Deep Learning Interpretation.
MM '18: ACM Multimedia Conference Seoul Republic of Korea October, 2018(2018)
摘要
Deep learning has been successfully exploited in addressing different multimedia problems in recent years. The academic researchers are now transferring their attention from identifying what problem deep learning CAN address to exploring what problem deep learning CAN NOT address. This tutorial starts with a summarization of six 'CAN NOT' problems deep learning fails to solve at the current stage, i.e., low stability, debugging difficulty, poor parameter transparency, poor incrementality, poor reasoning ability, and machine bias. These problems share a common origin from the lack of deep learning interpretation. This tutorial attempts to correspond the six 'NOT' problems to three levels of deep learning interpretation: (1) Locating - accurately and efficiently locating which feature contributes much to the output. (2) Understanding - bidirectional semantic accessing between human knowledge and deep learning algorithm. (3) Expandability - well storing, accumulating and reusing the models learned from deep learning. Existing studies falling into these three levels will be reviewed in detail, and a discussion on the future interesting directions will be provided in the end.
更多查看译文
关键词
deep learning, interpretable machine learning
AI 理解论文
溯源树
样例
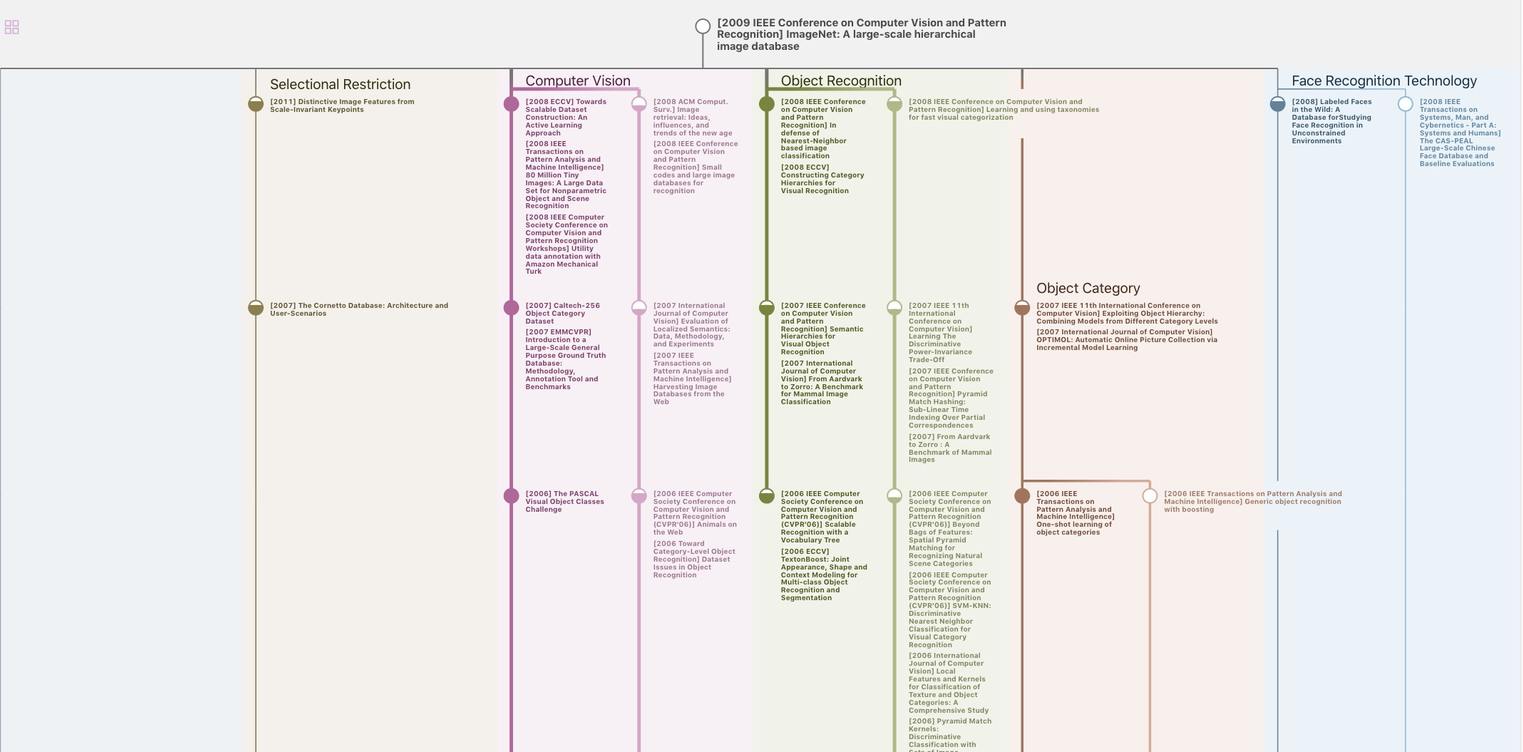
生成溯源树,研究论文发展脉络
Chat Paper
正在生成论文摘要