Fooling End-to-end Speaker Verification by Adversarial Examples
2018 IEEE INTERNATIONAL CONFERENCE ON ACOUSTICS, SPEECH AND SIGNAL PROCESSING (ICASSP)(2018)
摘要
Automatic speaker verification systems are increasingly used as the primary means to authenticate costumers. Recently, it has been proposed to train speaker verification systems using end-to-end deep neural models. In this paper, we show that such systems are vulnerable to adversarial example attack. Adversarial examples are generated by adding a peculiar noise to original speaker examples, in such a way that they are almost indistinguishable from the original examples by a human listener. Yet, the generated waveforms, which sound as speaker A can be used to fool such a system by claiming as if the waveforms were uttered by speaker B. We present white-box attacks on an end-to-end deep network that was either trained on YOHO or NTIMIT. We also present two black-box attacks: where the adversarial examples were generated with a system that was trained on YOHO, but the attack is on a system that was trained on NTIMIT; and when the adversarial examples were generated with a system that was trained on Mel-spectrum feature set, but the attack is on a system that was trained on MFCC. Results suggest that the accuracy of the attacked system was decreased and the false-positive rate was dramatically increased.
更多查看译文
关键词
Automatic speaker verification, adversarial examples
AI 理解论文
溯源树
样例
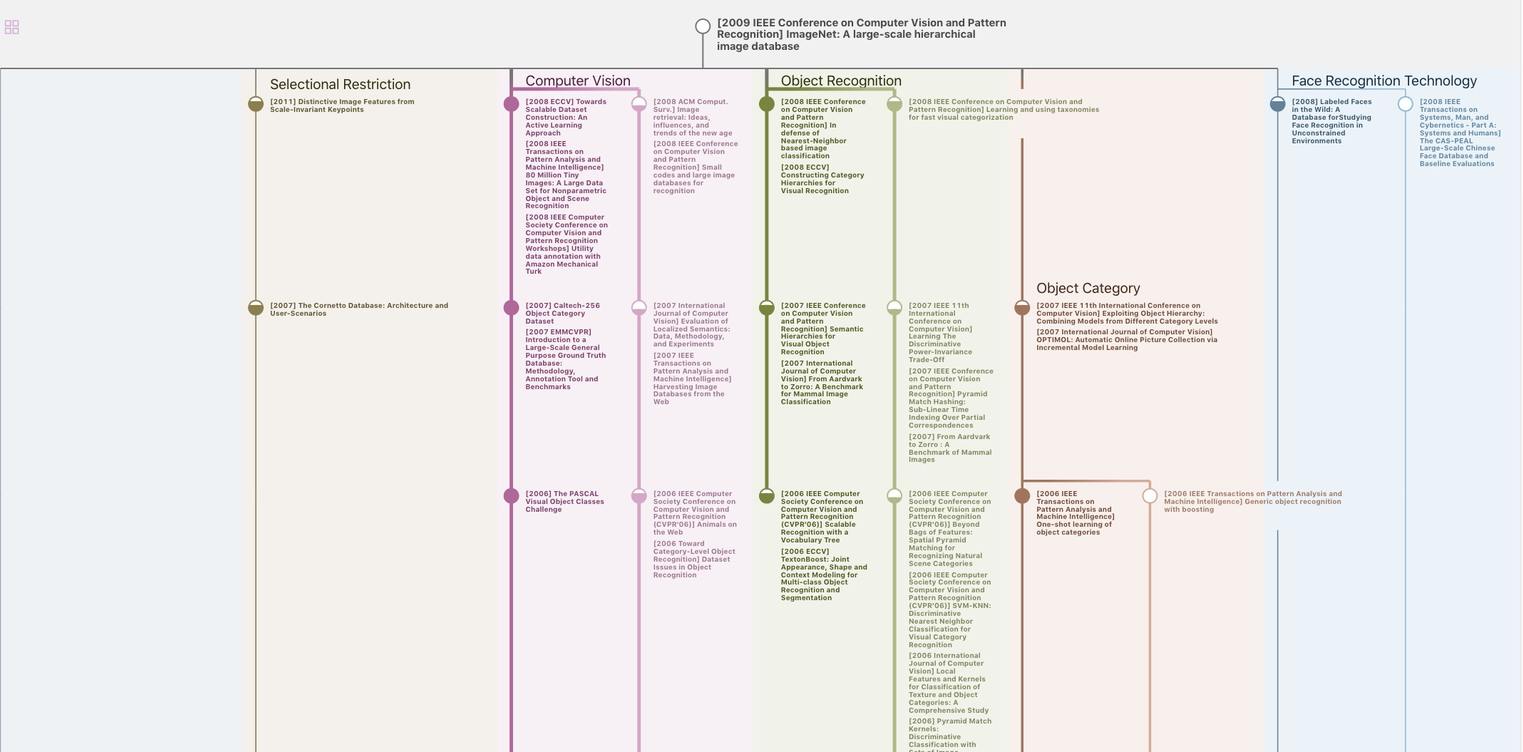
生成溯源树,研究论文发展脉络
Chat Paper
正在生成论文摘要