CSAN: Contextual Self-Attention Network for User Sequential Recommendation.
MM '18: ACM Multimedia Conference Seoul Republic of Korea October, 2018(2018)
摘要
The sequential recommendation is an important task for online user-oriented services, such as purchasing products, watching videos, and social media consumption. Recent work usually used RNN-based methods to derive an overall embedding of the whole behavior sequence, which fails to discriminate the significance of individual user behaviors and thus decreases the recommendation performance. Besides, RNN-based encoding has fixed size and makes further recommendation application inefficient and inflexible. The online sequential behaviors of a user are generally heterogeneous, polysemous, and dynamically context-dependent. In this paper, we propose a unified Contextual Self-Attention Network (CSAN) to address the three properties. Heterogeneous user behaviors are considered in our model that are projected into a common latent semantic space. Then the output is fed into the feature-wise self-attention network to capture the polysemy of user behaviors. In addition, the forward and backward position encoding matrices are proposed to model dynamic contextual dependency. Through extensive experiments on two real-world datasets, we demonstrate the superior performance of the proposed model compared with other state-of-the-art algorithms.
更多查看译文
关键词
self-attention, contextual, sequential recommendation, multi-modal
AI 理解论文
溯源树
样例
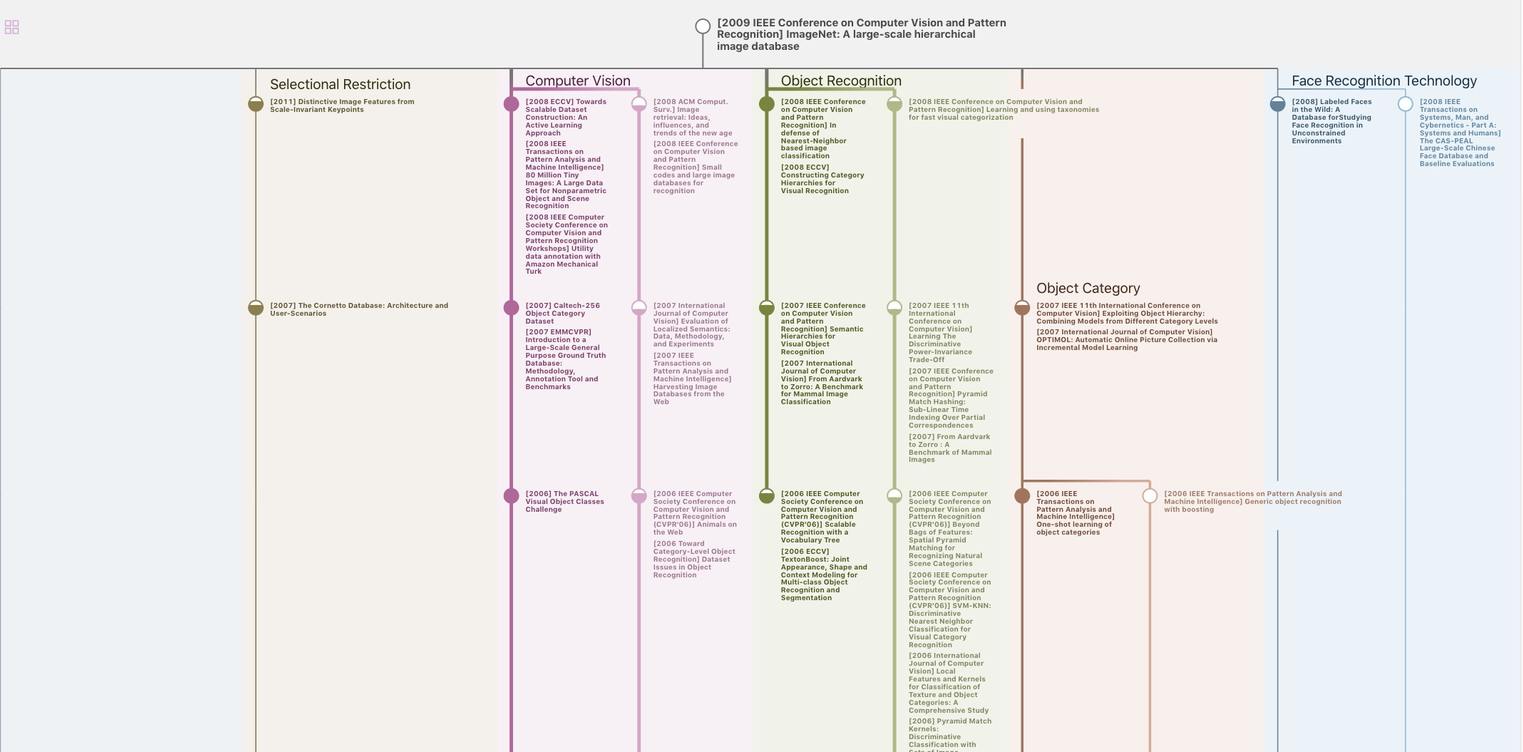
生成溯源树,研究论文发展脉络
Chat Paper
正在生成论文摘要