Cascaded Feature Augmentation with Diffusion for Image Retrieval.
MM '18: ACM Multimedia Conference Seoul Republic of Korea October, 2018(2018)
摘要
Recently, as an effective re-ranking technique, diffusion has attracted considerable attention in research on image retrieval. It inherits from random surfer model and is effective to deeply explore data manifold structure. However, as a common practice, diffusion is performed at query time which relies heavily on initial retrieval shortlists and suffers the bottleneck of online time-efficiency. To this end, in this paper, we present a more generalized method named CFA (cascaded feature augmentation) based on diffusion. First of all, we transfer diffusion process from online stage to offline stage and innovatively utilize output of diffusion to augment database features in a cascaded mode, which can eliminate iteration process at query time radically. Second, to scale the diffusion method to large image database, we propose a cascaded cluster diffusion technique for feature augmentation which largely reduces computational cost. Third, we extend our cascaded feature augmentation scheme to cases with multiple features without involving extra memory and time cost. Our CFA is compatible with other re-ranking methods. Extensive experiments on four public datasets demonstrate the effectiveness of our proposed algorithm.
更多查看译文
关键词
Offline cascaded feature augmentation, diffusion, image retrieval
AI 理解论文
溯源树
样例
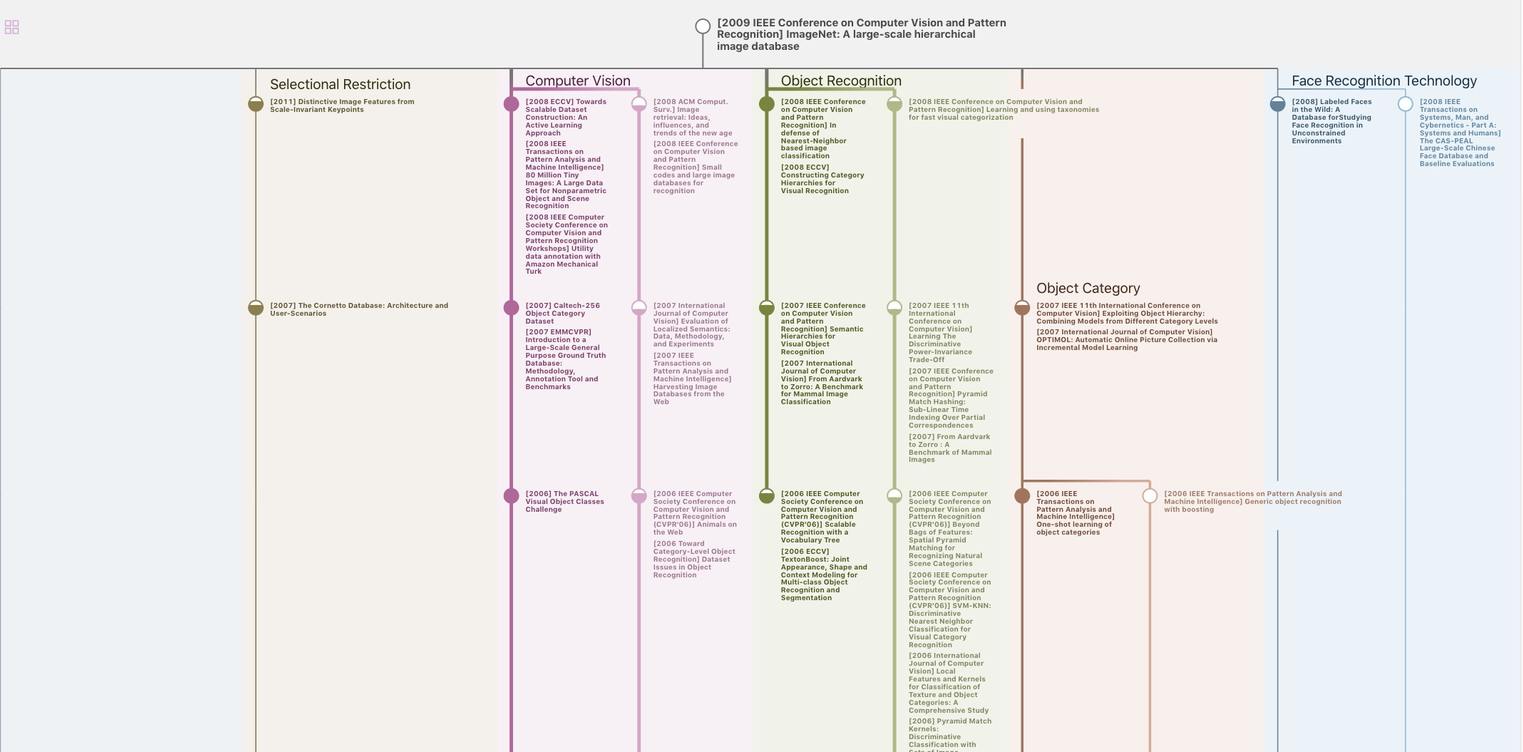
生成溯源树,研究论文发展脉络
Chat Paper
正在生成论文摘要