Bigdata logs analysis based on seq2seq networks for cognitive Internet of Things.
Future Generation Computer Systems(2019)
摘要
While bigdata system processes high-volume data at high speed, it also generates a large amount of logs. However, it is hard for people to predict future events based on massive, multi-source, heterogeneous bigdata logs. This paper proposes a comprehensive method for smart computation and prediction of massive logs in the internet of things (IoT). Traditional machine learning, Hidden Markov Model (HMM) and Autoregressive Integrated Moving Average Model (ARIMA) methods are not accurate enough to predict time series based data over time. In this work we first elaborate the distributed collection and storage, event location, and vectorized representations of bigdata logs. Next, we present a log fusion algorithm to convert the logs (unstructured text data) of each component of bigdata into structured data by removing noise, adding timestamps and classification labels. Then, we introduce a predictive model for bigdata system. We use an attention mechanism to improve sequence to sequence (seq2seq) algorithm and add an adjustor to globally fit the data distribution. Our experimental results show that the neural network model trained by our method has a good performance with the real-world data. Compared with the previous predictive method, the root mean square error (RMSE) is reduced by 46.65% and the R-squared (R2) fitting degree is improved by 14.28%.
更多查看译文
关键词
Cognitive computing,Internet of Things,Bigdata,Recurrent neural network,Log analysis
AI 理解论文
溯源树
样例
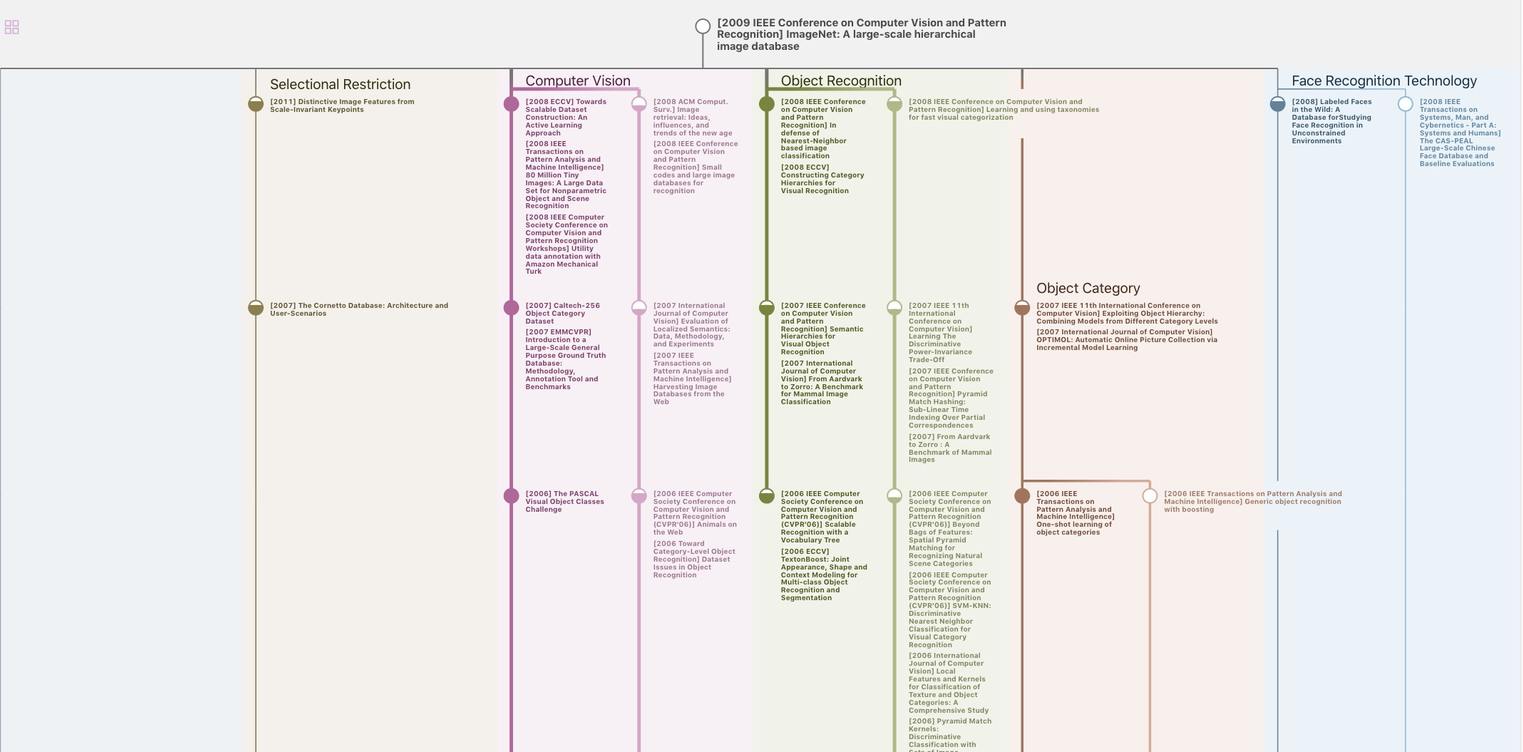
生成溯源树,研究论文发展脉络
Chat Paper
正在生成论文摘要