The Application of Gaussian Mixture Models for the Identification of At-Risk Learners in Massive Open Online Courses
2018 IEEE CONGRESS ON EVOLUTIONARY COMPUTATION (CEC)(2018)
摘要
With high learner withdrawal rates in the setting of MOOC platforms, the early identification of at-risk student groups has become increasingly important. Although many prior studies consider the dropout issue in form of a sequence classification problem, such works address only a limited set of behavioural dynamics, typically recorded as sequence of weekly interval, neglecting important contextual factors such as assignment deadlines that may be important components of student latent engagement. In this paper we, therefore, aim to investigate the use of Gaussian Mixture Models for the incorporation such important dynamics, providing an analytical assessment of the influence of latent engagement on students and their subsequent risk of leaving the course. Additionally, linear regression and, k-nearest neighbors classifiers were used to provide a performance comparison. The features used in the study were constructed from student behavioural records, capturing activity over time, which were subsequently organized into six-time intervals, corresponding to assignment submission dates. Results obtained from the classification procedure yielded an F1-Measure of 0.835 for the Gaussian Mixture Model, indicating that such an approach holds promise for the identification of at-risk students within the MODe setting.
更多查看译文
关键词
At-risk Learners,MOOC platforms,sequence classification problem,behavioural dynamics,student latent engagement,student behavioural records,at-risk students,MODe setting,gaussian mixture model,massive open online courses,student groups,contextual factors,learner withdrawal rates,linear regression,k-nearest neighbors classifiers
AI 理解论文
溯源树
样例
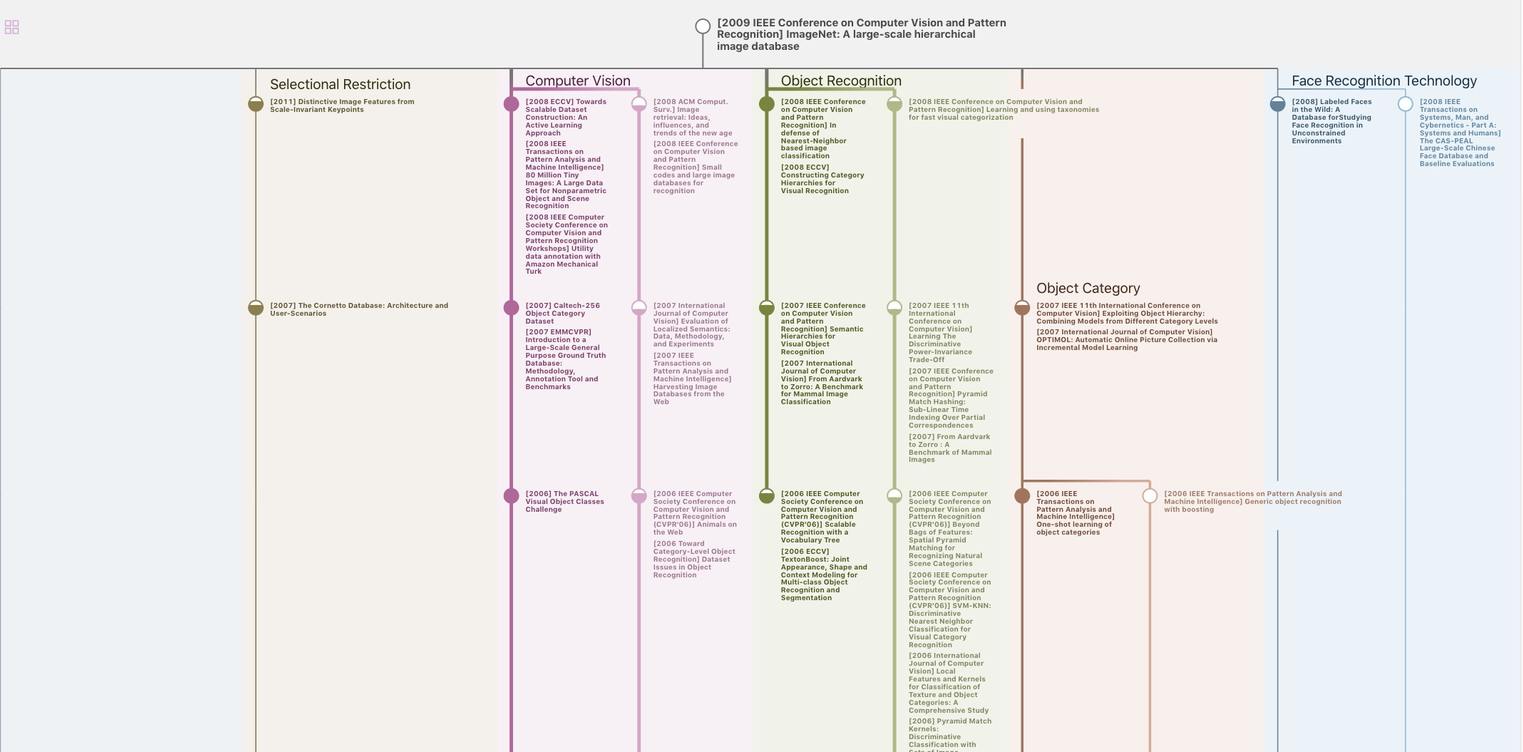
生成溯源树,研究论文发展脉络
Chat Paper
正在生成论文摘要