Avoiding Degradation In Deep Feed-Forward Networks By Phasing Out Skip-Connections
ARTIFICIAL NEURAL NETWORKS AND MACHINE LEARNING - ICANN 2018, PT III(2018)
摘要
A widely observed phenomenon in deep learning is the degradation problem: increasing the depth of a network leads to a decrease in performance on both test and training data. Novel architectures such as ResNets and Highway networks have addressed this issue by introducing various flavors of skip-connections or gating mechanisms. However, the degradation problem persists in the context of plain feed-forward networks. In this work we propose a simple method to address this issue. The proposed method poses the learning of weights in deep networks as a constrained optimization problem where the presence of skip-connections is penalized by Lagrange multipliers. This allows for skip-connections to be introduced during the early stages of training and subsequently phased out in a principled manner. We demonstrate the benefits of such an approach with experiments on MNIST, fashion-MNIST, CIFAR-10 and CIFAR-100 where the proposed method is shown to greatly decrease the degradation effect and is often competitive with ResNets.
更多查看译文
关键词
Degradation, Shattered/vanishing gradients, Skip-connections
AI 理解论文
溯源树
样例
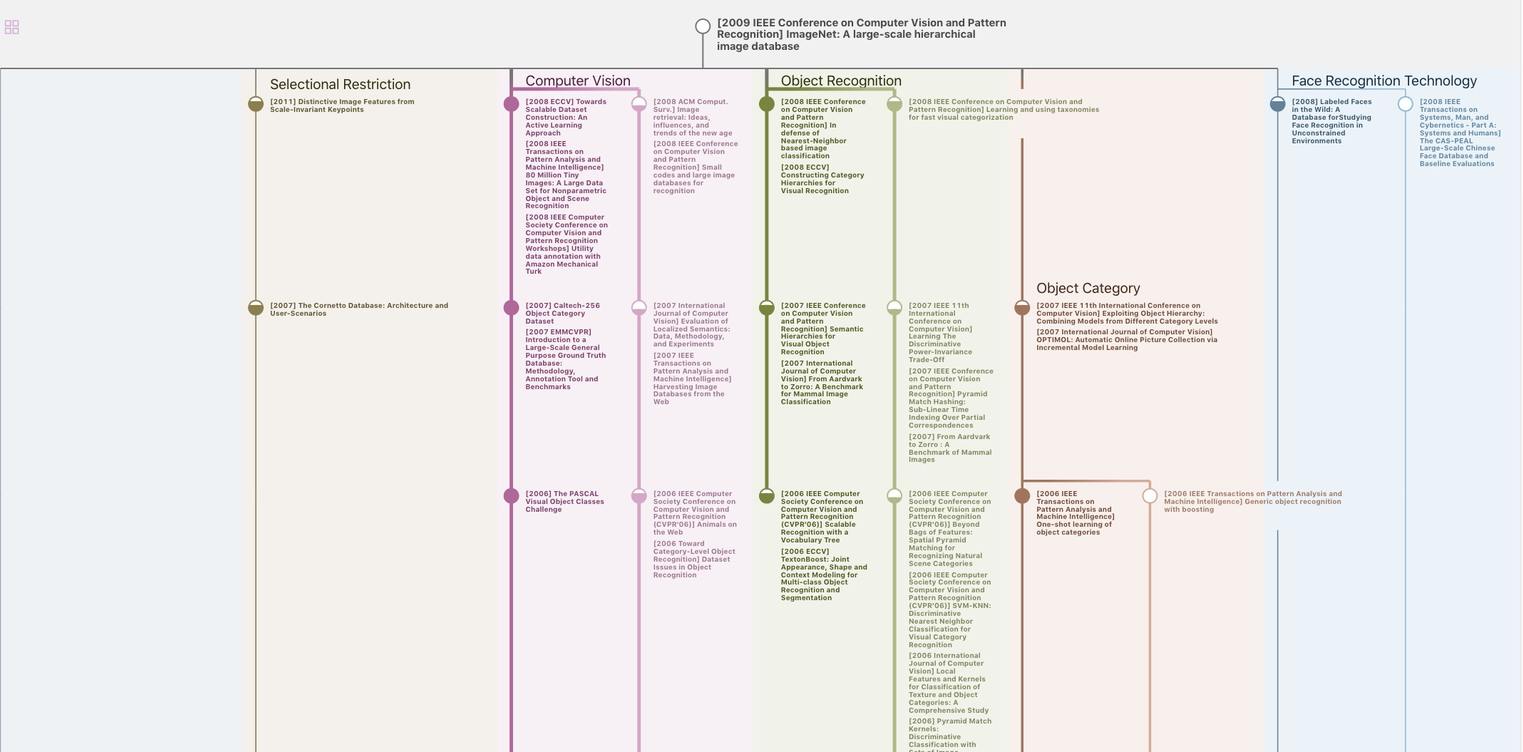
生成溯源树,研究论文发展脉络
Chat Paper
正在生成论文摘要