Feasibility of human activity recognition using wearable depth cameras.
UbiComp '18: The 2018 ACM International Joint Conference on Pervasive and Ubiquitous Computing Singapore Singapore October, 2018(2018)
摘要
Human Activity Recognition (HAR) with body-worn sensors has been studied intensively in the past decade. Existing approaches typically rely on data from inertial sensors. This paper explores the potential of using point cloud data gathered from wearable depth cameras for on-body activity recognition. We discuss effects of different granularity in the depth information and compare their performance to inertial sensor based HAR. We evaluated our approach with a total of sixteen participants performing nine distinct activity classes in three home environments. 10-fold cross-validation results of KNN and Random Forests classification exhibit a significant increase in F-score from inertial data to depth information (by > 12 percentage points) and show a further improvement when combining low-resolution depth matrices and sensor data. We discuss the performance of the different sensor types for different contexts and show that overall, depth sensors prove to be suitable for HAR.
更多查看译文
关键词
Human Activity Recognition, Mobile Computing, Google Project Tango, Wearables, Depth Sensor
AI 理解论文
溯源树
样例
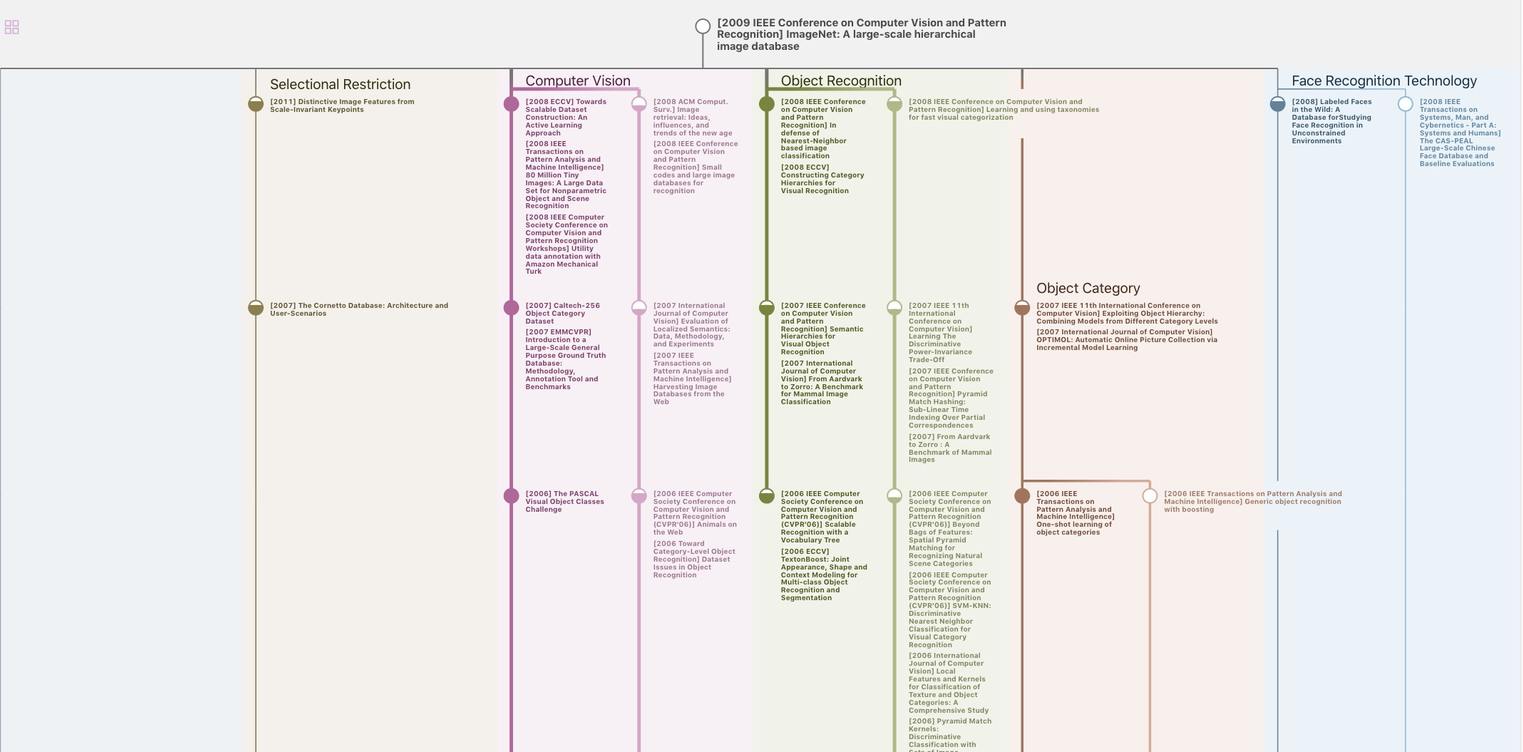
生成溯源树,研究论文发展脉络
Chat Paper
正在生成论文摘要