Radmm: Recurrent Adaptive Mixture Model With Applications To Domain Robust Language Modeling
2018 IEEE INTERNATIONAL CONFERENCE ON ACOUSTICS, SPEECH AND SIGNAL PROCESSING (ICASSP)(2018)
摘要
We present a new architecture and a training strategy for an adaptive mixture of experts with applications to domain robust language modeling. The proposed model is designed to benefit from the scenario where the training data are available in diverse domains as is the case for YouTube speech recognition. The two core components of our model are an ensemble of parallel long short-term memory (LSTM) expert layers for each domain and another LSTM based network which generates state dependent mixture weights for combining expert LSTM states by linear interpolation. The resulting model is a recurrent adaptive mixture model (RADMM) of domain experts. We train our model on 4.4B words from YouTube speech recognition data. We report results on the YouTube speech recognition test set. Compared with a background LSTM model, we obtain up to 12% relative improvement in perplexity and an improvement in word error rate from 12.3% to 12.1% while using a lattice rescoring with strong pruning.
更多查看译文
关键词
language modeling, neural networks, speech recognition, mixture of experts, domain adaptation
AI 理解论文
溯源树
样例
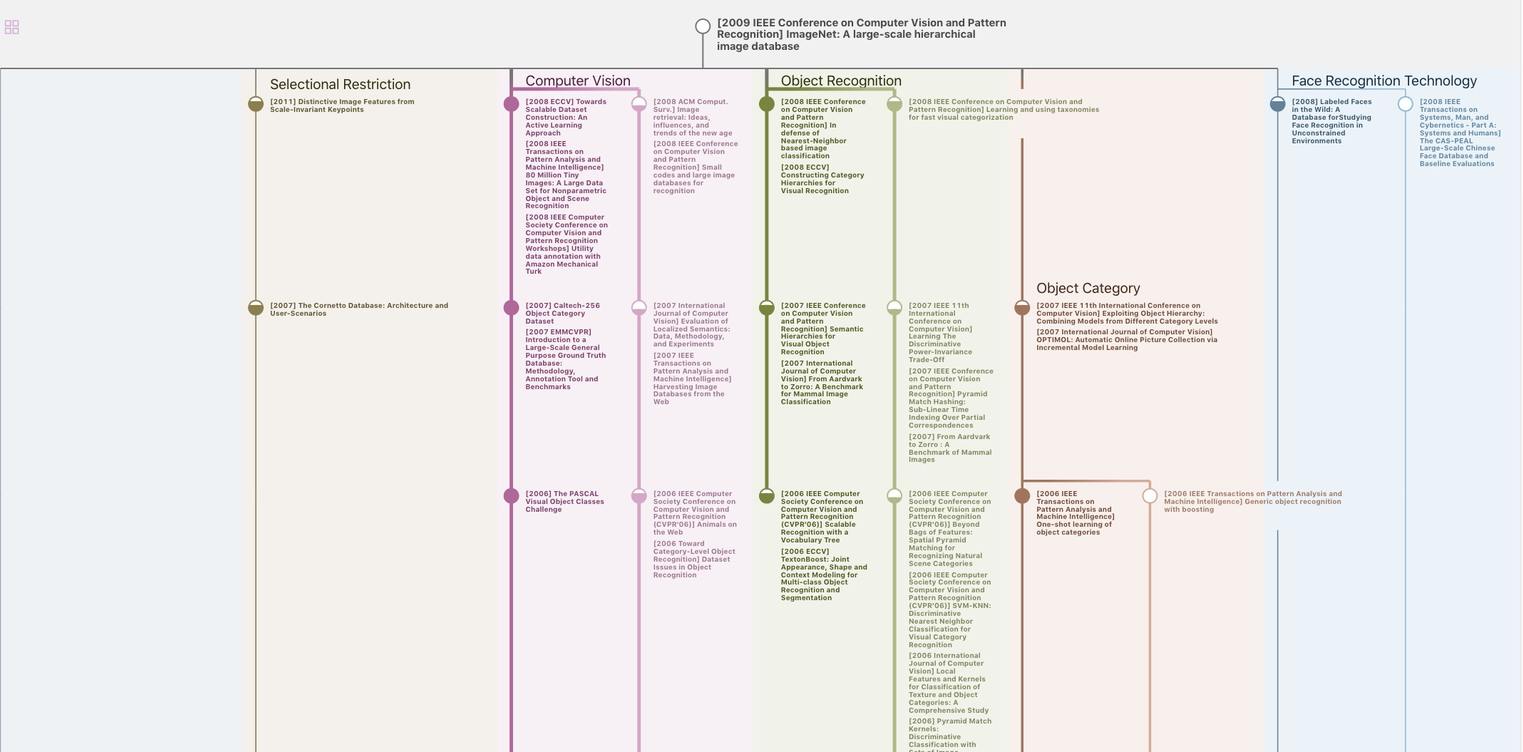
生成溯源树,研究论文发展脉络
Chat Paper
正在生成论文摘要