Modeling Non-Linguistic Contextual Signals In Lstm Language Models Via Domain Adaptation
2018 IEEE INTERNATIONAL CONFERENCE ON ACOUSTICS, SPEECH AND SIGNAL PROCESSING (ICASSP)(2018)
摘要
Language Models (LMs) for Automatic Speech Recognition (ASR) can benefit from utilizing non-linguistic contextual signals in modeling. Examples of these signals include the geographical location of the user speaking to the system and/or the identity of the application (app) being spoken to. In practice, the vast majority of input speech queries typically lack annotations of such signals, which poses a challenge to directly train domain-specific LMs. To obtain robust domain LMs, generally an LM which has been pre-trained on general data will be adapted to specific domains. We propose four domain adaptation schemes to improve the domain performance of Long Short-Term Memory (LSTM) LMs, by incorporating app based contextual signals of voice search queries. We show that most of our adaptation strategies are effective, reducing word perplexity up to 21% relative to a fine-tuned baseline on a held-out domain-specific development set. Initial experiments using a state-of-the-art Italian ASR system show a 3% relative reduction in WER on top of an unadapted 5-gram LM. In addition, human evaluations show significant improvements on sub-domains from using app signals.
更多查看译文
关键词
neural network based language models, language model adaptation, domain adaptation, speech recognition
AI 理解论文
溯源树
样例
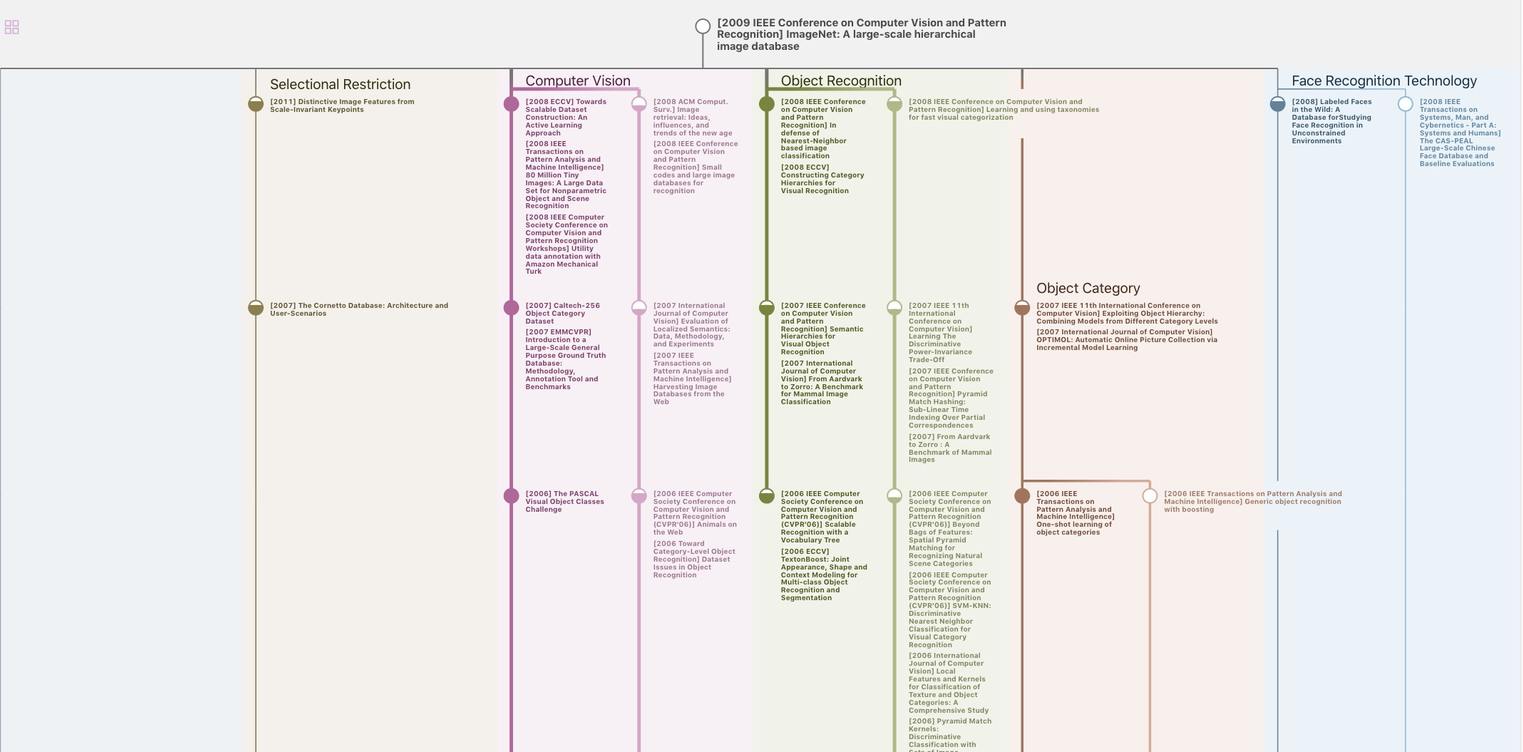
生成溯源树,研究论文发展脉络
Chat Paper
正在生成论文摘要