Learning Semantic Structure-preserved Embeddings for Cross-modal Retrieval.
MM '18: ACM Multimedia Conference Seoul Republic of Korea October, 2018(2018)
摘要
This paper learns semantic embeddings for multi-label cross-modal retrieval. Our method exploits the structure in semantics represented by label vectors to guide the learning of embeddings. First, we construct a semantic graph based on label vectors which incorporates data from both modalities, and enforce the embeddings to preserve the local structure of this semantic graph. Second, we enforce the embeddings to well reconstruct the labels, i.e., the global semantic structure. In addition, we encourage the embeddings to preserve local geometric structure of each modality. Accordingly, the local and global semantic structure consistencies as well as the local geometric structure consistency are enforced, simultaneously. The mappings between inputs and embeddings are designed to be nonlinear neural network with larger capacity and more flexibility. The overall objective function is optimized by stochastic gradient descent to gain the scalability on large datasets. Experiments conducted on three real world datasets clearly demonstrate the superiority of our proposed approach over the state-of-the-art methods.
更多查看译文
关键词
cross-modal retrieval, graph embeddings, semantic embeddings
AI 理解论文
溯源树
样例
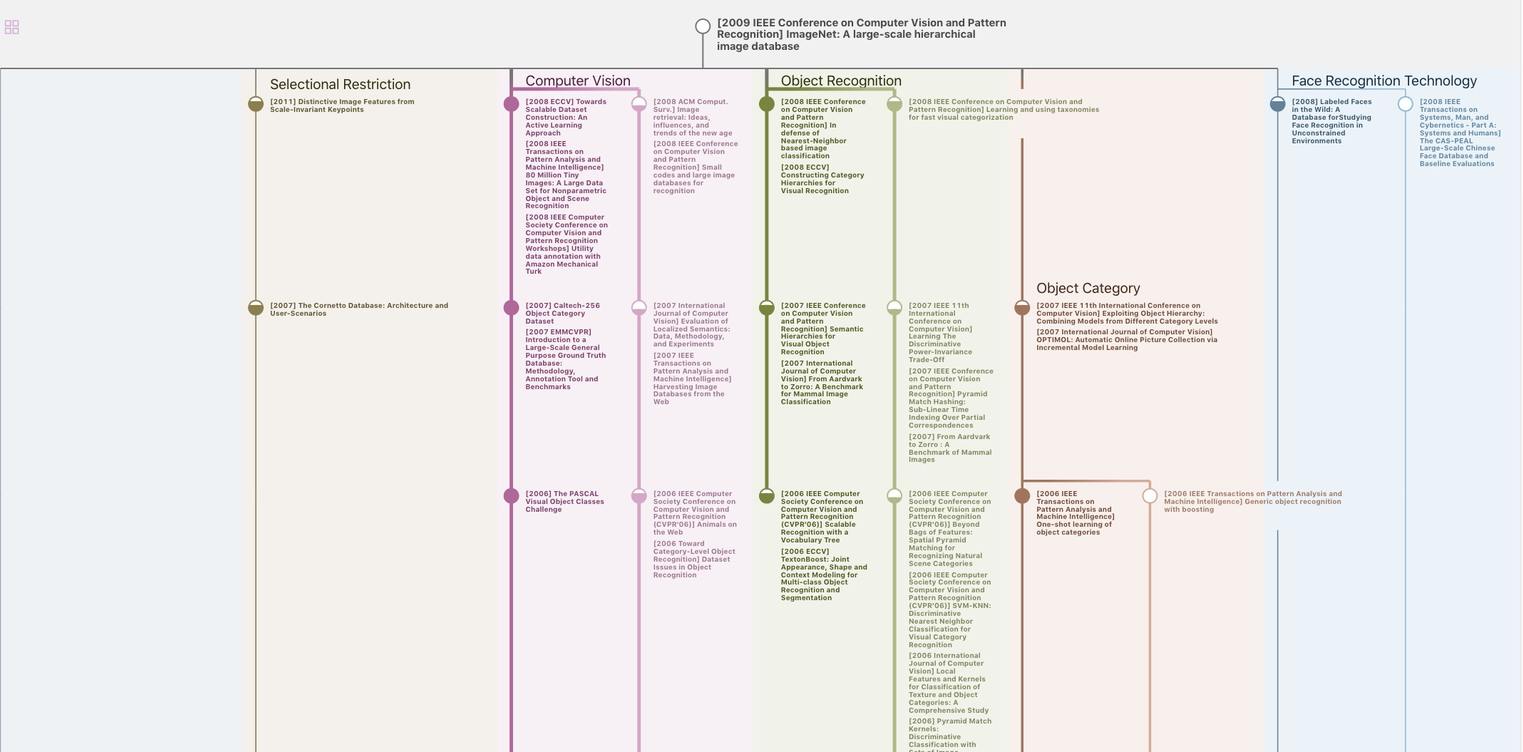
生成溯源树,研究论文发展脉络
Chat Paper
正在生成论文摘要