Voxel-Based Lesion-Symptom Mapping: A Nonparametric Bayesian Approach
IEEE International Conference on Acoustics, Speech, and Signal Processing (ICASSP)(2018)CCF B
Univ Tours | Univ Orleans | CHR Orleans
Abstract
The study of brain-injured patients (or lesion-based analysis) is a powerful paradigm for investigating structure-function relationships using neuroimaging techniques. Voxel-based Lesion-Symptom Mapping (VLSM) has been widely used to detect structure-function associations in neuroimaging studies. However this approach is based on Student t-test for which normality does not always hold. Our aim in the current study is twofold: 1) to confirm/refute the implication of the classical language areas using the Language Screening (LAST) test; and 2) to determine if it is possible to reduce the number of patients included in the VLSM study, using a different statistical approach. To achieve the second goal, we propose an alternative nonparametric and Bayesian test using Pólya trees. The approach is Bayesian, assigning prior distributions and computing the Bayes factor of H0 (null hypothesis) to H1 (alternative); and it is nonparametric since the priors are put on the unknown distribution functions under H0 and H1. Our results highlight that the Pólya tree prior provides a convenient and effective way for testing two sample differences in VLSM studies.
MoreTranslated text
Key words
Hypothesis testing,Nonparametric Bayes,Polya tree,VLSM,stroke,LAST test
求助PDF
上传PDF
View via Publisher
AI Read Science
AI Summary
AI Summary is the key point extracted automatically understanding the full text of the paper, including the background, methods, results, conclusions, icons and other key content, so that you can get the outline of the paper at a glance.
Example
Background
Key content
Introduction
Methods
Results
Related work
Fund
Key content
- Pretraining has recently greatly promoted the development of natural language processing (NLP)
- We show that M6 outperforms the baselines in multimodal downstream tasks, and the large M6 with 10 parameters can reach a better performance
- We propose a method called M6 that is able to process information of multiple modalities and perform both single-modal and cross-modal understanding and generation
- The model is scaled to large model with 10 billion parameters with sophisticated deployment, and the 10 -parameter M6-large is the largest pretrained model in Chinese
- Experimental results show that our proposed M6 outperforms the baseline in a number of downstream tasks concerning both single modality and multiple modalities We will continue the pretraining of extremely large models by increasing data to explore the limit of its performance
Upload PDF to Generate Summary
Must-Reading Tree
Example
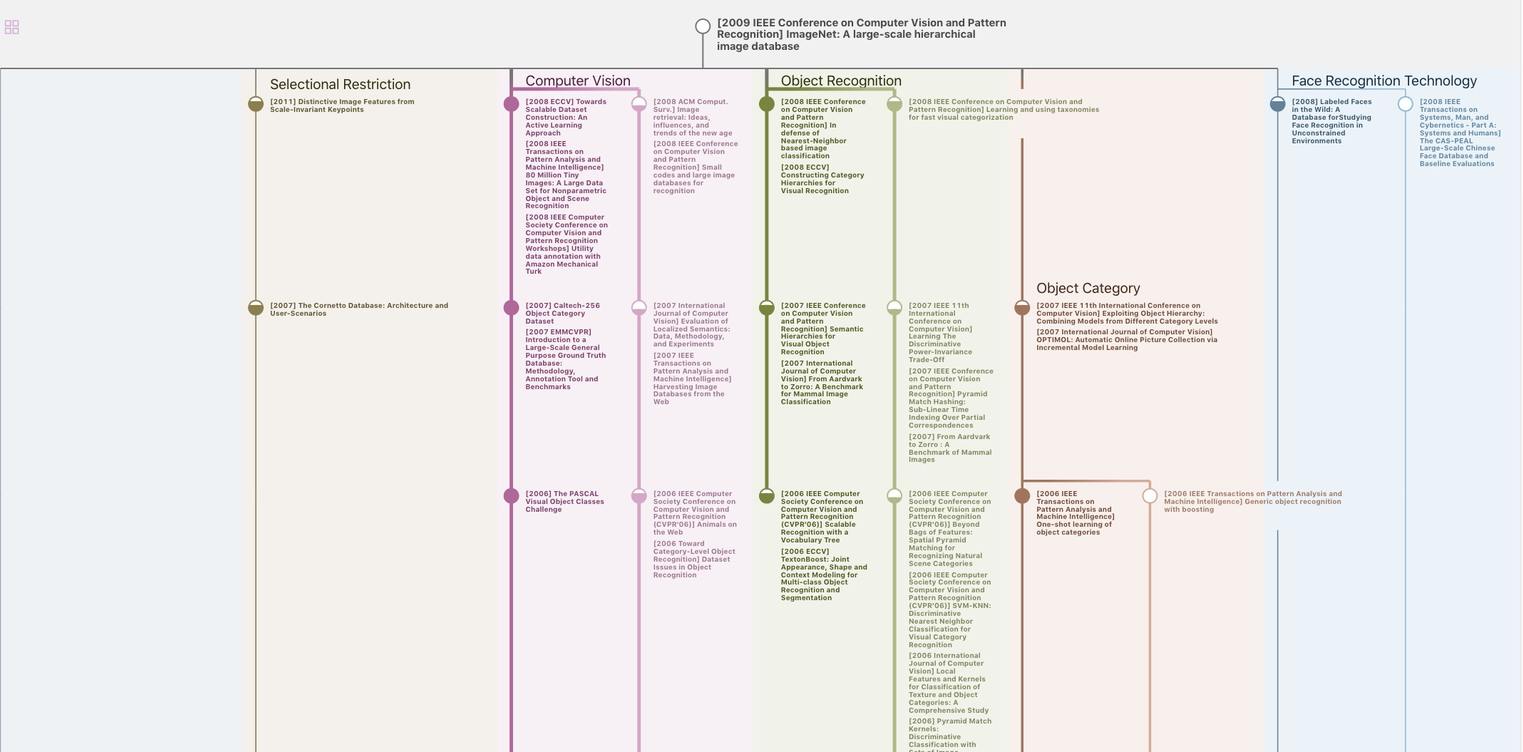
Generate MRT to find the research sequence of this paper
Related Papers
A Bayesian Estimation Formulation to Voxel-Based Lesion-Symptom Mapping.
2022 30th European Signal Processing Conference (EUSIPCO) 2022
被引用0
Data Disclaimer
The page data are from open Internet sources, cooperative publishers and automatic analysis results through AI technology. We do not make any commitments and guarantees for the validity, accuracy, correctness, reliability, completeness and timeliness of the page data. If you have any questions, please contact us by email: report@aminer.cn
Chat Paper
GPU is busy, summary generation fails
Rerequest