A Mixed (2 ,p -like)-Norm Penalized Least Mean Squares Algorithm for Block-Sparse System Identification
CSSP(2018)
摘要
This work presents a new mixed (2, p -like)-norm penalized least mean squares (LMS) algorithm for block-sparse system identifications where the nonzero coefficients in the impulse response vector of unknown systems are structured in a single cluster or multiple clusters. The new algorithm divides the tap-weight vector into groups of equal-sized sub-vectors and then introduces a mixed l_2,p-like -norm constraint on the filter tap-weight vector in addition to the original mean-square-error cost function. The parameter p in the l_2,p-like -norm constraint takes any value between zero and two, thus improving the identification performance of the block-sparse systems. The effect of the parameter p and the group size on the performance of the proposed algorithm is studied, and general guidelines for choosing these two parameters are provided to facilitate practical use. The advantage of the proposed scheme is that no comparison operations are required while algebraic operations are of the same order as the block-sparse LMS algorithm. Numerical simulations show that the proposed (2,p-like) -norm penalized LMS algorithm outperforms the existing l_2,0 - and l_2,1 -norm-based block-sparsity-aware algorithms and single-norm penalized LMS strategies.
更多查看译文
关键词
Adaptive filtering,l_2, p-like norm,LMS algorithm,Block-sparse system identifications
AI 理解论文
溯源树
样例
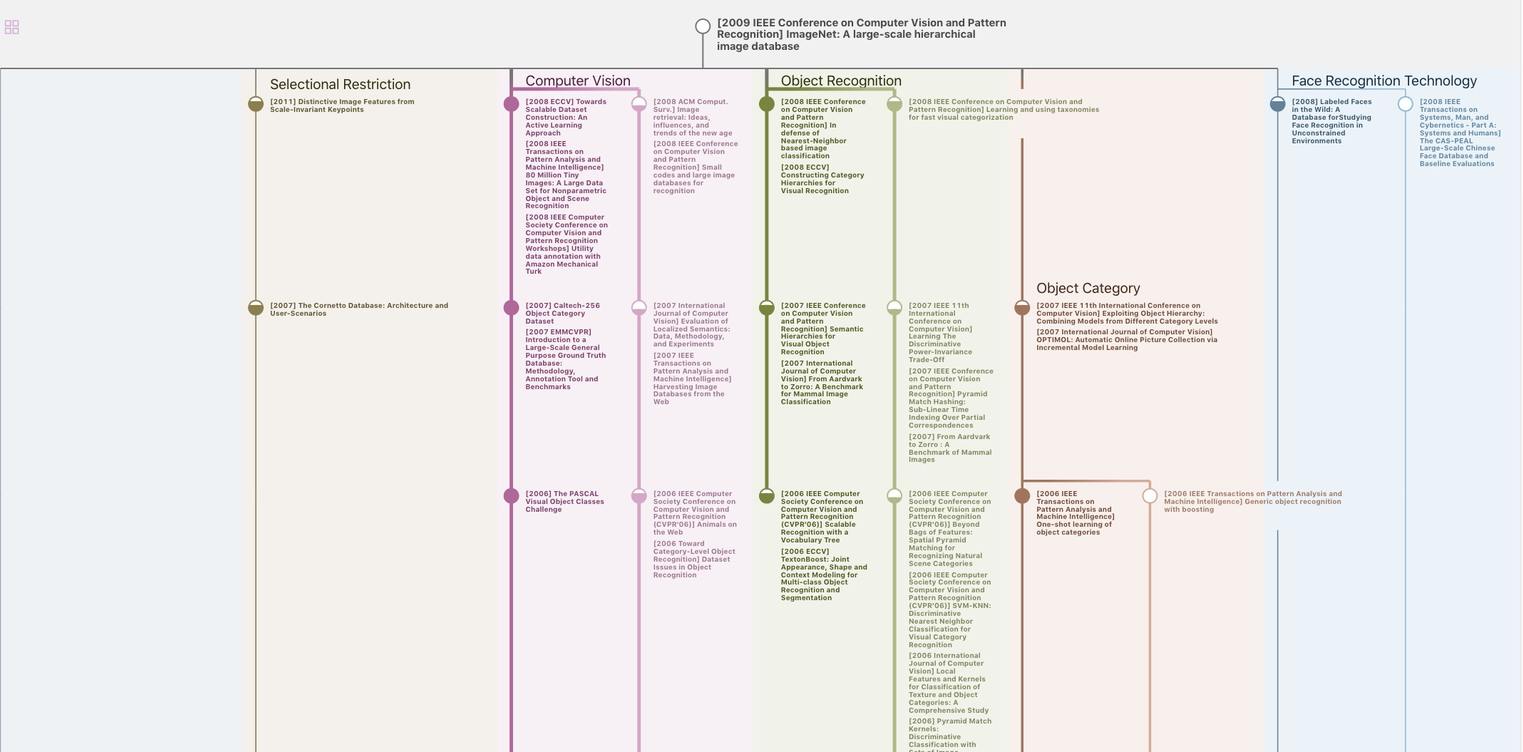
生成溯源树,研究论文发展脉络
Chat Paper
正在生成论文摘要