Calibrated recommendations.
RecSys '18: Twelfth ACM Conference on Recommender Systems Vancouver British Columbia Canada October, 2018(2018)
摘要
When a user has watched, say, 70 romance movies and 30 action movies, then it is reasonable to expect the personalized list of recommended movies to be comprised of about 70% romance and 30% action movies as well. This important property is known as calibration, and recently received renewed attention in the context of fairness in machine learning. In the recommended list of items, calibration ensures that the various (past) areas of interest of a user are reflected with their corresponding proportions. Calibration is especially important in light of the fact that recommender systems optimized toward accuracy (e.g., ranking metrics) in the usual offline-setting can easily lead to recommendations where the lesser interests of a user get crowded out by the user's main interests-which we show empirically as well as in thought-experiments. This can be prevented by calibrated recommendations. To this end, we outline metrics for quantifying the degree of calibration, as well as a simple yet effective re-ranking algorithm for post-processing the output of recommender systems.
更多查看译文
关键词
Recommender Systems, Calibration, Fairness, Diversity
AI 理解论文
溯源树
样例
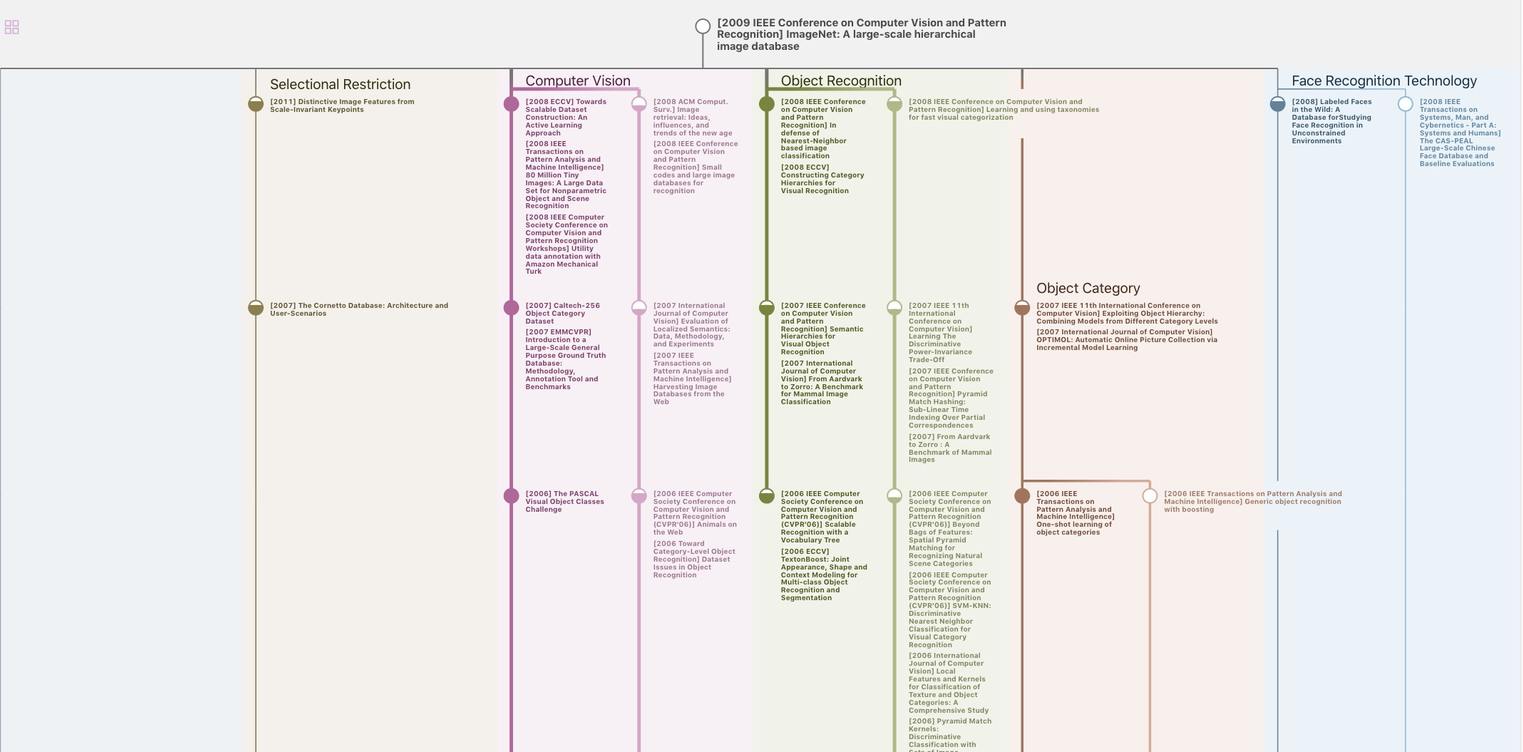
生成溯源树,研究论文发展脉络
Chat Paper
正在生成论文摘要