FITsense: Employing Multi-modal Sensors in Smart Homes to Predict Falls.
ICCBR(2018)
摘要
As people live longer, the increasing average age of the population places additional strains on our health and social services. There are widely recognised benefits to both the individual and society from supporting people to live independently for longer in their own homes. However, falls in particular have been found to be a leading cause of the elderly moving into care, and yet surprisingly preventative approaches are not in place; fall detection and rehabilitation are too late. In this paper we present FITsense, which is building a Smart Home environment to identify increased risk of falls for residents, and so allow timely interventions before falls occurs. An ambient sensor network, installed in the Smart Home, identifies low level events taking place which is analysed to generate a resident’s profile of activities of daily living (ADLs). These ADL profiles are compared to both the resident’s typical profile and to known “risky” profiles to allow evidence-driven intervention recommendations. Human activity recognition to identify ADLs from sensor data is a key challenge. Here we compare a windowing-based and a sequence-based event representation on four existing datasets. We find that windowing works well, giving consistent performance but may lack sufficient granularity for more complex multi-part activities.
更多查看译文
关键词
Human activity recognition,Smart Homes,Sensors
AI 理解论文
溯源树
样例
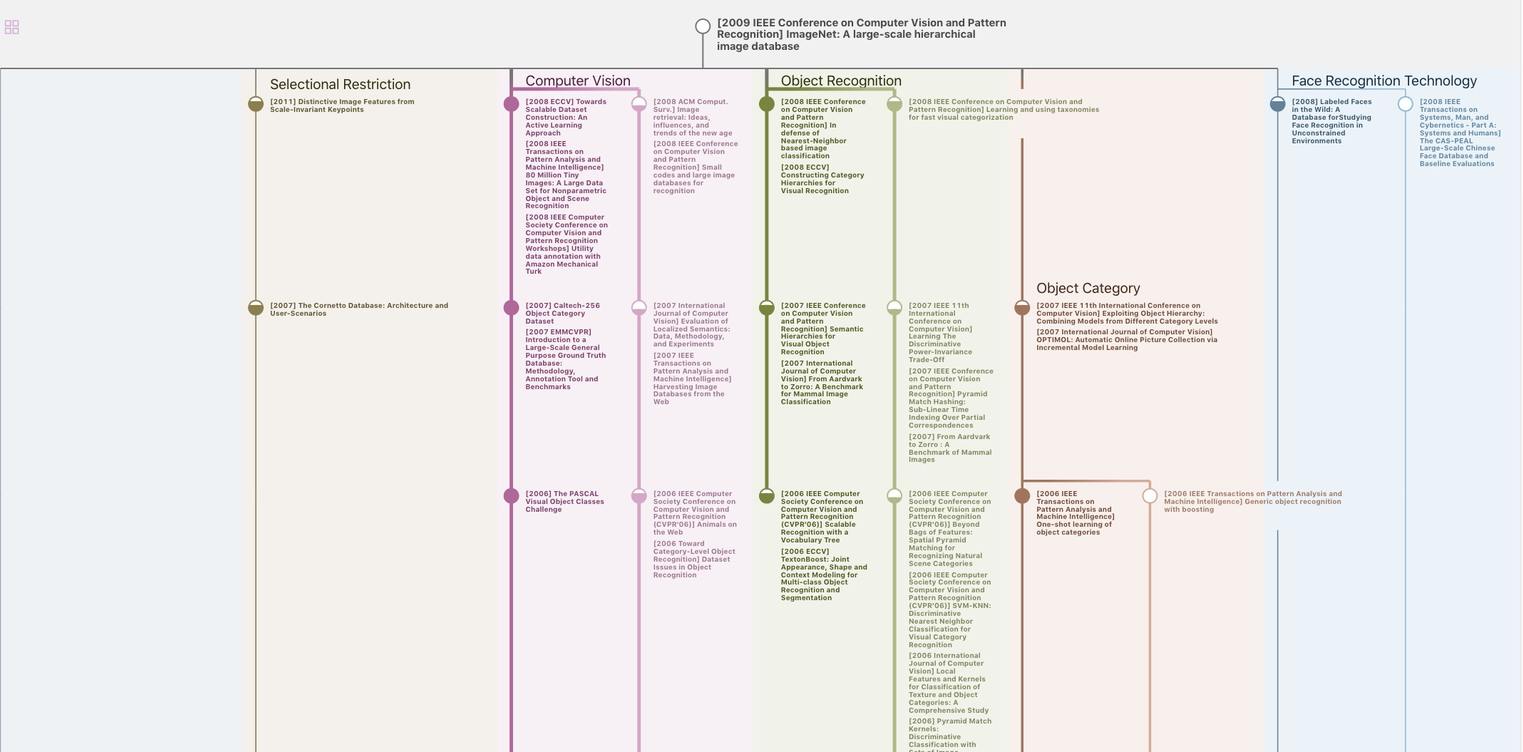
生成溯源树,研究论文发展脉络
Chat Paper
正在生成论文摘要