Efficient Circulant Matrix Construction And Implementation For Compressed Sensing
2018 IEEE INTERNATIONAL CONFERENCE ON ACOUSTICS, SPEECH AND SIGNAL PROCESSING (ICASSP)(2018)
摘要
The design of measurement matrices is an important part in compressed sensing (CS). Random matrices superior to incoherence are considered to be optimal measurement matrices to achieve successful recovery. However, they are deficient in memory cost. Structure matrices like circulant matrices are preferred for low-memory cost. Nevertheless, their recovery performance is greatly damaged because of element coherence. In this paper, a new method called different-spaced selection & different-spaced flipping (DSS & DSF) is proposed to modify structure matrices. Based on circulant matrices, regular extraction and symbol flipping imposed on columns of measurement matrices can increase randomness to a large scale. As a result, not only near optimal recovery but also much less memory cost can be achieved. Compared with Gaussian random matrices, the memory cost can be reduced to 4% when measurement matrices based on circulant matrices are in 128 x 512 dimensions. An efficient hardware design and VLSI implementation are also presented at the end of this paper.
更多查看译文
关键词
Measurement matrix, compressed sensing (CS), structure matrix, memory cost, hardware implementation
AI 理解论文
溯源树
样例
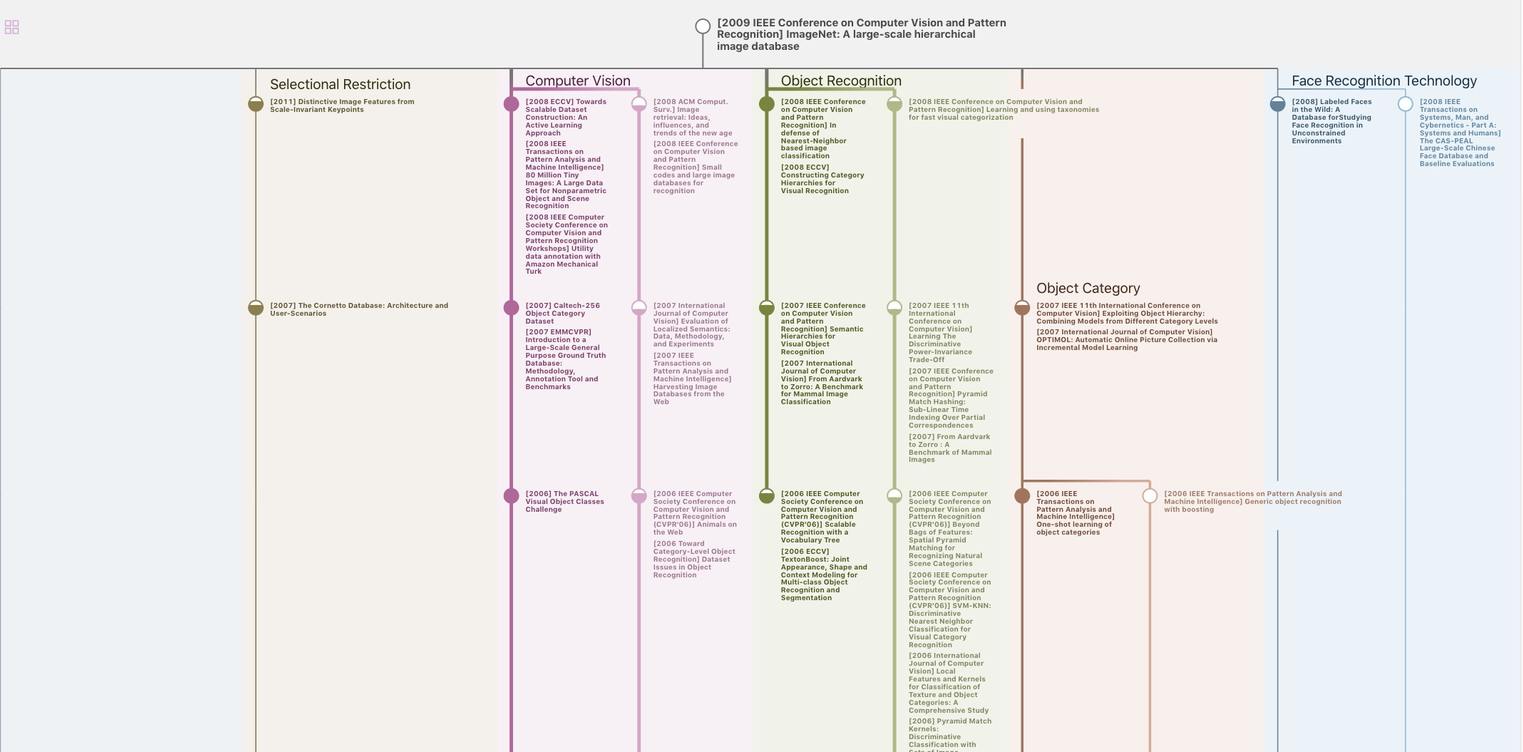
生成溯源树,研究论文发展脉络
Chat Paper
正在生成论文摘要