A Deep Neural Network Approach for Time-Of- Arrival Estimation in Multipath Channels.
ICASSP(2018)
摘要
Attaining accurate estimation of a signal time-of-arrival (TOA) in dense multipath channels is very challenging. This problem was traditionally solved with signal processing techniques. In this paper, a novel deep convolutional neural networks (DCNN) TOA estimator is developed. The DCNN was trained with synthetically generates multipath channel realizations based on statistical modeling, and then tested on real-life measurements from indoor environments. It is shown that the DCNN attains the performance of state-of-the-art signal processing based estimators (maximum likelihood performance), and has the advantage that it does not require knowledge of the channel statistics nor the knowledge of the transmitted signal waveform. This work inspires further study on the applicability of neural networks to other related problems, which have been traditionally solved with signal processing methods.
更多查看译文
关键词
time-of-arrival estimation,transmitted signal waveform,channel statistics,maximum likelihood performance,deep convolutional neural networks TOA estimator,dense multipath channels,signal time-of-arrival,deep neural network approach
AI 理解论文
溯源树
样例
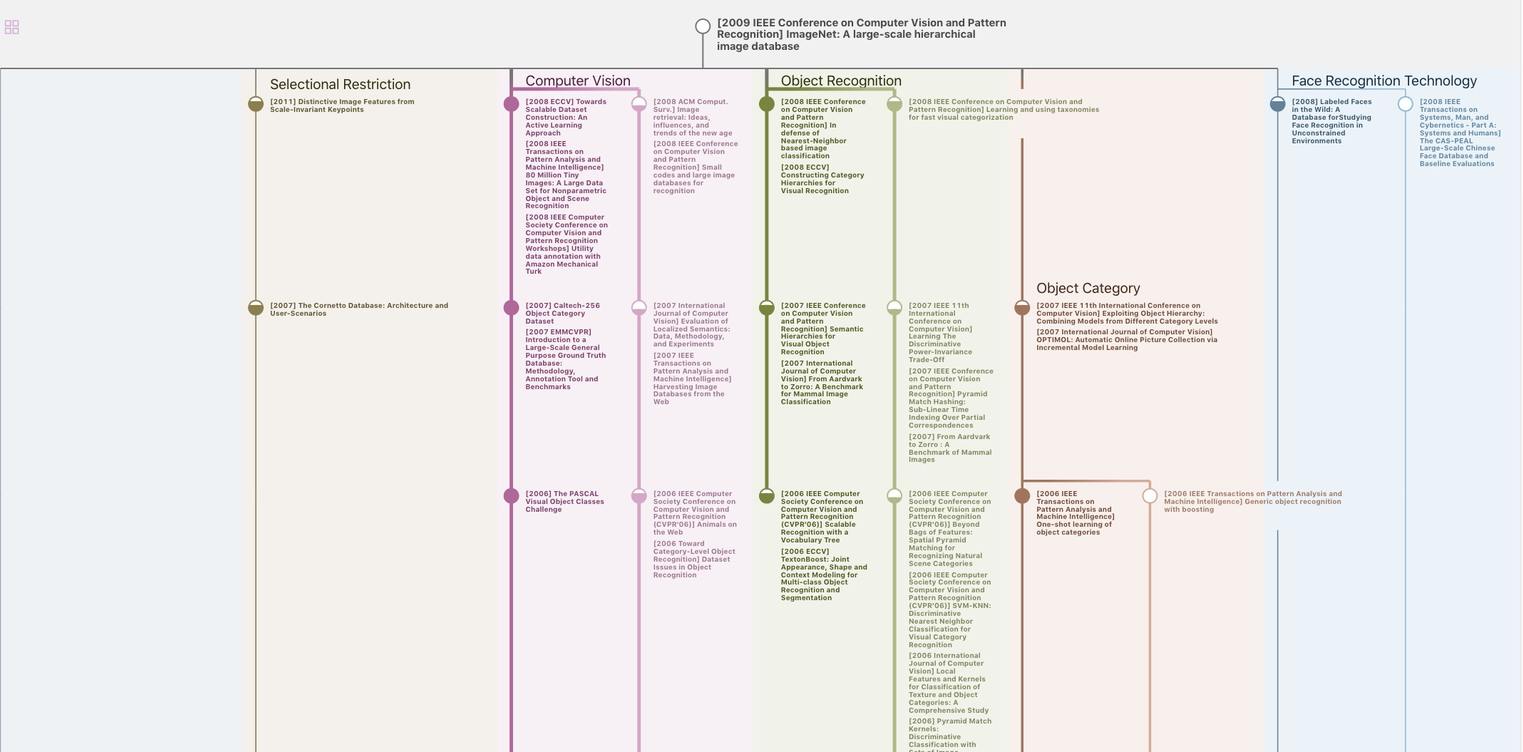
生成溯源树,研究论文发展脉络
Chat Paper
正在生成论文摘要