Feature Reinforcement Network for Image Classification
2018 IEEE International Conference on Multimedia and Expo (ICME)(2018)
摘要
Deep Learning has attracted much attention these years as it produces fabulous performance in various applications. Most researchers have mainly focused on improving and optimizing the network structure, e.g., deeper and deeper networks are constructed to extract high-level features from raw data. In this paper, we propose a two-wing deep convolutional network, called Feature Reinforcement Networks (FRN). One wing acts as the traditional operation in VGG, ResNet and DenseNet, while the other wing called feature reinforcement block (FRB) also conducts layer-wise convolution operations which share the convolution parameters of the former layer. Then, Relu function is employed in FRB to rectify the feature maps except the output layer. The outputs of these wings are integrated as the input of the next convolution layer. It is confirmed that the proposed FRN is more sensitive to the informative features. Our experiments on a few multimedia datasets prove FRN outperforms the original deep neural networks.
更多查看译文
关键词
Deep learning,Two-wing deep convolutional network,Feature reinforcement block,Image classification
AI 理解论文
溯源树
样例
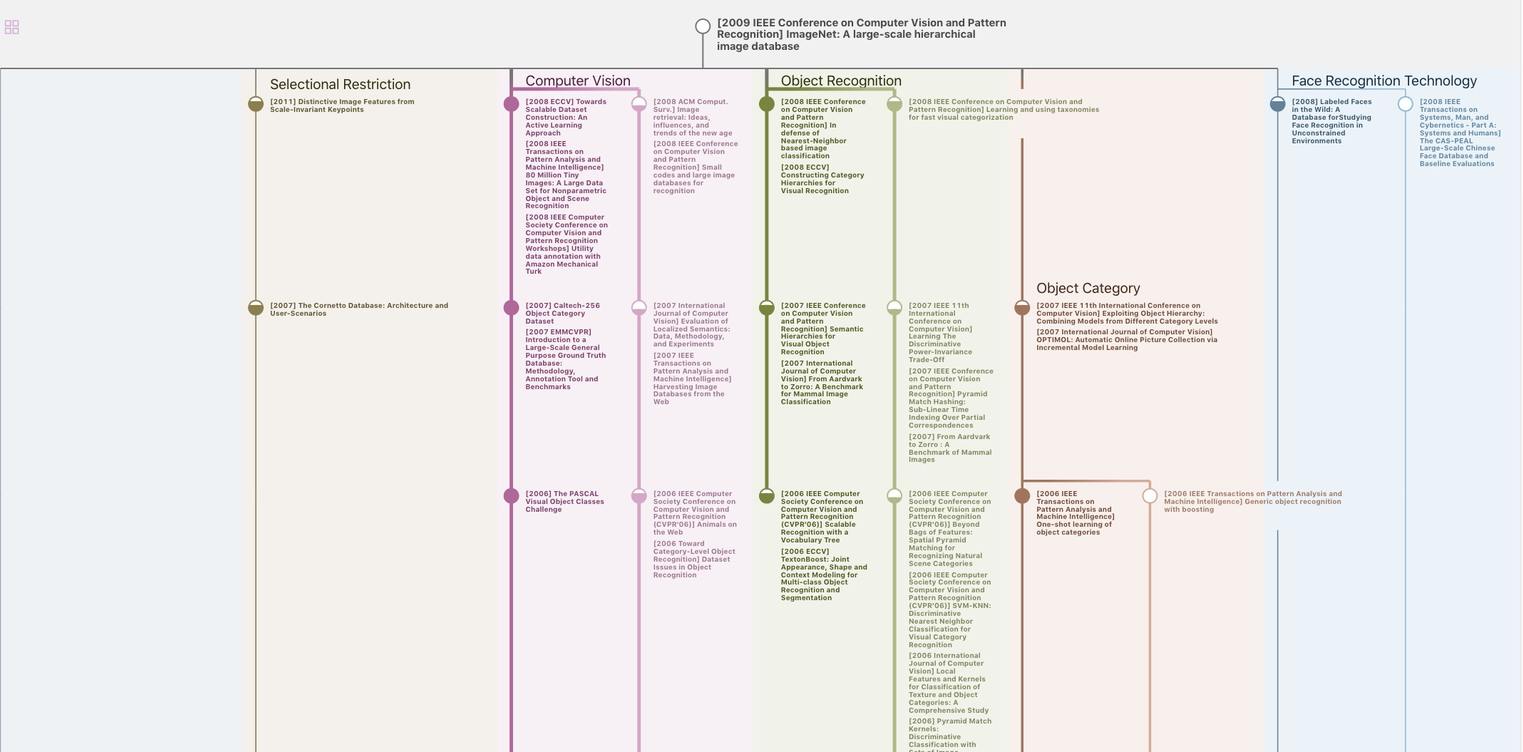
生成溯源树,研究论文发展脉络
Chat Paper
正在生成论文摘要