Nonlinear feature selection using Gaussian kernel SVM-RFE for fault diagnosis
Appl. Intell.(2018)
摘要
Feature selection can directly ascertain causes of faults by selecting useful features for fault diagnosis, which can simplify the procedures of fault diagnosis. As an efficient feature selection method, the linear kernel support vector machine recursive feature elimination (SVM-RFE) has been successfully applied to fault diagnosis. However, fault diagnosis is not a linear issue. Thus, this paper introduces the Gaussian kernel SVM-RFE to extract nonlinear features for fault diagnosis. The key issue is the selection of the kernel parameter for the Gaussian kernel SVM-RFE. We introduce three classical and simple kernel parameter selection methods and compare them in experiments. The proposed fault diagnosis framework combines the Gaussian kernel SVM-RFE and the SVM classifier, which can improve the performance of fault diagnosis. Experimental results on the Tennessee Eastman process indicate that the proposed framework for fault diagnosis is an advanced technique.
更多查看译文
关键词
Feature selection,Gaussian kernel,Fault diagnosis,Support vector machine,Tennessee Eastman process
AI 理解论文
溯源树
样例
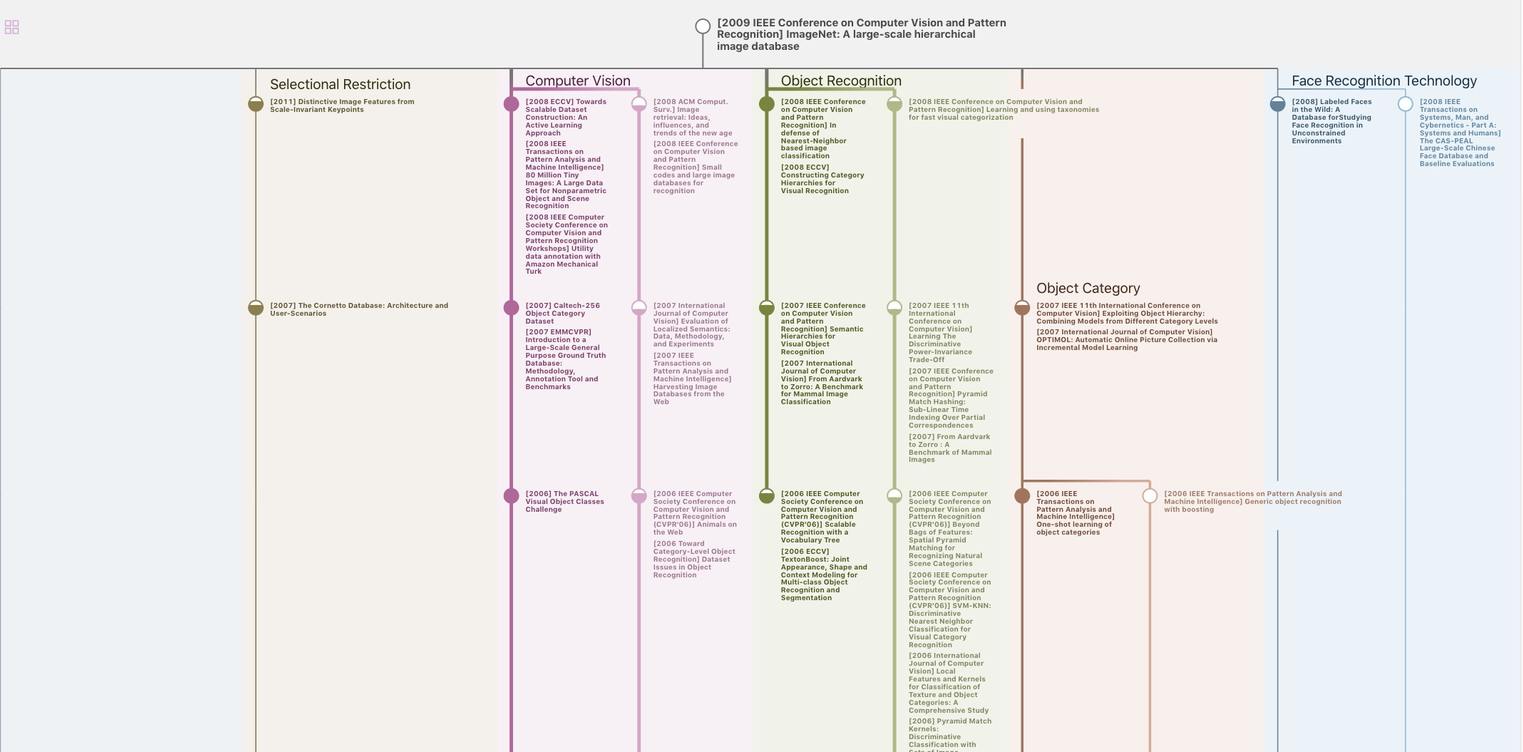
生成溯源树,研究论文发展脉络
Chat Paper
正在生成论文摘要