Mobile Bayesian Spectrum Learning For Heterogeneous Networks
2018 IEEE INTERNATIONAL CONFERENCE ON ACOUSTICS, SPEECH AND SIGNAL PROCESSING (ICASSP)(2018)
摘要
Spectrum sensing in heterogeneous networks is very challenging as it usually requires a large number of static secondary users (SUs) to capture the global spectrum states. In this paper, we tackle the spectrum sensing in heterogeneous networks from a new perspective. We exploit the mobility of multiple SUs to simultaneously collect spatial-temporal spectrum sensing data. Then, we propose a new non-parametric Bayesian learning model, referred to as beta process hidden Markov model to capture the spatio-temporal correlation in the collected spectrum data. Finally, Bayesian inference is carried out to establish the global spectrum picture. Simulation results show that the proposed algorithm can achieve a significant spectrum sensing performance improvement in terms of receiver operating characteristic curve and detection accuracy compared with other existing spectrum sensing algorithm.
更多查看译文
关键词
global spectrum picture,Bayesian inference,spatio-temporal correlation,nonparametric Bayesian learning model,spatial-temporal spectrum sensing data,global spectrum states,static secondary users,heterogeneous networks,mobile Bayesian spectrum learning
AI 理解论文
溯源树
样例
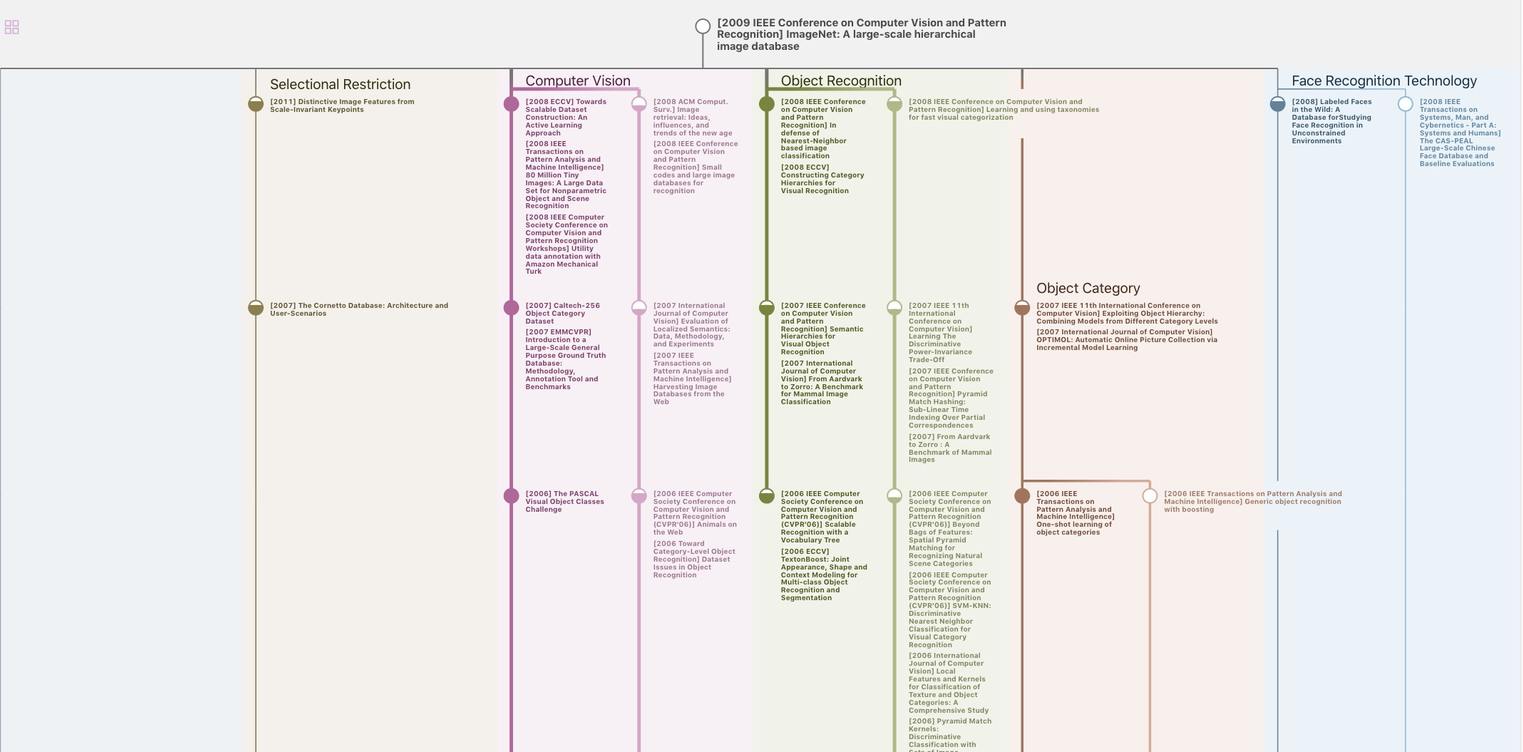
生成溯源树,研究论文发展脉络
Chat Paper
正在生成论文摘要