Sampled Connectionist Temporal Classification
2018 IEEE INTERNATIONAL CONFERENCE ON ACOUSTICS, SPEECH AND SIGNAL PROCESSING (ICASSP)(2018)
摘要
This article introduces and evaluates Sampled Connectionist Temporal Classification (CTC) which connects the CTC criterion to the Cross Entropy (CE) objective through sampling. Instead of computing the logarithm of the sum of the alignment path likelihoods, at each training step the sampled CTC only computes the CE loss between the sampled alignment path and model posteriors. It is shown that the sampled CTC objective is an unbiased estimator of an upper bound for the CTC loss, thus minimization of the sampled CTC is equivalent to the minimization of the upper bound of the CTC objective. The definition of the sampled CTC objective has the advantage that it is scalable computationally to the massive datasets using accelerated computation machines. The sampled CTC is compared with CTC in two large-scale speech recognition tasks and it is shown that sampled CTC can achieve similar WER performance of the best CTC baseline in about one fourth of the training time of the CTC baseline.
更多查看译文
关键词
Deep Neural Networks, CTC, Cross Entropy, Sampling
AI 理解论文
溯源树
样例
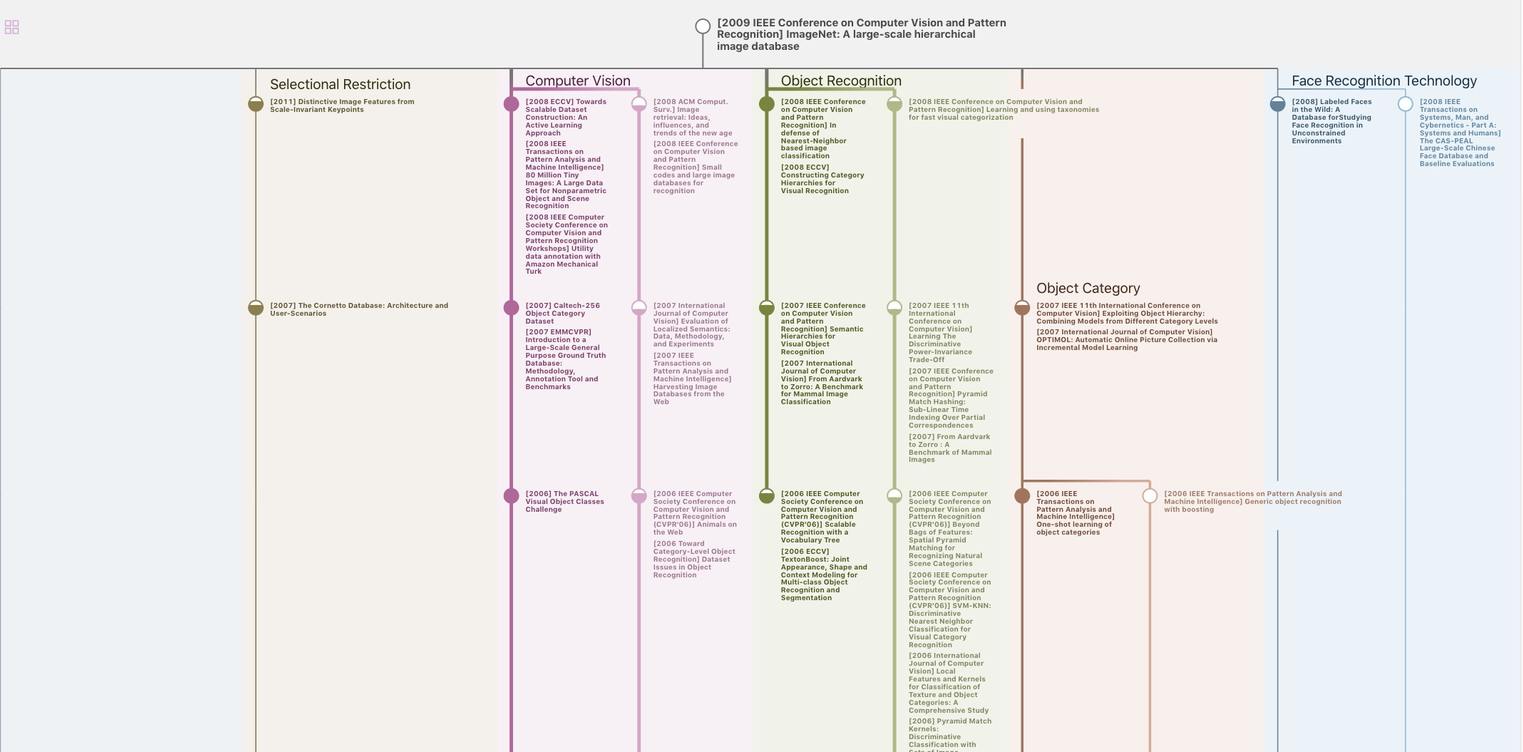
生成溯源树,研究论文发展脉络
Chat Paper
正在生成论文摘要