Application of Ensemble-Based Machine Learning Models to Landslide Susceptibility Mapping.
REMOTE SENSING(2018)
摘要
The main purpose of this study was to produce landslide susceptibility maps using various ensemble-based machine learning models (i.e., the AdaBoost, LogitBoost, Multiclass Classifier, and Bagging models) for the Sacheon-myeon area of South Korea. A landslide inventory map including a total of 762 landslides was compiled based on reports and aerial photograph interpretations. The landslides were randomly separated into two datasets: 70% of landslides were selected for the model establishment and 30% were used for validation purposes. Additionally, 20 landslide condition factors divided into five categories (topographic factors, hydrological factors, soil map, geological map, and forest map) were considered in the landslide susceptibility mapping. The relationships among landslide occurrence and landslide conditioning factors were analyzed and the landslide susceptibility maps were calculated and drawn using the AdaBoost, LogitBoost, Multiclass Classifier, and Bagging models. Finally, the maps were validated using the area under the curve (AUC) method. The Multiclass Classifier method had higher prediction accuracy (85.9%) than the Bagging (AUC = 85.4%), LogitBoost (AUC = 84.8%), and AdaBoost (84.0%) methods.
更多查看译文
关键词
landslide susceptibility,Korea,AdaBoost,Bagging,LogitBoost,Multiclass classification
AI 理解论文
溯源树
样例
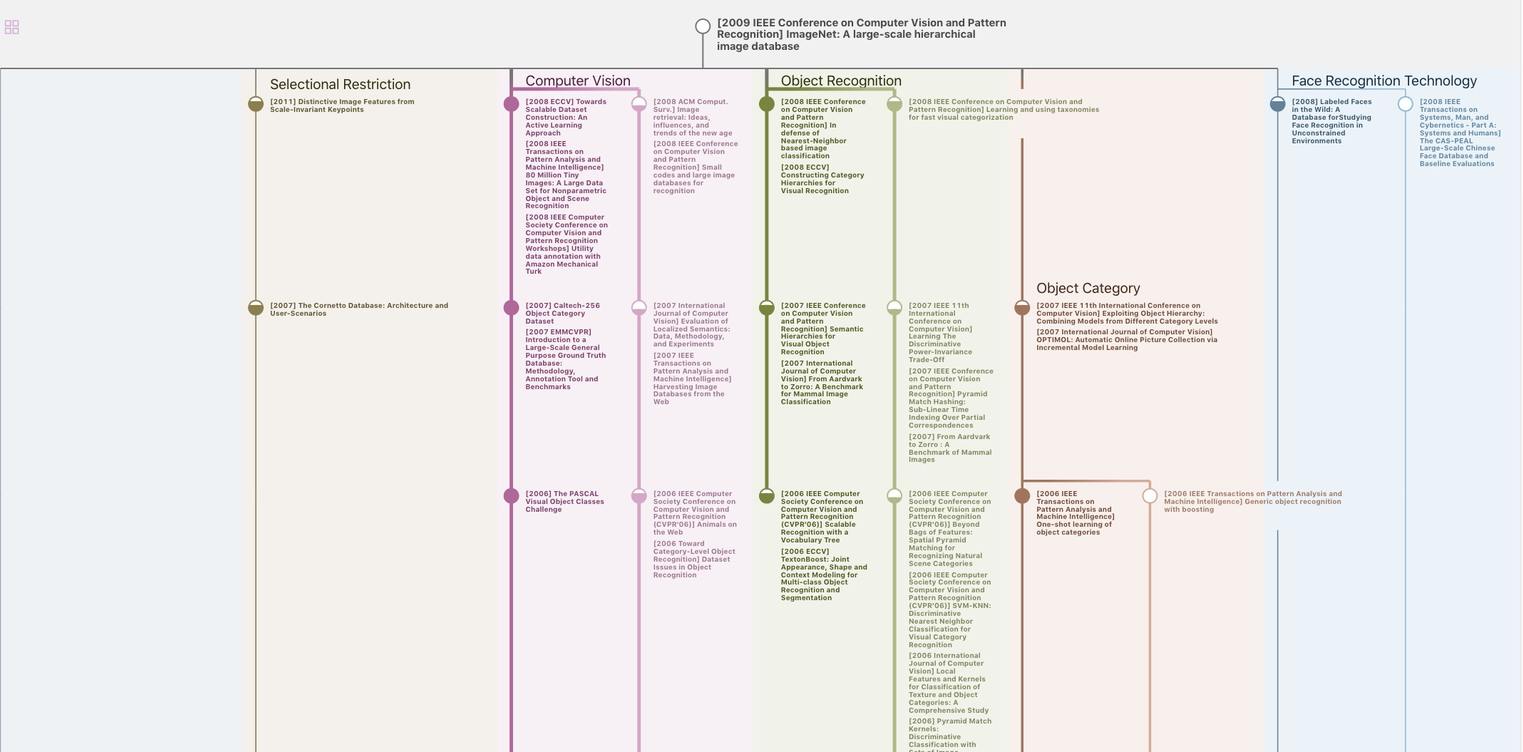
生成溯源树,研究论文发展脉络
Chat Paper
正在生成论文摘要