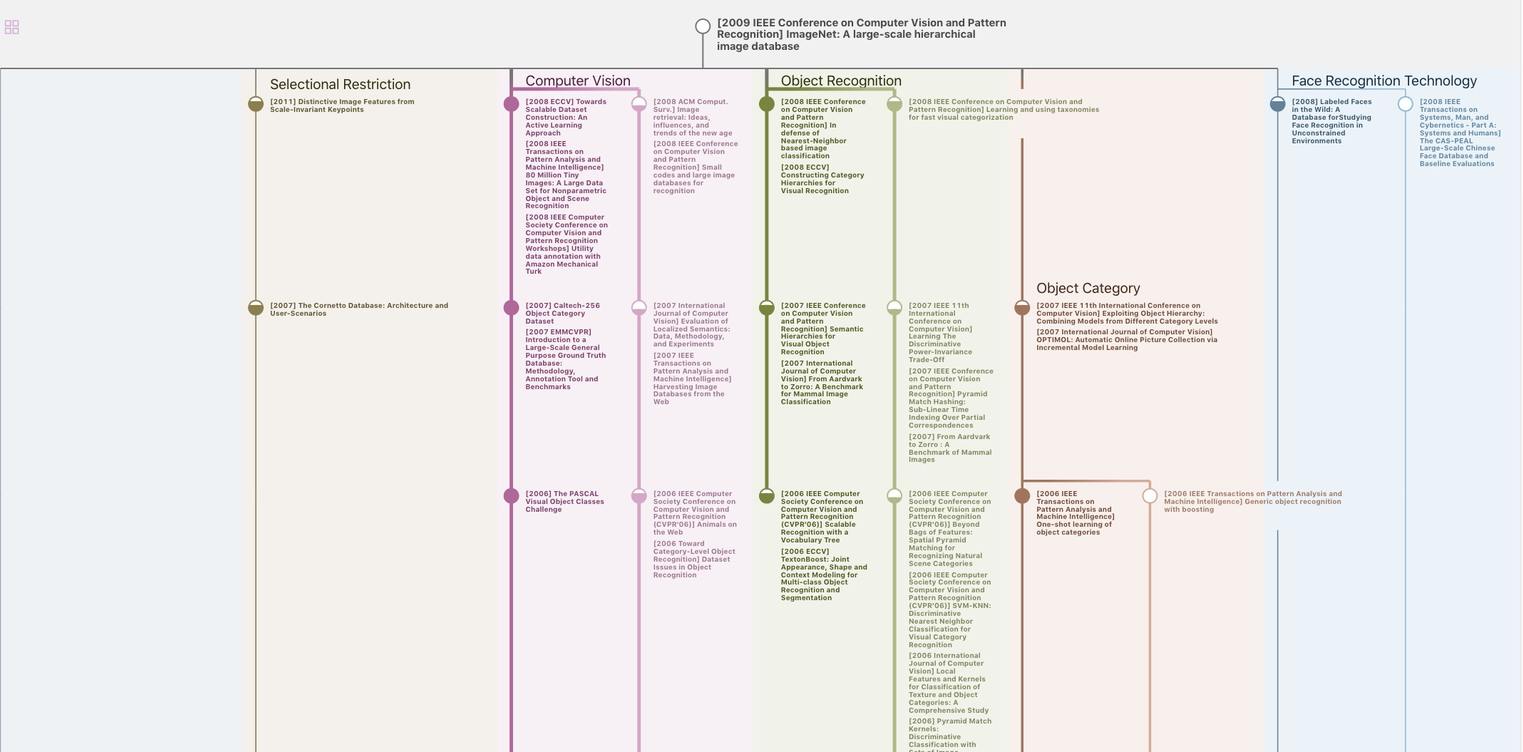
生成溯源树,研究论文发展脉络
Chat Paper
正在生成论文摘要