Learning visual models for person detection and action prediction. (Apprentissage de modèles visuels pour la détection de personnes et la prédiction d'actions).
dblp(2018)
摘要
In this thesis, we address person detection and action prediction in visual data. We develop models that learn representations for visual data and the structure in the output space while making use of contextual cues and temporal consistency. We also propose a predictive model to anticipate person’s attention in given static scenes. In the first part of the thesis, we explores the strong association between scene categories and actions. Based on that understanding, we formulate a new task of predicting human actions in static scenes. To train and evaluate the proposed model, we collect a new dataset of scene-action associations, named SUN Action dataset. The success of this task enables potential applications such as affordance geo-localization. The second part of the thesis is focused on person and generic object detection in videos. First, we construct contextual models to enhance person detection in individual frames. We train and evaluate our method on our new HollywoodHeads dataset with annotated human heads in movies. Our models consistently improve detection performance over baseline detectors. Second, we introduce a novel convolutional neural network architecture operating on short clips of frames to leverage temporal consistency and to learn spatio-temporal representations. By empirical experiments, we demonstrate the benefit of our spatio-temporal representations for object detection in videos. Last, we learn video representations that incorporate multiscale information on coarse time scales and design practical frameworks that achieve accuracy, efficiency and predictive power. Compared to per-frame features, our video …
更多查看译文
AI 理解论文
溯源树
样例
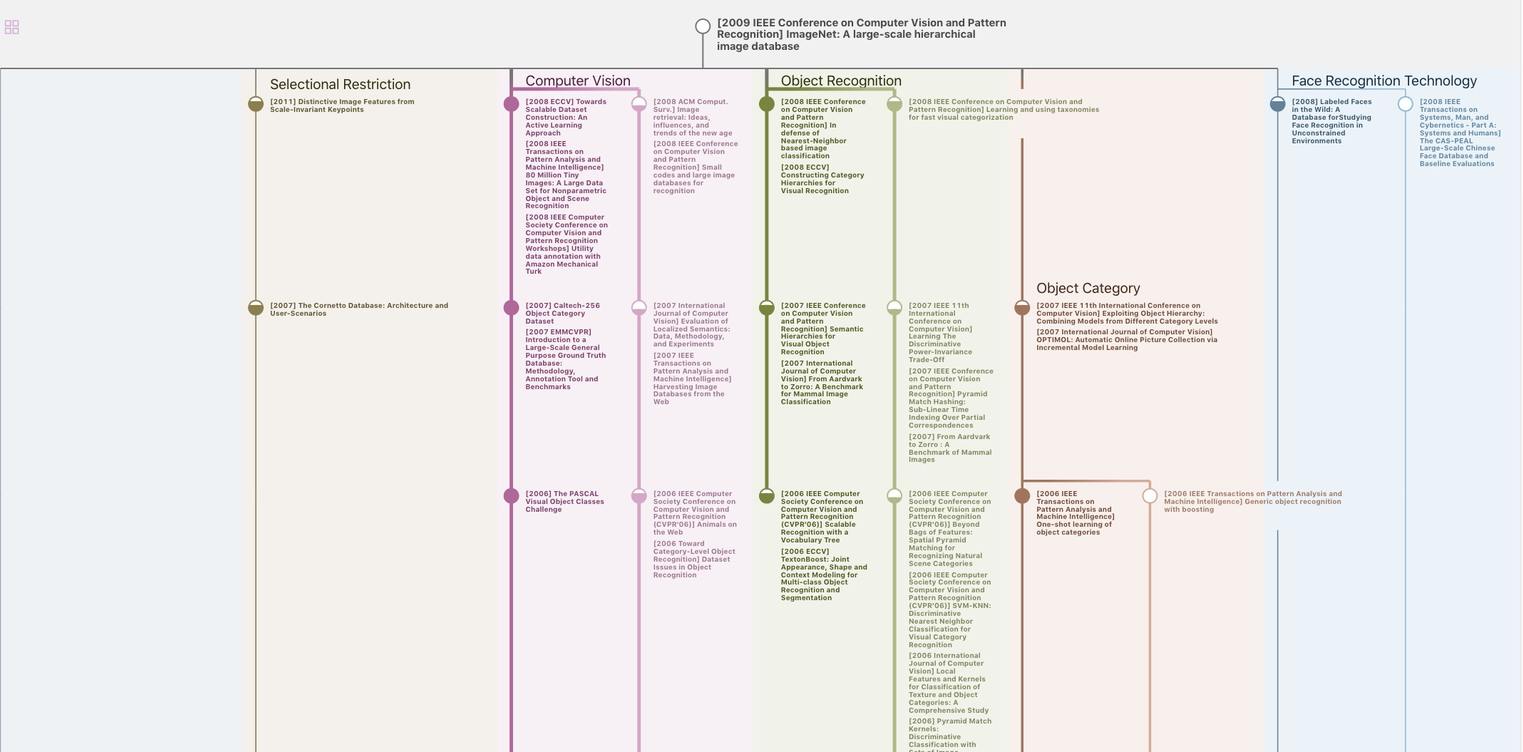
生成溯源树,研究论文发展脉络
Chat Paper
正在生成论文摘要